A vertebral level-wise data augmentation scheme for segmentation via deep learning
MEDICAL IMAGING 2021: IMAGE PROCESSING(2021)
摘要
Accurate vertebral segmentation is important for image-related assessment of spine pathologies. Recent spine segmentation techniques focus on deep learning-based methods. However, they typically require a large training dataset with accurate annotations, which may not be feasible in practice. To mitigate the challenge, here we propose a data augmentation scheme based on a single spine to incorporate biomechanically constrained intervertebral motion (rotation and translation) to generate a large training dataset (N=3000). Vertebrae are then segmented using a point cloud-based deep learning architecture, PointNet++, which appears not to have been applied to spine segmentation before. Spine testing samples (N=90) are first generated by applying our data augmentation technique to three separate spines. Segmentation performances are compared with two baseline data augmentation methods that do not include intervertebral motion. Then, we further evaluate our technique based on 8 spine samples from actual image acquisitions. In both cases, we show that incorporation of vertebral level-wise data augmentation improves segmentation accuracy in terms of mean Dice coefficient (e.g., 0.932 vs. 0.902 for augmented testing samples and 0.940 vs. 0.924 for acquired testing samples). These results suggest that our vertebral level-wise data augmentation is useful to facilitate deep learning-based spine segmentation, which is important especially when it is not feasible to generate accurate annotations for a large number of training samples.
更多查看译文
关键词
Spine segmentation,data augmentation,computed tomography,point clouds
AI 理解论文
溯源树
样例
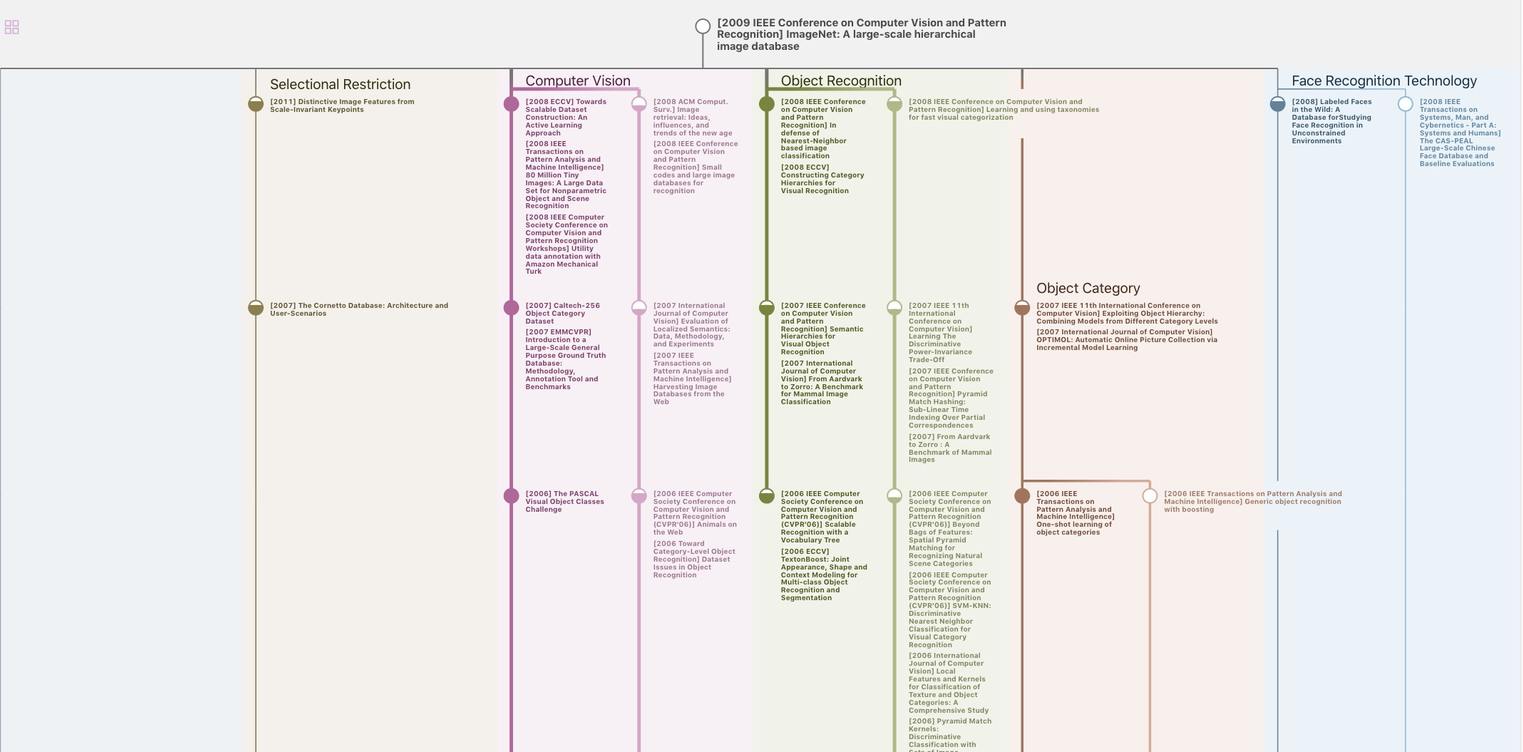
生成溯源树,研究论文发展脉络
Chat Paper
正在生成论文摘要