Quantification And Reduction Of Uncertainties In A Wind Turbine Numerical Model Based On A Global Sensitivity Analysis And A Recursive Bayesian Inference Approach
INTERNATIONAL JOURNAL FOR NUMERICAL METHODS IN ENGINEERING(2021)
摘要
A framework to perform quantification and reduction of uncertainties in a wind turbine numerical model using a global sensitivity analysis and a recursive Bayesian inference method is developed in this article. We explain how a prior probability distribution on the model parameters is transformed into a posterior probability distribution, by incorporating a physical model and real field noisy observations. Nevertheless, these approaches suffer from the so-called curse of dimensionality. In order to reduce the dimension, Sobol' indices approach for global sensitivity analysis, in the context of wind turbine modeling, is presented. A major issue arising for such inverse problems is identifiability, that is, whether the observations are sufficient to unambiguously determine the input parameters that generated the observations. Global sensitivity analysis is also used in the context of identifiability.
更多查看译文
关键词
Global sensitivity analysis, inference, model calibration, nonlinear Kalman filters, uncertainty quantification
AI 理解论文
溯源树
样例
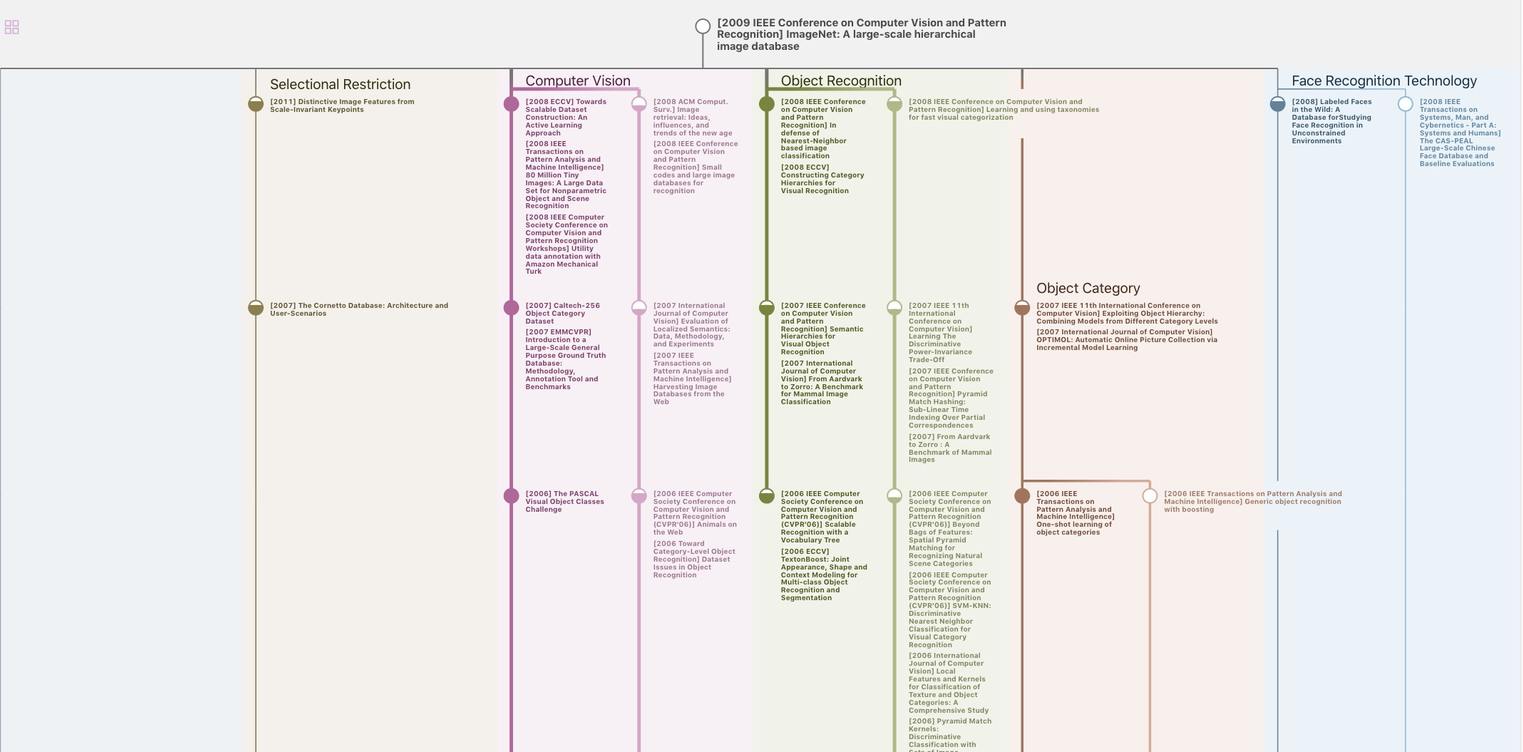
生成溯源树,研究论文发展脉络
Chat Paper
正在生成论文摘要