Feedforward Beta Control In The Kstar Tokamak By Deep Reinforcement Learning
NUCLEAR FUSION(2021)
摘要
In this work, we address a new feedforward control scheme for the normalized beta (beta (N)) in tokamak plasmas, using the deep reinforcement learning (RL) technique. The deep RL algorithm optimizes an artificial decision-making agent that adjusts the discharge scenario to obtain a given target beta (N) from the state-action-reward sets explored by its own trial and error in a virtual tokamak environment. The virtual environment for the RL training is constructed using a long short-term memory (LSTM) network that imitates the plasma responses to external actuator controls, which is trained using five years' worth of KSTAR experimental data. The RL agent then experiences numerous discharges with different actuator controls in the LSTM simulator, and its internal parameters are optimized in the direction of maximizing the reward. We analyze a series of KSTAR experiments conducted with the RL-determined scenarios to validate the feasibility of the beta control scheme in a real device. We discuss the successes and limitations of feedforward beta control by RL, and suggest a future research path for this area of study.
更多查看译文
关键词
machine learning, reinforcement learning, beta control, data-driven simulation, KSTAR, tokamak
AI 理解论文
溯源树
样例
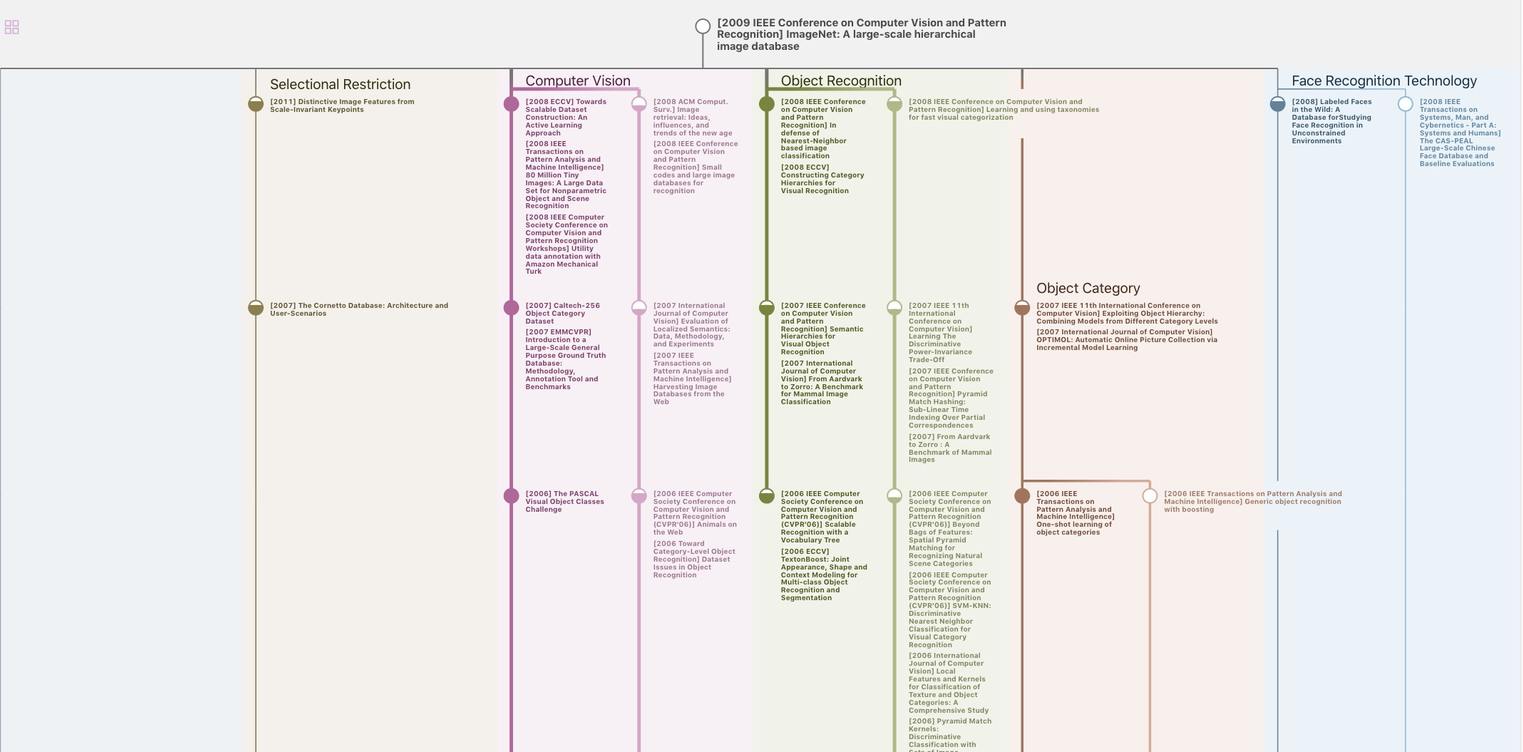
生成溯源树,研究论文发展脉络
Chat Paper
正在生成论文摘要