Incremental Parameter Estimation Of Stochastic State-Based Models
2021 IEEE 19TH WORLD SYMPOSIUM ON APPLIED MACHINE INTELLIGENCE AND INFORMATICS (SAMI 2021)(2021)
摘要
This paper presents an incremental learning approach for estimating the structural parameters in stochastic state-based models (SSMs). SSMs have proven to be useful for modelling biological and medical processes, as they can represent both time dependency and stochastic processes. A major challenge in modelling in bioinformatics is that learning processes usually rely on large publicly accessible databases. In this work, a new approach is presented, where models are trained incrementally locally at different data sources, e.g., hospitals, without having to pass on sensitive data. After learning, only the parameters of the model are passed on, in this case the arc weights of stochastic Petri nets. As a result, data protection and privacy of patients in hospitals are respected and it is no longer necessary to rely on the existence of a suitable accessible database. Simulations are used to evaluate the performance of the algorithm for a gene regulatory network.
更多查看译文
关键词
Bioinformatics, incremental learning, federated learning, stochastic state-based models
AI 理解论文
溯源树
样例
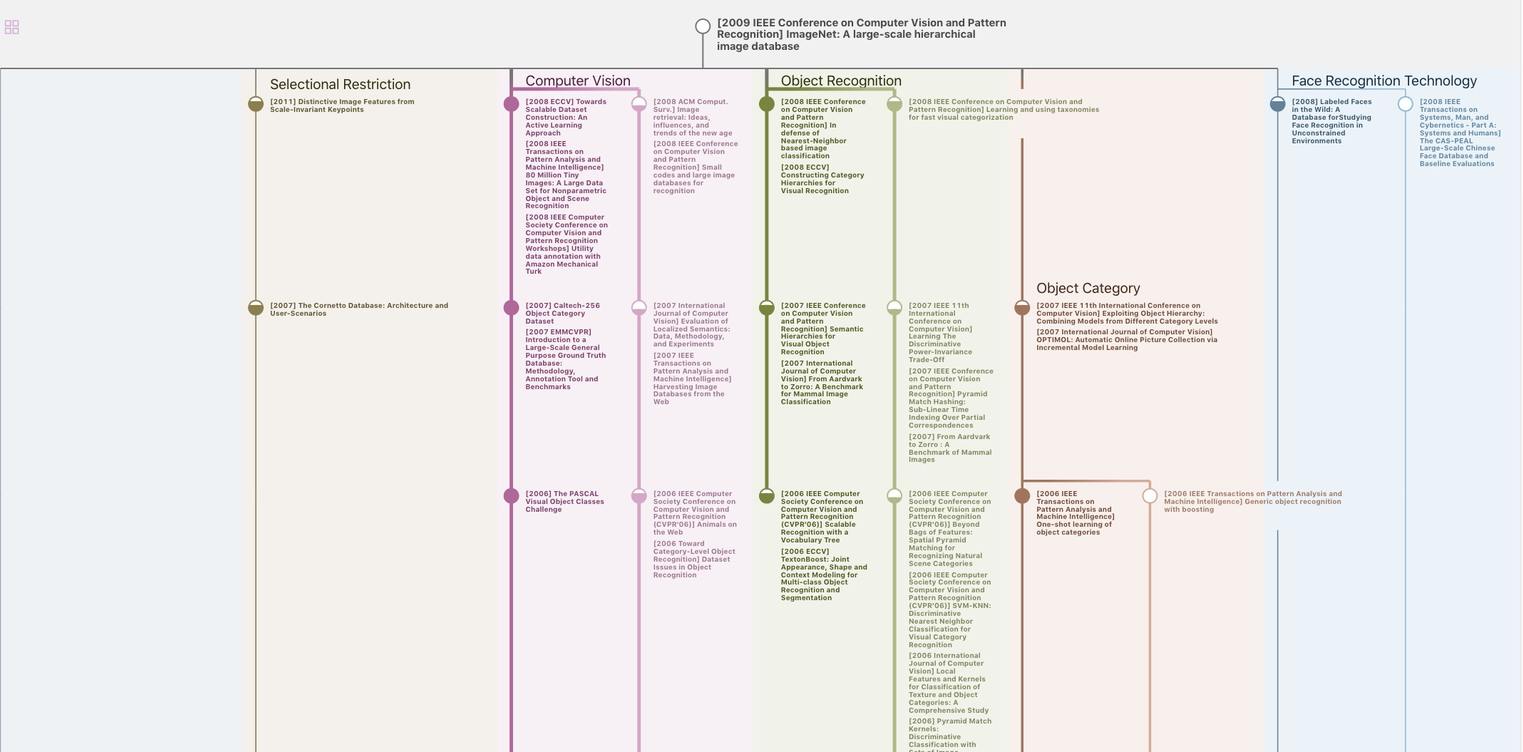
生成溯源树,研究论文发展脉络
Chat Paper
正在生成论文摘要