Low-Cost Network Scheduling Of 3d-Cnn Processing For Embedded Action Recognition
IEEE ACCESS(2021)
摘要
The recent 3D convolutional neural network (3D-CNN) is a promising candidate for solving the action recognition problem by providing attractive algorithm-level performance. Due to the excessive amount of computational costs, however, it is almost impractical to apply the advanced 3D-CNN architecture to the resource-limited real-time embedded system. In this work, we present several optimization schemes that can relax the complexity of 3D-CNN processing without sacrificing recognition accuracy. More precisely, we first develop several 3D-CNN architectures for exploiting the trade-off between the network complexity and recognition performance. Evaluating the current confidential level, then, the proposed method dynamically changes the network structure to be used for the next clip-level inference. In addition, we introduce a systematic way of managing the network sequence for minimizing the computing overheads while supporting the acceptable algorithm-level performance. Compared to the previous works, as a result, the proposed approaches drastically relax the processing costs as well as the energy consumption by selecting the simplest 3D-CNN architecture at the run time, allowing the cost-effective action recognition for embedded edges.
更多查看译文
关键词
Three-dimensional displays, Heuristic algorithms, Convolution, Sports, Memory management, Dynamic scheduling, Computational efficiency, 3D-CNN architecture, action recognition, video analysis, embedded systems
AI 理解论文
溯源树
样例
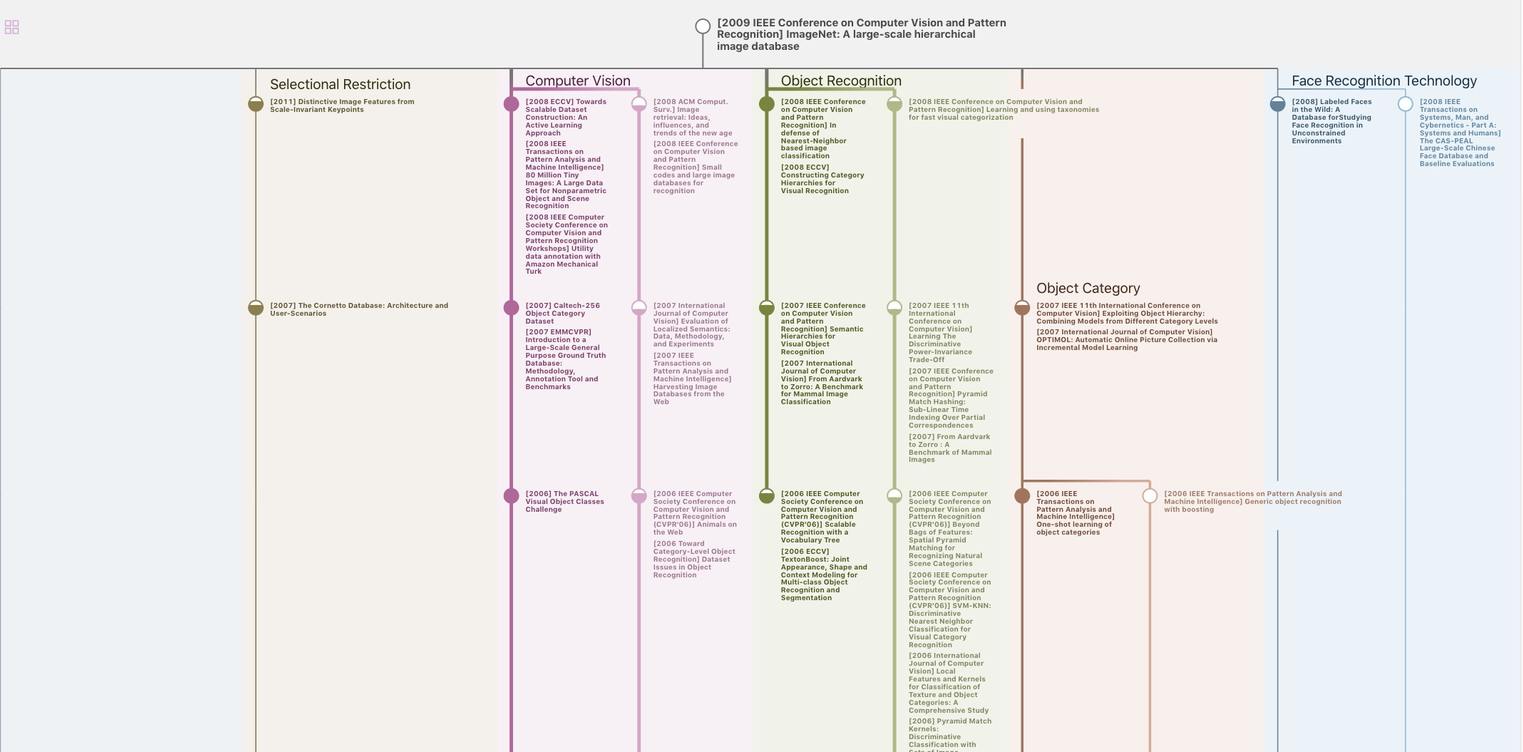
生成溯源树,研究论文发展脉络
Chat Paper
正在生成论文摘要