Estimating near term breast cancer risk from sequential mammograms using deep learning, Radon Cumulative Distribution Transform, and a clinical risk factor: preliminary analysis
MEDICAL IMAGING 2021: COMPUTER-AIDED DIAGNOSIS(2021)
摘要
Our ultimate goal is to develop a personalized breast screening model. This model will provide information to an individual woman for planning her own screening options based on her imaging and other characteristics. To do so, we are developing a computerized method to estimate a near term risk of screen detectable breast cancer (sBCa) by analyzing lateral and temporal differences in screening mammograms using the Radon Cumulative Distribution Transform (RCDT), a deep convolutional neural network (dCNN). The RCDT can highlight subtle signals associated with future sBCa by exploiting lateral and temporal differences in screening mammograms of women. We used a dCNN to build a robust classification marker of having sBCa within a year from a negative screening. We explored the potential for incremental improvement over known risk factors by combining the dCNN with age and showed consistent gains in identifying asymptomatic women with a low risk of having sBCa (who could use the personalized screening option to safely skip their next screening). We used 4296 screening mammogram images of 537 women with 151 sBCa cases and fine-tuned the VGG16 network using a data split ratio of 0.64:0.16:0.2 for training, validation, and testing. The final model on the independent testing dataset achieved an AUC of 0.82, and a specificity at a sensitivity level of 98% (Sp@Se98) of 0.51 with a 95% confidence interval of 0.40 to 0.65. This indicates that the model could reliably identify at least 40% of low-risk women with a 2% error for a false negative rate.
更多查看译文
关键词
Breast cancer,near term risk estimation,deep learning,mammogram
AI 理解论文
溯源树
样例
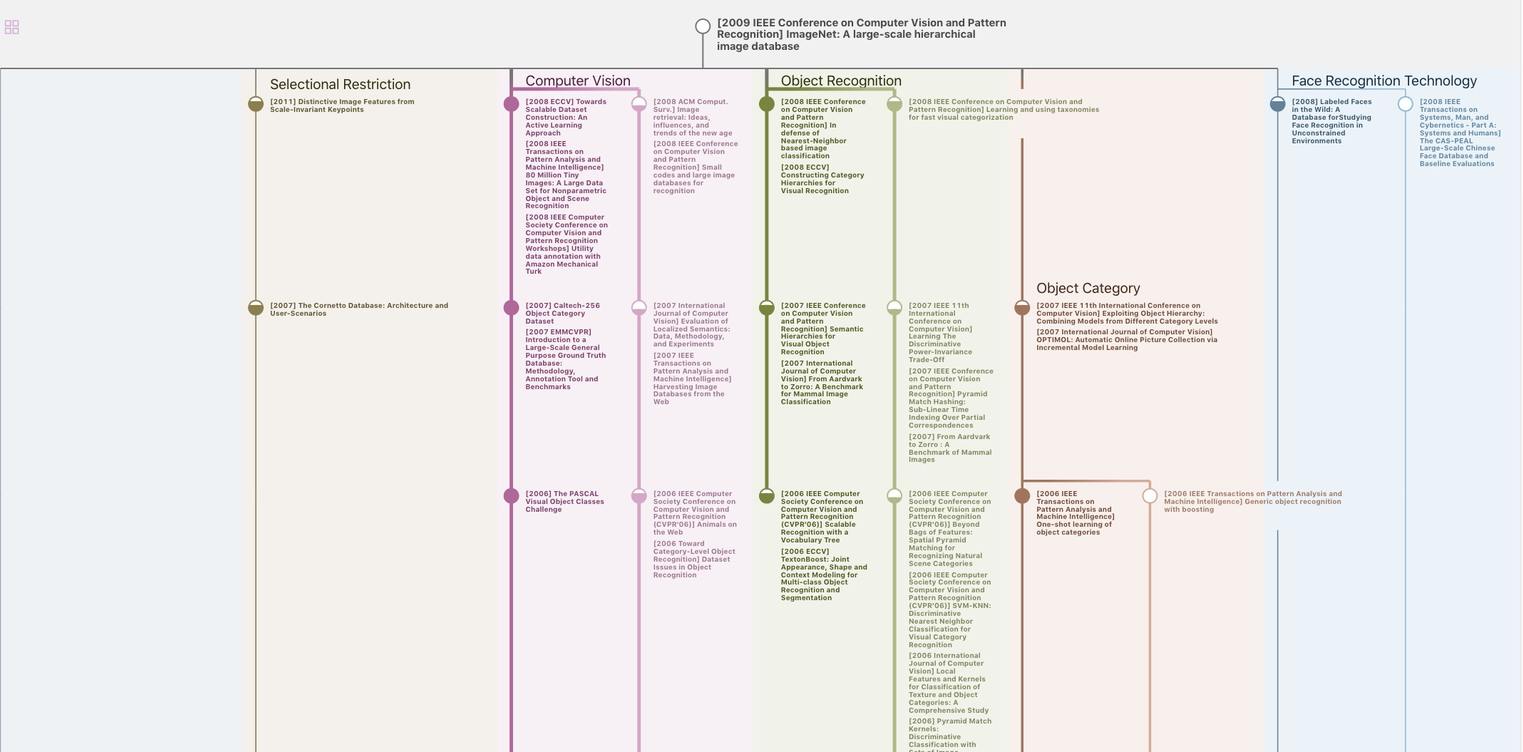
生成溯源树,研究论文发展脉络
Chat Paper
正在生成论文摘要