Riemannian Multi-Manifold Modeling and Clustering in Brain Networks
Wavelets and Sparsity XVII(2017)
摘要
This paper introduces Riemannian multi-manifold modeling in the context of brain-network analytics: Brain network time-series yield features which are modeled as points lying in or close to a union of a finite number of submanifolds within a known Riemannian manifold. Distinguishing disparate time series amounts thus to clustering multiple Riemannian submanifolds. To this end, two feature-generation schemes for brain-network time series are put forth. The first one is motivated by Granger-causality arguments and uses an auto-regressive moving average model to map low-rank linear vector subspaces, spanned by column vectors of appropriately defined observability matrices, to points into the Grassmann manifold. The second one utilizes (non-linear) dependencies among network nodes by introducing kernel-based partial correlations to generate points in the manifold of positive definite matrices. Based on recently developed research on clustering Riemannian submanifolds, an algorithm is provided for distinguishing time series based on their Riemannian-geometry properties. Numerical tests on time series, synthetically generated from real brain-network structural connectivity matrices, reveal that the proposed scheme outperforms classical and state-of-the-art techniques in clustering brain-network states/structures.
更多查看译文
关键词
Time series,(brain) networks,Riemannian manifold,clustering,ARMA model,partial correlations,kernels
AI 理解论文
溯源树
样例
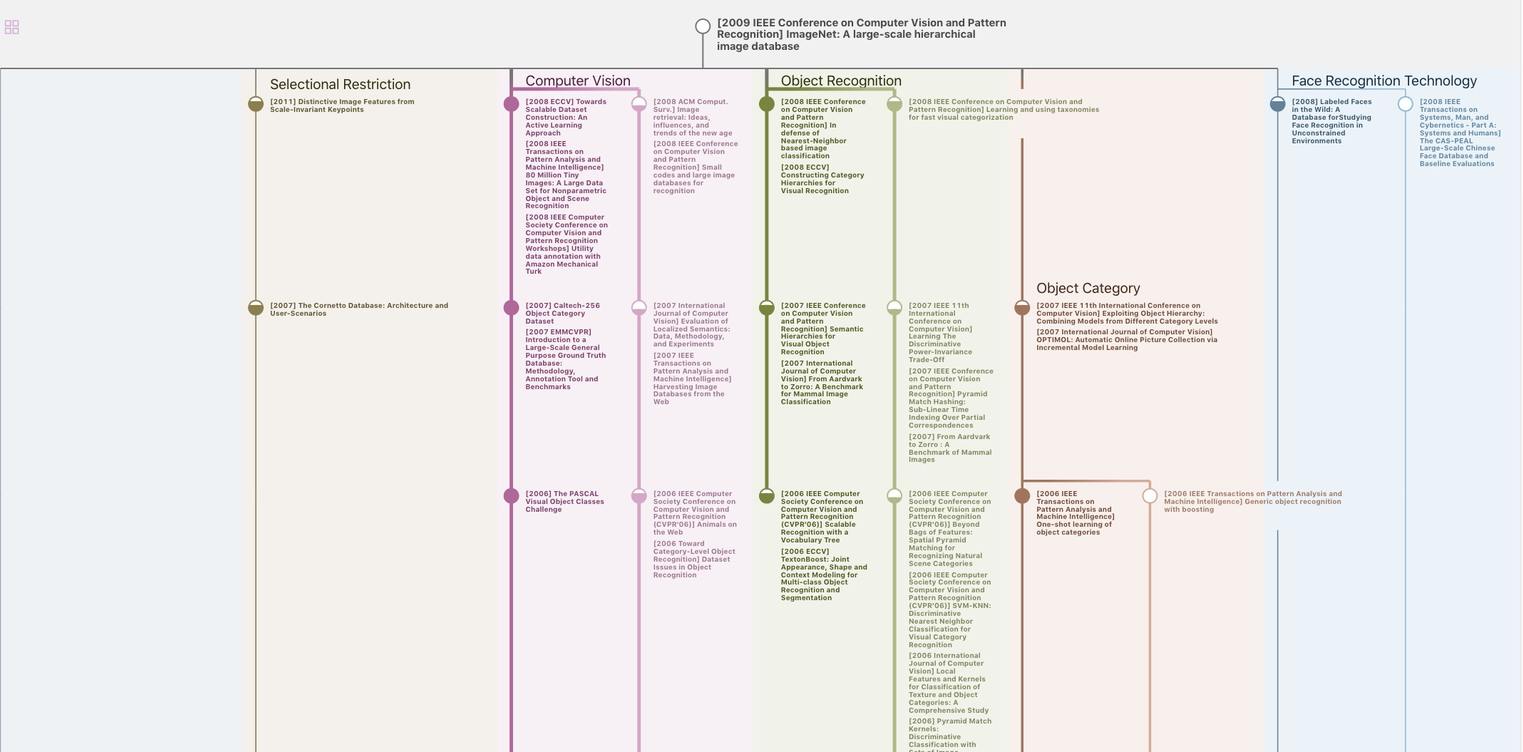
生成溯源树,研究论文发展脉络
Chat Paper
正在生成论文摘要