Prediction of Posttraumatic Epilepsy using Machine Learning
MEDICAL IMAGING 2021: BIOMEDICAL APPLICATIONS IN MOLECULAR, STRUCTURAL, AND FUNCTIONAL IMAGING(2021)
摘要
Post-traumatic Epilepsy is one of the common aftereffects of brain injury. This neurological disorder can persist throughout the lifetime of patients and impacts their quality of life significantly. Identification of markers that indicate the likelihood of developing PTE can help develop preventive care for subjects identified as at risk. Despite the relatively high prevalence of PTE, brain imaging-based biomarkers for the diagnosis of PTE are lacking. This is due in part to the heterogeneity of injury in traumatic brain injury patients. Here we investigate the use of structural and functional imaging features for training machine learning models. We compare the performance of four popular machine learning methods in predicting development of PTE after brain injury: (i) support vector machines, (ii) random forests, (iii) fully connected neural networks, and (iv) graph convolutional networks. Our results demonstrate the advantage of using a combination of connectivity features (functional) and lesion volume (structural) in conjunction with a Kernel SVM approach in predicting PTE. We also demonstrate that using a feature reduction method such as principal component analysis (PCA) can be more effective than penalizing classifiers. This might be due to the limitation of penalized models for a framework where features are correlated.
更多查看译文
关键词
fMRI,lesion detection,PTE,machine learning
AI 理解论文
溯源树
样例
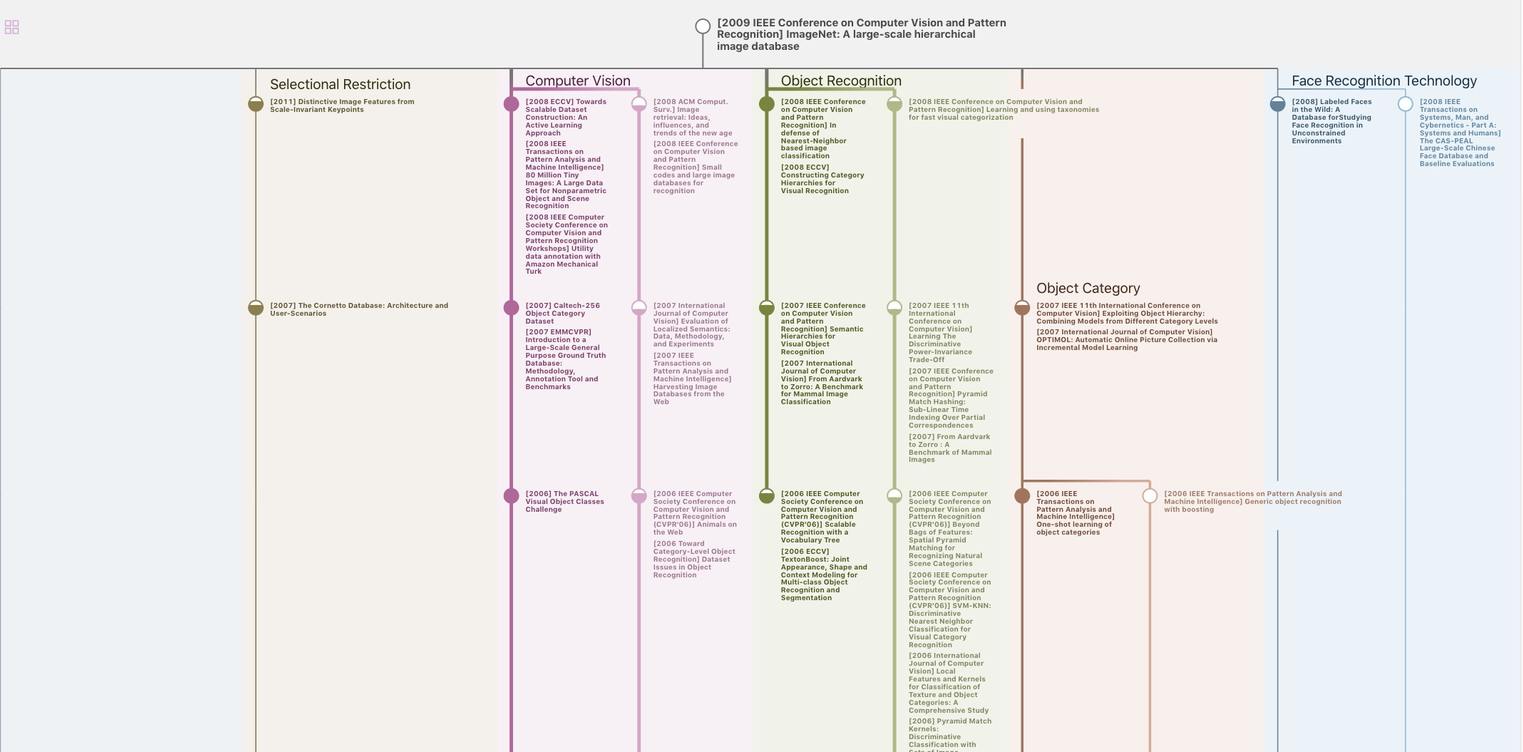
生成溯源树,研究论文发展脉络
Chat Paper
正在生成论文摘要