Streetgan: Towards Road Network Synthesis With Generative Adversarial Networks
25. INTERNATIONAL CONFERENCE IN CENTRAL EUROPE ON COMPUTER GRAPHICS, VISUALIZATION AND COMPUTER VISION (WSCG 2017)(2017)
摘要
We propose a novel example-based approach for road network synthesis relying on Generative Adversarial Networks (GANs), a recently introduced deep learning technique. In a pre-processing step, we first convert a given representation of a road network patch into a binary image where pixel intensities encode the presence or absence of streets. We then train a GAN that is able to automatically synthesize a multitude of arbitrary sized street networks that faithfully reproduce the style of the original patch. In a post-processing step, we extract a graph-based representation from the generated images. In contrast to other methods, our approach does neither require domain-specific expert knowledge, nor is it restricted to a limited number of street network templates. We demonstrate the general feasibility of our approach by synthesizing street networks of largely varying style and evaluate the results in terms of visual similarity as well as statistical similarity based on road network similarity measures.
更多查看译文
关键词
deep learning, generative modeling, generative adversarial networks (GANs), road network generation
AI 理解论文
溯源树
样例
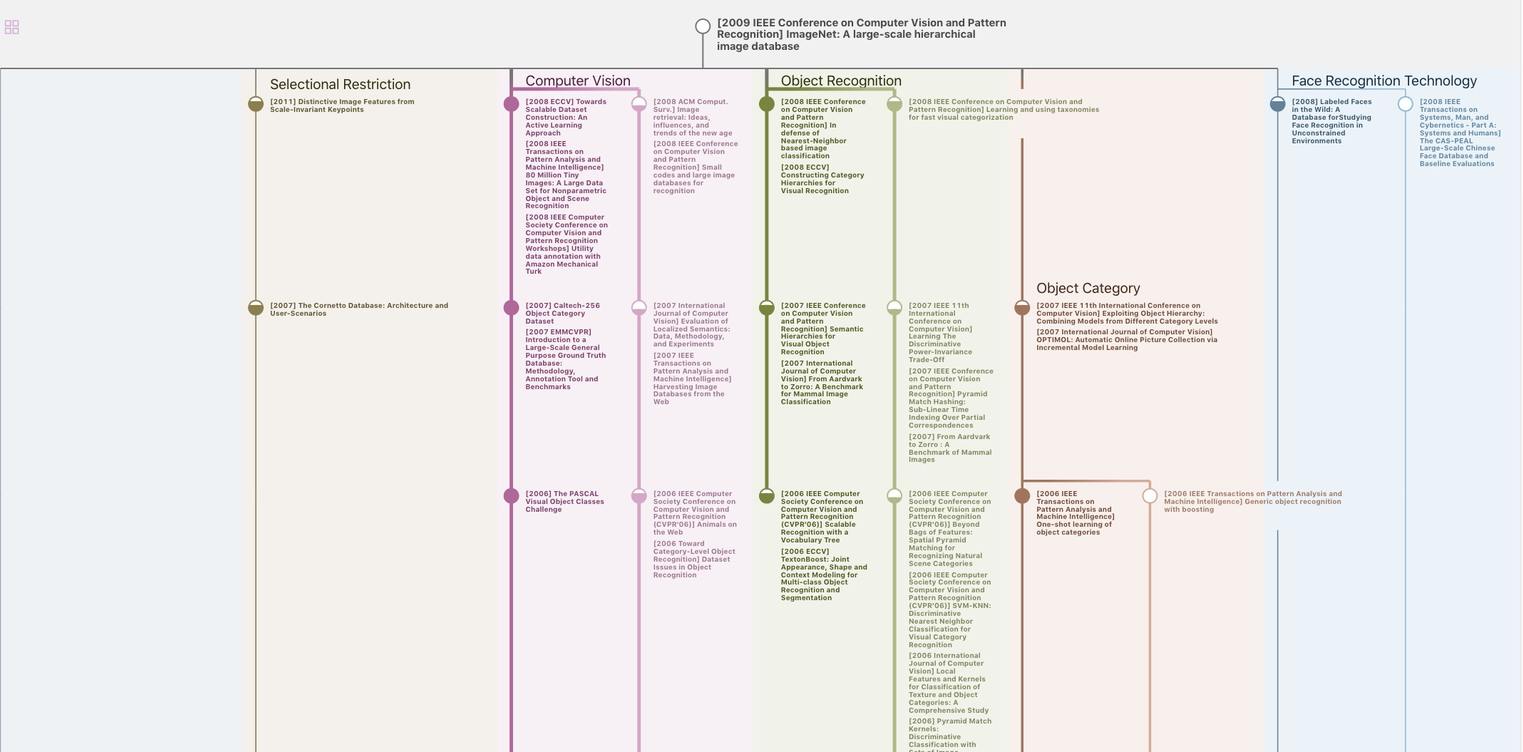
生成溯源树,研究论文发展脉络
Chat Paper
正在生成论文摘要