COVID-19 Opacity Segmentation in Chest CT Via HydraNet: a Joint Learning Multi-Decoder Network.
MEDICAL IMAGING 2021 COMPUTER-AIDED DIAGNOSIS(2021)
摘要
The outbreak of the coronavirus and its rapid spread was recently acknowledged as a worldwide pandemic. Chest CT scans show high potential for detecting pathological manifestations. Hence, the demand for computer-aided tools to support radiologists has grown exponentially. In this work, we developed a deep learning based algorithm, with an emphasis on novel transfer learning methods, to segment COVID-19 opacity in chest CT scans. Our method focuses on creating a deep encoder for feature extraction by using a Fully Convolutional Network (FCN) architecture with one shared encoder and N task-related decoders, named HydraNet. The HydraNet architecture allowed the leverage of a large variety of medical datasets from different domains, in order to achieve better performances on a limited dataset. We achieved a dice score, sensitivity, and precision of 0.724, 0.75, and 0.807 respectively, on the test set, which is competitive with known state-of-the-art results.
更多查看译文
关键词
COVID-19,Chest CT,Lung,AI,Deep Learning,Transfer Learning
AI 理解论文
溯源树
样例
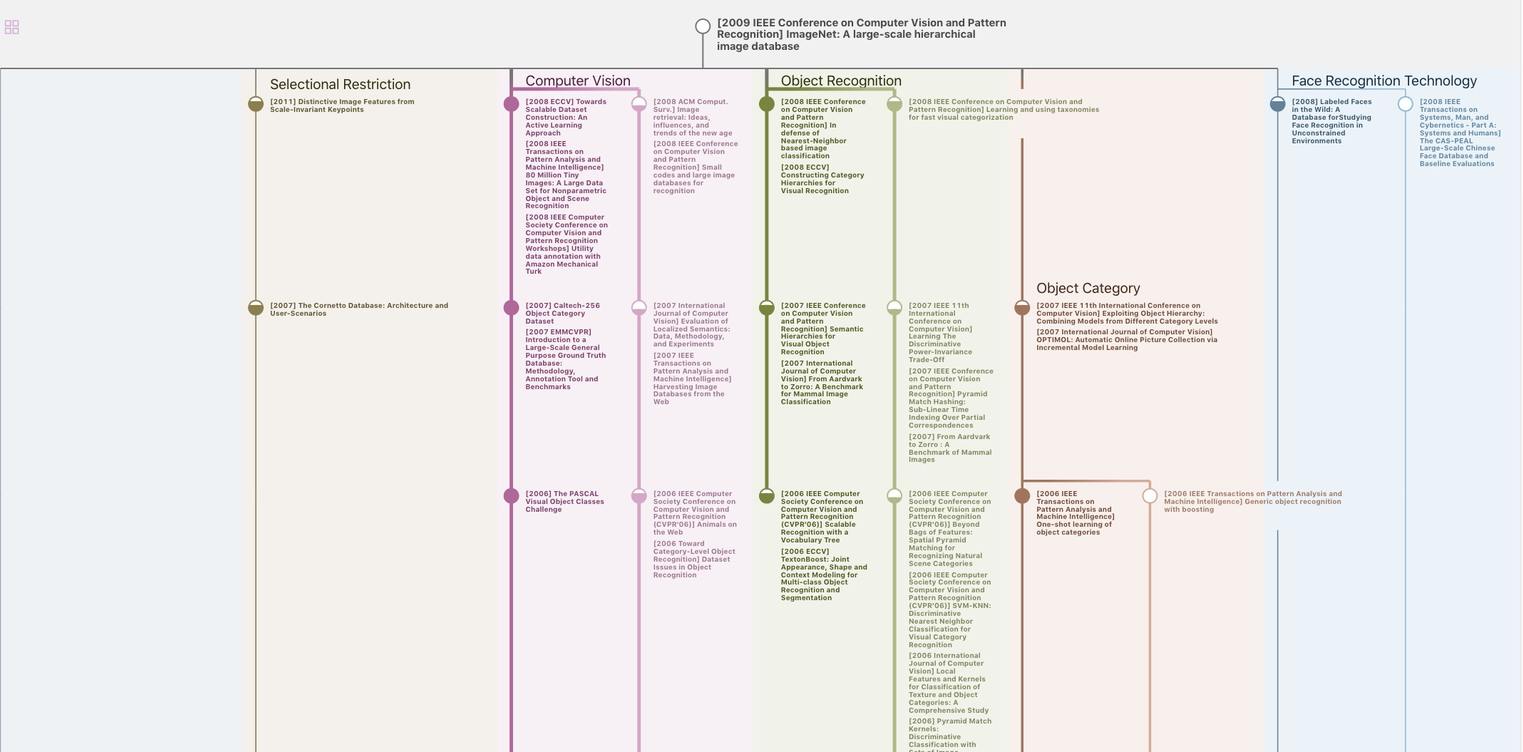
生成溯源树,研究论文发展脉络
Chat Paper
正在生成论文摘要