Quantifying plant mimesis in fossil insects using deep learning
HISTORICAL BIOLOGY(2022)
摘要
As an important combination of behaviour and pattern in animals to resemble benign objects, biolog ical mimesis can effectively avoid the detection of their prey and predators. It at least dates back to the Permian in fossil records. The recognition of mimesis within fossil, however, might be subjective and lack quantitative analysis being only based on few fossils with limited information. To compensate for this omission, we propose a new method using a Siamese network to measure the dissimilarity between hypothetical mimics and their models from images. It generates dissimilarity values between paired images of organisms by extracting feature vectors and calculating Euclidean distances. Additionally, the idea of 'transfer learning' is adopted to fine-tune the Siamese network, to overcome the limitations of available fossil image pairs. We use the processed Totally-Looks-Like, a large similar image data set, to pretrain the Siamese network and fine-tune it with a collected mimetic-image data set. Based on our results, we propose two recommended image dissimilarity thresholds for judging the mimicry of extant insects (0-0.4556) and fossil insects (0-0.4717). Deep learning algorithms are used to quantify the mimicry of fossil insects in this study, providing novel insights into exploring the early evolution of mimicry.
更多查看译文
关键词
Mimesis, fossil insects, similarity, deep learning, Siamese network
AI 理解论文
溯源树
样例
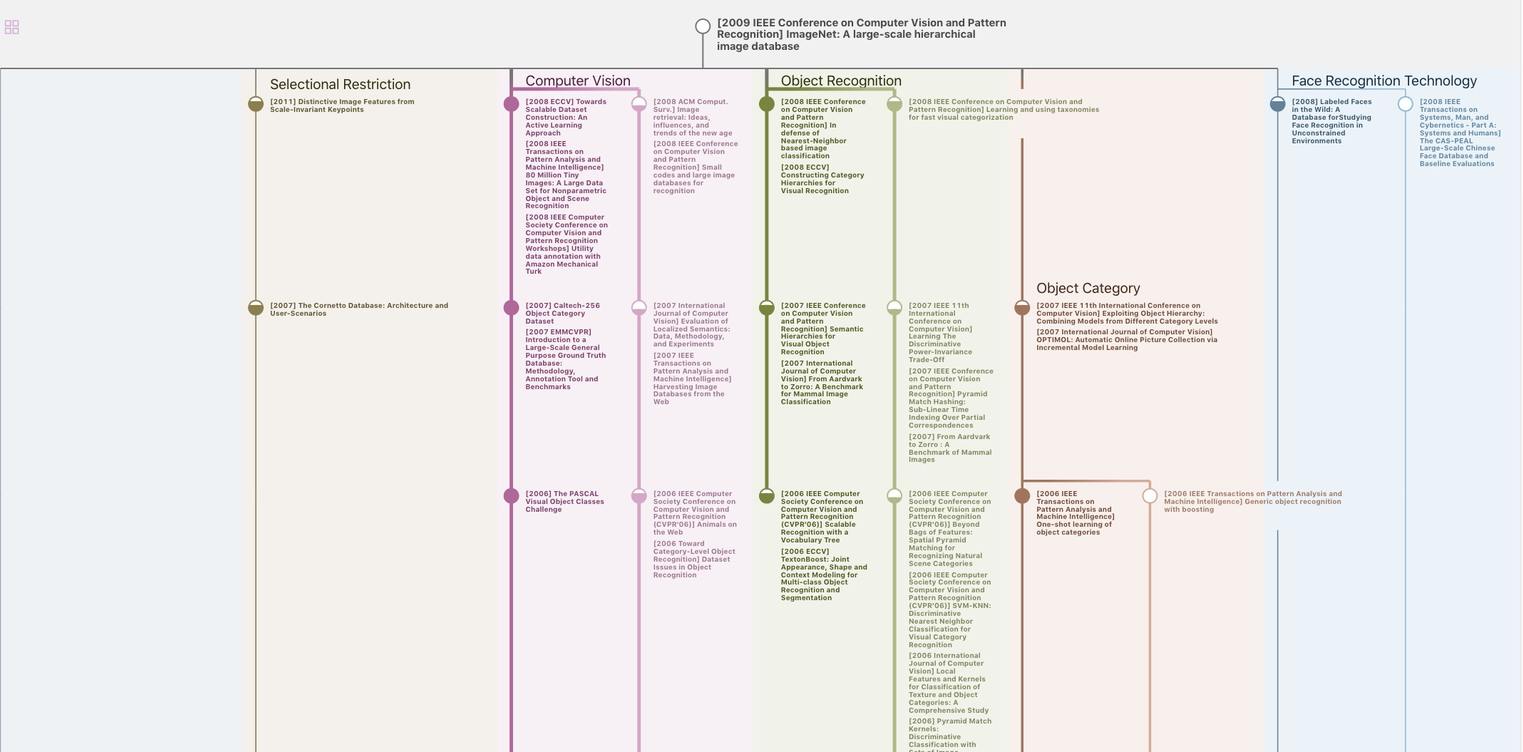
生成溯源树,研究论文发展脉络
Chat Paper
正在生成论文摘要