Deep Reinforcement Learning For Minimizing Tardiness In Parallel Machine Scheduling With Sequence Dependent Family Setups
IEEE ACCESS(2021)
摘要
Parallel machine scheduling with sequence-dependent family setups has attracted much attention from academia and industry due to its practical applications. In a real-world manufacturing system, however, solving the scheduling problem becomes challenging since it is required to address urgent and frequent changes in demand and due-dates of products. To minimize the total tardiness of the scheduling problem, we propose a deep reinforcement learning (RL) based scheduling framework in which trained neural networks (NNs) are able to solve unseen scheduling problems without re-training even when such changes occur. Specifically, we propose state and action representations whose dimensions are independent of production requirements and due-dates of jobs while accommodating family setups. At the same time, an NN architecture with parameter sharing was utilized to improve the training efficiency. Extensive experiments demonstrate that the proposed method outperforms the recent metaheuristics, rule-based, and other RL-based methods in terms of total tardiness. Moreover, the computation time for obtaining a schedule by our framework is shorter than those of the metaheuristics and other RL-based methods.
更多查看译文
关键词
Deep reinforcement learning, unrelated parallel machine scheduling, sequence-dependent family setups, total tardiness objective, deep Q-network
AI 理解论文
溯源树
样例
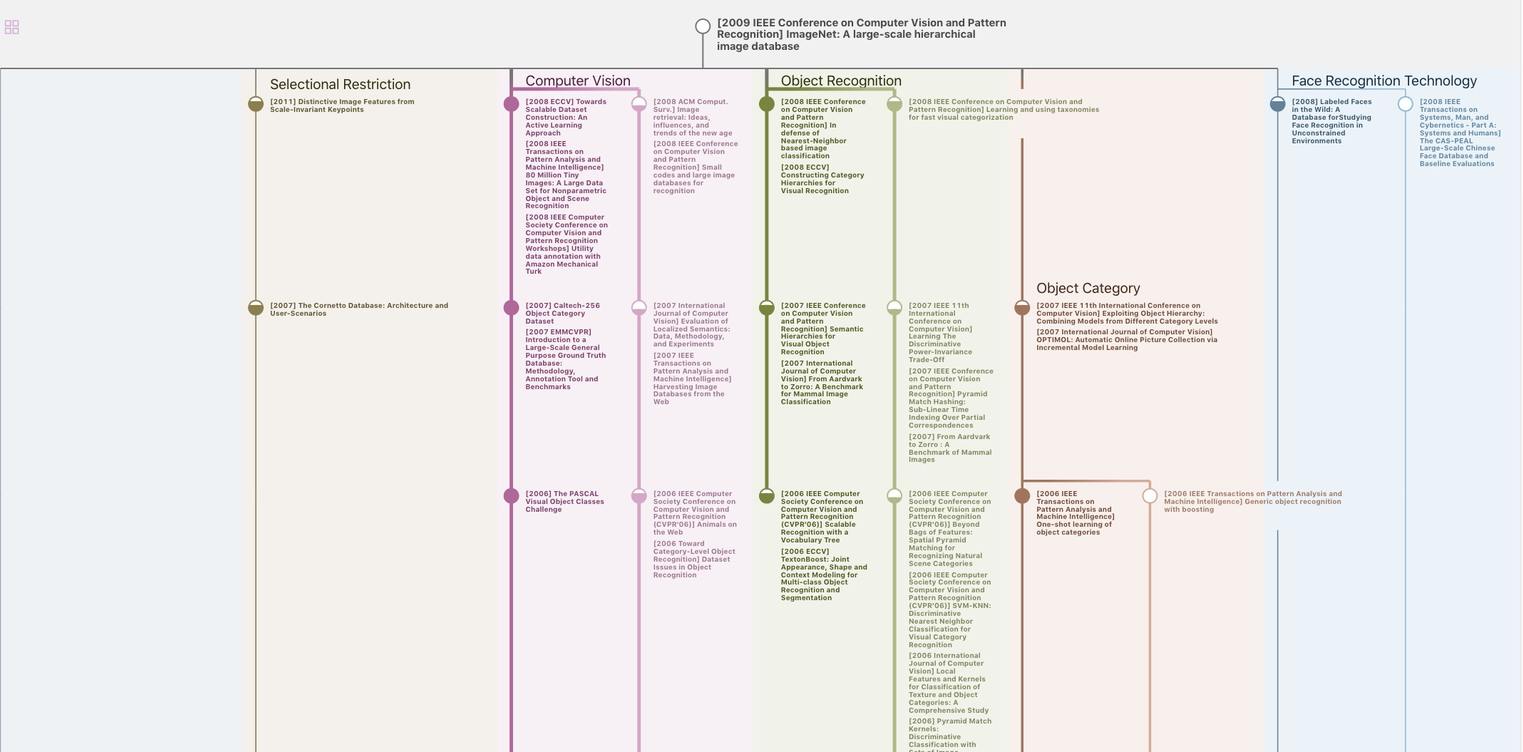
生成溯源树,研究论文发展脉络
Chat Paper
正在生成论文摘要