Block-Attentive Subpixel Prediction Networks For Computationally Efficient Image Restoration
IEEE ACCESS(2021)
摘要
Image restoration based on the Deep Convolutional Neural Network (CNN) based image restoration has demonstrated promising results in many sub-tasks, such as image super-resolution, compression artifacts removal, image denoising, and image enhancement. Compared to many CNN-based high-level vision tasks that predict sparse probabilities of each class, the CNN for image restoration requires dense pixel-level predictions with precise intensity-level values. Therefore, a minimum number of spatial pooling (or down-sampling) operations is required to maintain the image details. Therefore, designing a fast or lightweight model for image restoration is a difficult problem and is even more critical when the spatial resolution of an image becomes larger. In this paper, we propose a family of networks called Subpixel Prediction Networks (SPNs) that predict reshaped and spatially down-sampled block-wise tensors instead of raw images with full resolution. Under this scheme, spatial downsampling decreases the restoration performance less while making the network faster. We propose a novel Subpixel Block Attention (SBA) module that re-calibrates blockwise features to diminish blockwise discontinuity to increase the performance further. The experimental results reveal that these networks demonstrate good trade-offs between speed (number of computations) and restoration performance in the three image restoration tasks: image compression artifacts removal, color image denoising, and image enhancement.
更多查看译文
关键词
Image restoration, Task analysis, Spatial resolution, Image coding, Convolution, Image enhancement, Image denoising, Image restoration, image compression artifacts removal, image denoising, image enhancement, fast deep neural networks
AI 理解论文
溯源树
样例
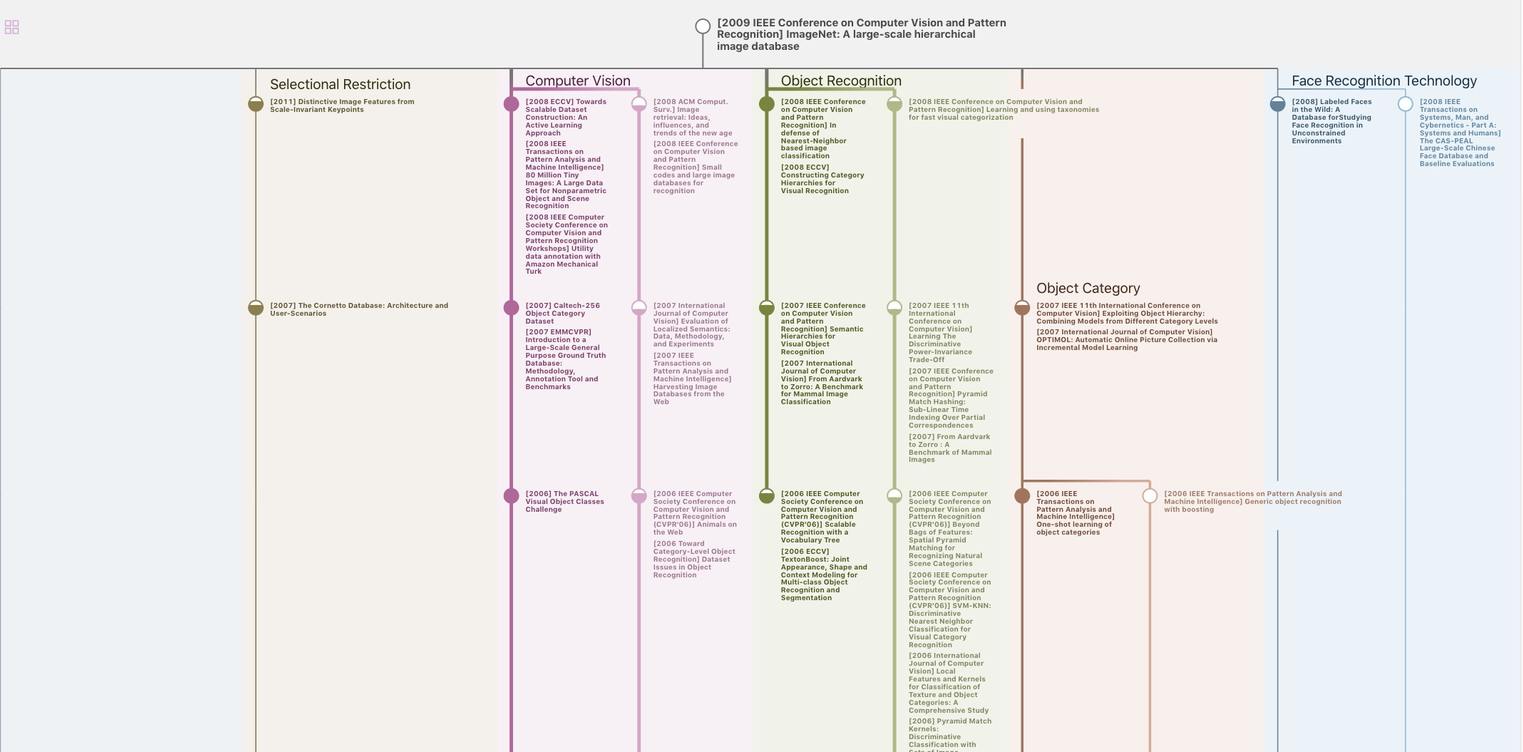
生成溯源树,研究论文发展脉络
Chat Paper
正在生成论文摘要