Deep-Freeze Graph Training For Latent Learning
COMPUTATIONAL MATERIALS SCIENCE(2021)
摘要
Scientific and engineering advances are primarily driven by multi-tier conceptual constructs and conditional theoretical frameworks. The theories allow predictions of hypothetical system responses, given a set of approximate conditions (ranges of applicability) imposed on latent parameters that cannot be measured directly. Learning to estimate the latent variables (Latent Learning) helps to pinpoint the anticipated range-edge anomalies and improves the confidence in interpretation, interpolation and extrapolation of limited experimental data. Due to high dimensionality and extreme non-linearity of the materials science problems, very large datasets are typically required for conventional data-driven model development. The vital experimental data collection, particularly on microstructural phases, is very challenging, which makes it difficult to compile a high-quality database. Incorporation of the domain knowledge into the computational graph structure, initialization and optimization processes presents a viable mechanism for developing accurate models, with limited datasets. This study successfully utilized the approach to build the Deep Freeze Graph (DeepFreG) by mapping known causality relationships and by digitizing empirical domain knowledge for Latent Learning (LL), with specific applications in materials science.
更多查看译文
关键词
Artificial intelligence, Latent learning, Causality, Pattern discovery, Outlier
AI 理解论文
溯源树
样例
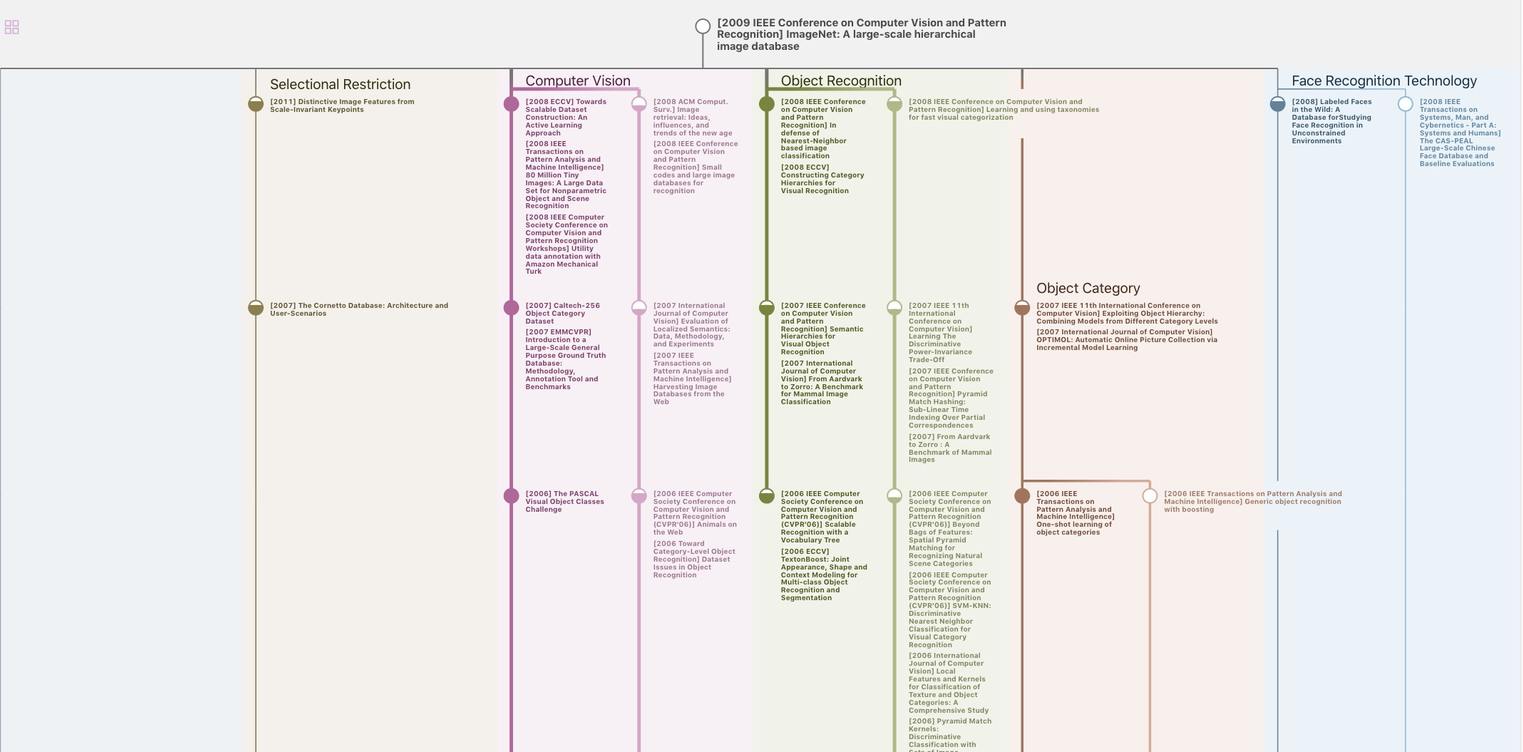
生成溯源树,研究论文发展脉络
Chat Paper
正在生成论文摘要