Probabilistic Decision Model For Adaptive Task Planning In Human-Robot Collaborative Assembly Based On Designer And Operator Intents
IEEE ROBOTICS AND AUTOMATION LETTERS(2021)
摘要
In the manufacturing industry, the era of mass customization has arrived. Combining the complementary strengths of humans and robots will allow to cope with growing product diversity and fluctuating demands. When collaborating, humans and robots operate in a shared workspace to assemble a common product and it has to be decided how tasks are distributed between them. In previous work, human operators have often been modeled as controllable agents whose actions can be planned. To achieve a natural collaboration, we believe that the robot has to estimate the operator's assembly intentions and should behave accordingly. This work presents a framework for planning collaborative robot tasks in assembly, considering both the designer and operator intents. From the designer's CAD data, a set of potential assembly plans is automatically derived and translated into a state graph from which the operator intentions follow. To enable the robot to act optimally under a given belief of operator intentions, a partially observable Markov decision process (POMDP) is formulated, whose state space is represented by the intention graph. Experimental results obtained with our approach demonstrate the potential to estimate the true operator intent based on observations about the parts and tools manipulated during collaborative assembly.
更多查看译文
关键词
Human-robot collaboration, assembly, task planning, intention recognition, probabilistic inference
AI 理解论文
溯源树
样例
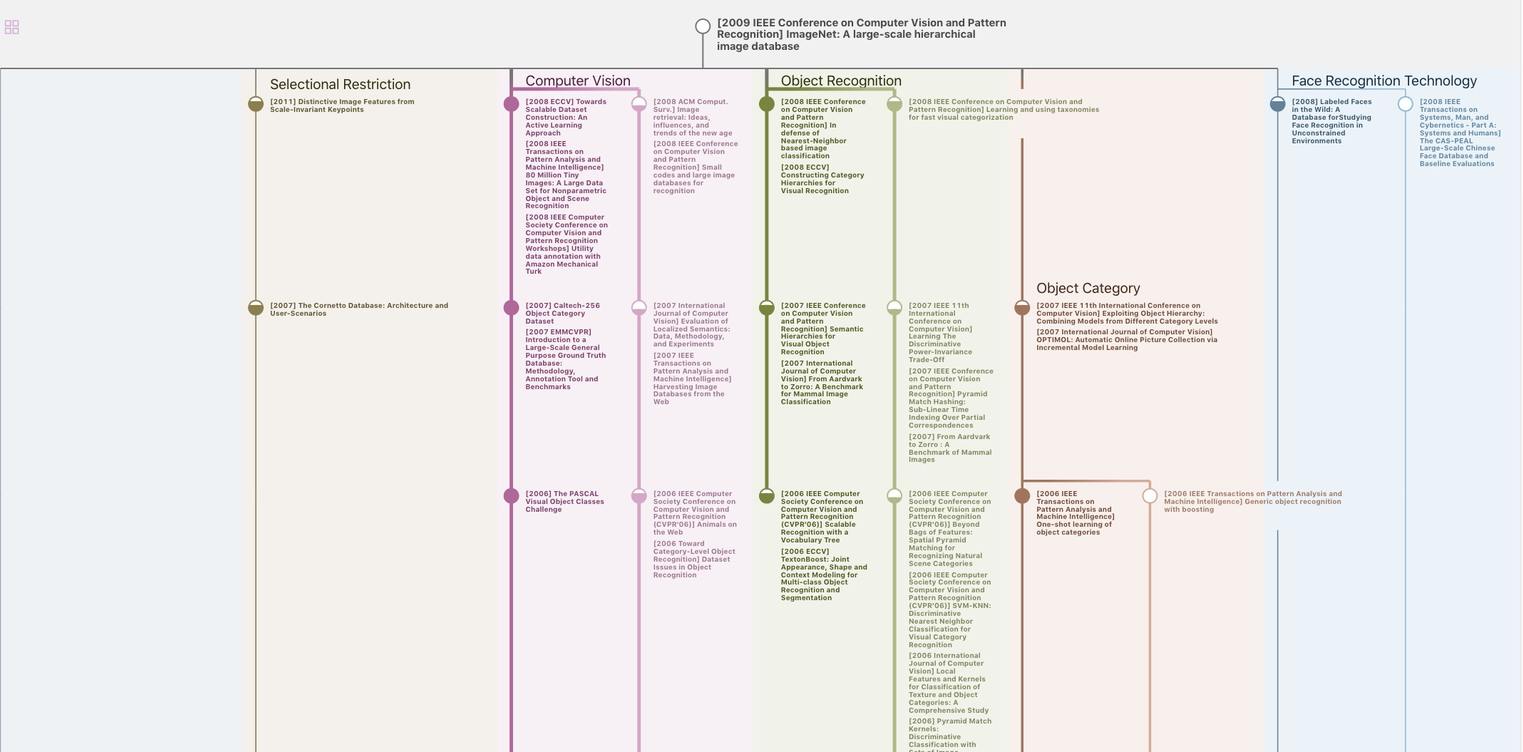
生成溯源树,研究论文发展脉络
Chat Paper
正在生成论文摘要