3D U-net for Registration of Lung Nodules in Longitudinal CT Scans
Medical Imaging(2021)
摘要
The use of low-dose Computed Tomography (CT) has been effective in reducing the mortality rate due to lung cancer. With the rapid increase in the number of studies, computer aided diagnosis (CAD) systems need to be developed to further assist radiologists in detecting lung nodules and determining their malignancy in CT scans. An important factor for determining malignancy in a sequential of scans is the rate of change and growth. In the past, deformable registration techniques have been widely applied to assess longitudinal change in data. In this paper we propose a new deep learning system based on Convolutional Neural Networks and U-Net architecture to assess longitudinal change in CT scans for a nodule over time. The VoxelMorph network [1] has been effective in deformable registration of brain MRIs. This paper extends the application of VoxelMorph to registration of lung nodules in multiple time point scans based on a subset of data from the National Lung Screening Trial (NLST), referred to as NLSTx. VoxelMorph has been modified to include both a sum-of-squared-differences (SSD) image similarity loss and spatial domain regularization to reduce the number of fold-overs and non-invertible transformations, indicated by the presence of negative Jacobian determinants. The network effectively performs 3D Deformable Registration of longitudinal nodule CT scans, providing the means to quantify nodule growth over time.
更多查看译文
AI 理解论文
溯源树
样例
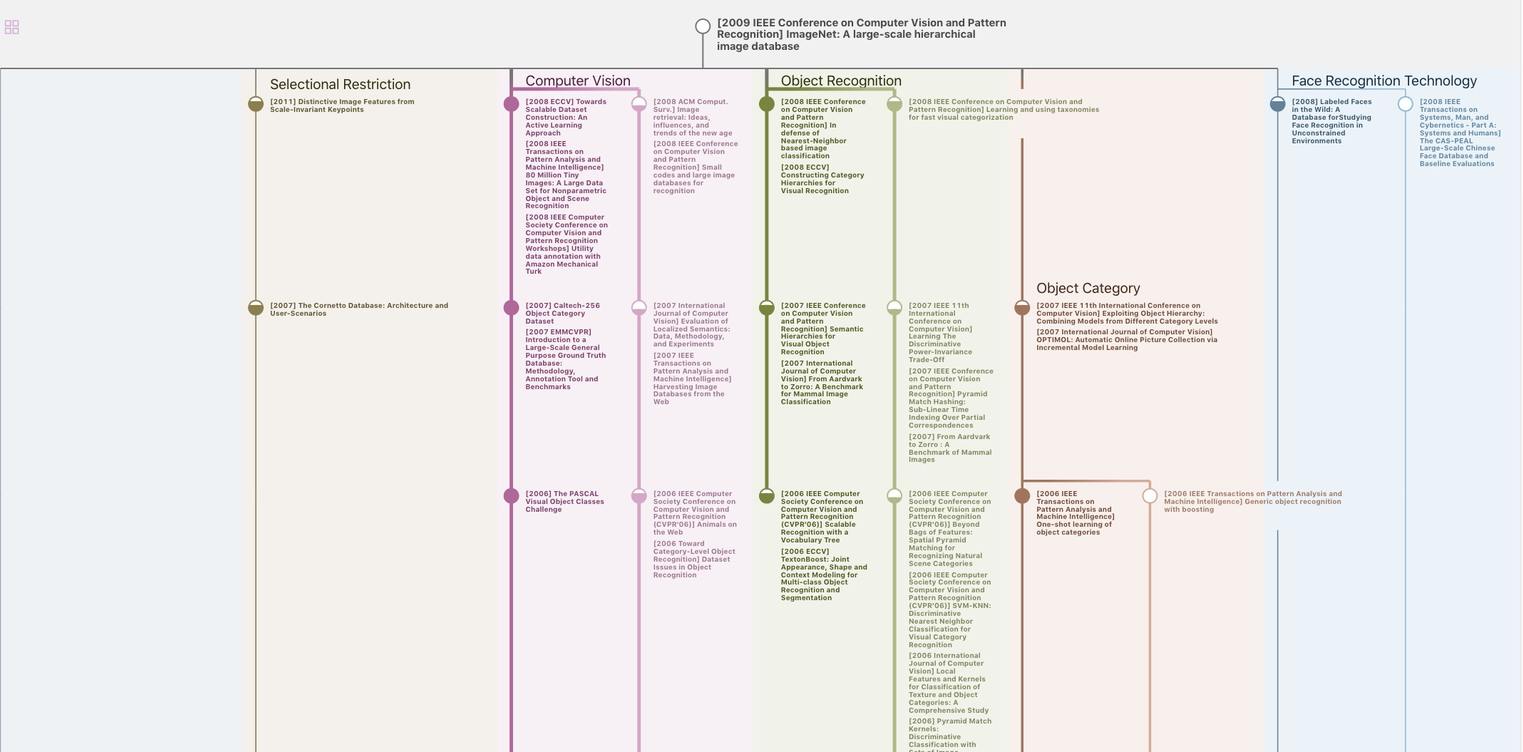
生成溯源树,研究论文发展脉络
Chat Paper
正在生成论文摘要