Fawos: Fairness-Aware Oversampling Algorithm Based On Distributions Of Sensitive Attributes
IEEE ACCESS(2021)
摘要
With the increased use of machine learning algorithms to make decisions which impact people's lives, it is of extreme importance to ensure that predictions do not prejudice subgroups of the population with respect to sensitive attributes such as race or gender. Discrimination occurs when the probability of a positive outcome changes across privileged and unprivileged groups defined by the sensitive attributes. It has been shown that this bias can be originated from imbalanced data contexts where one of the classes contains a much smaller number of instances than the other classes. It is also important to identify the nature of the imbalanced data, including the characteristics of the minority classes' distribution. This paper presents FAWOS: a Fairness-Aware oversampling algorithm which aims to attenuate unfair treatment by handling sensitive attributes' imbalance. We categorize different types of datapoints according to their local neighbourhood with respect to the sensitive attributes, identifying which are more difficult to learn by the classifiers. In order to balance the dataset, FAWOS oversamples the training data by creating new synthetic datapoints using the different types of datapoints identified. We test the impact of FAWOS on different learning classifiers and analyze which can better handle sensitive attribute imbalance. Empirically, we observe that this algorithm can effectively increase the fairness results of the classifiers while not neglecting the classification performance. Source code can be found at: https://github.com/teresalazar13/FAWOS
更多查看译文
关键词
Prediction algorithms, Licenses, Training data, Support vector machines, Interpolation, Informatics, Training, Classification bias, fairness, imbalanced data, K-nearest neighborhood, oversampling
AI 理解论文
溯源树
样例
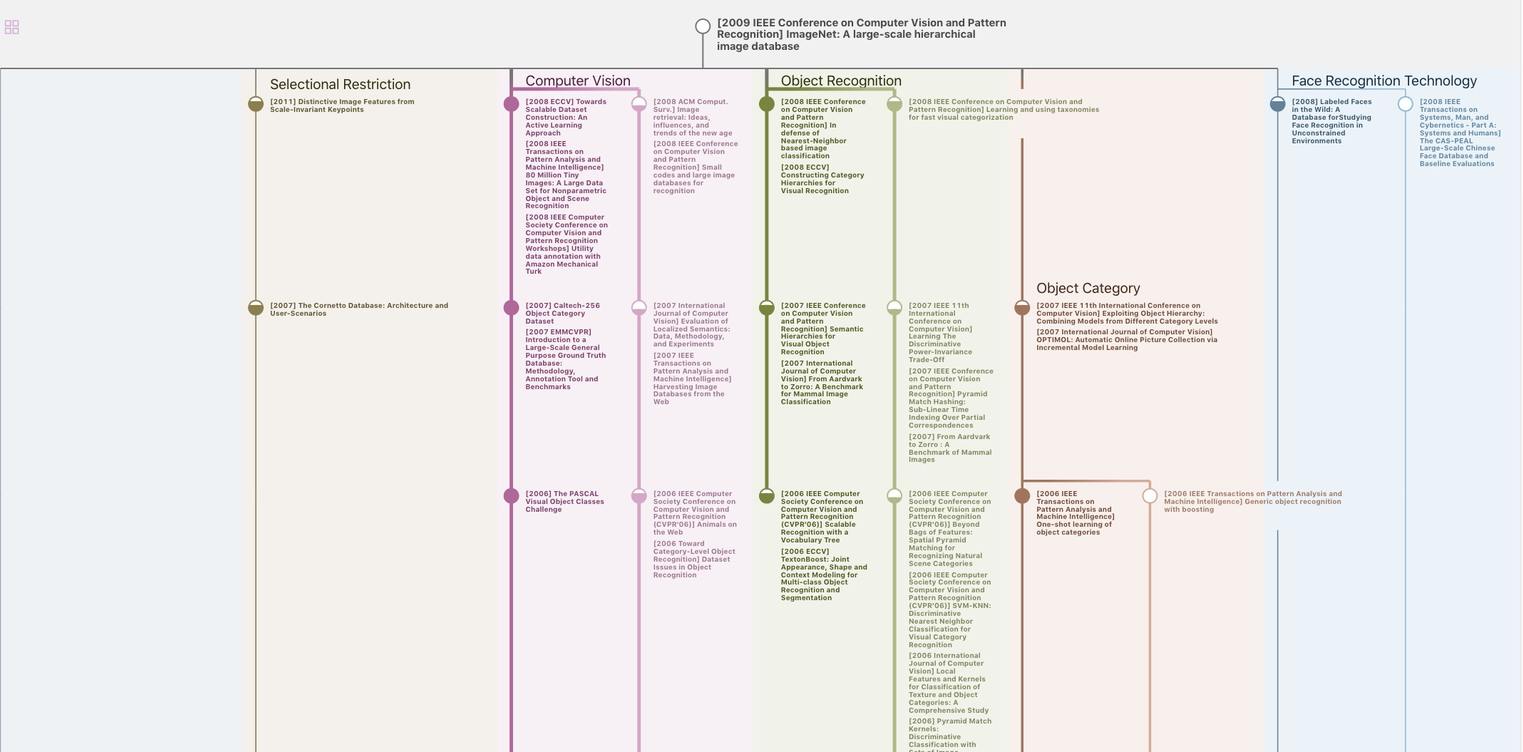
生成溯源树,研究论文发展脉络
Chat Paper
正在生成论文摘要