Value Function Guided Subgroup Identification via Gradient Tree Boosting: A Framework to Handle Multiple Outcomes for Optimal Treatment Recommendation
STATISTICS IN BIOPHARMACEUTICAL RESEARCH(2022)
摘要
In randomized clinical trials, there has been an increasing interest in identifying subgroups with heterogeneous responses to study treatment based on baseline patient characteristics. Even though the benefit risk assessment of any patient population or subgroups is almost always a multi-facet consideration, the statistical literature of subgroup identification has largely been limited to a single clinical outcome. In the article, we propose a nonparametric method that searches for subgroup membership scores by maximizing a value function that directly reflects the subgroup-treatment interaction effect considering clinical priorities of multiple outcomes based on win difference. A gradient tree boosting algorithm is proposed to search for the individual subgroup membership scores. We conduct simulation studies to evaluate the performance of the proposed method and an application to a colon cancer adjuvant clinical trial is performed for illustration.
更多查看译文
关键词
Gradient tree boosting, Nonparametric, Subgroup identification, Win difference
AI 理解论文
溯源树
样例
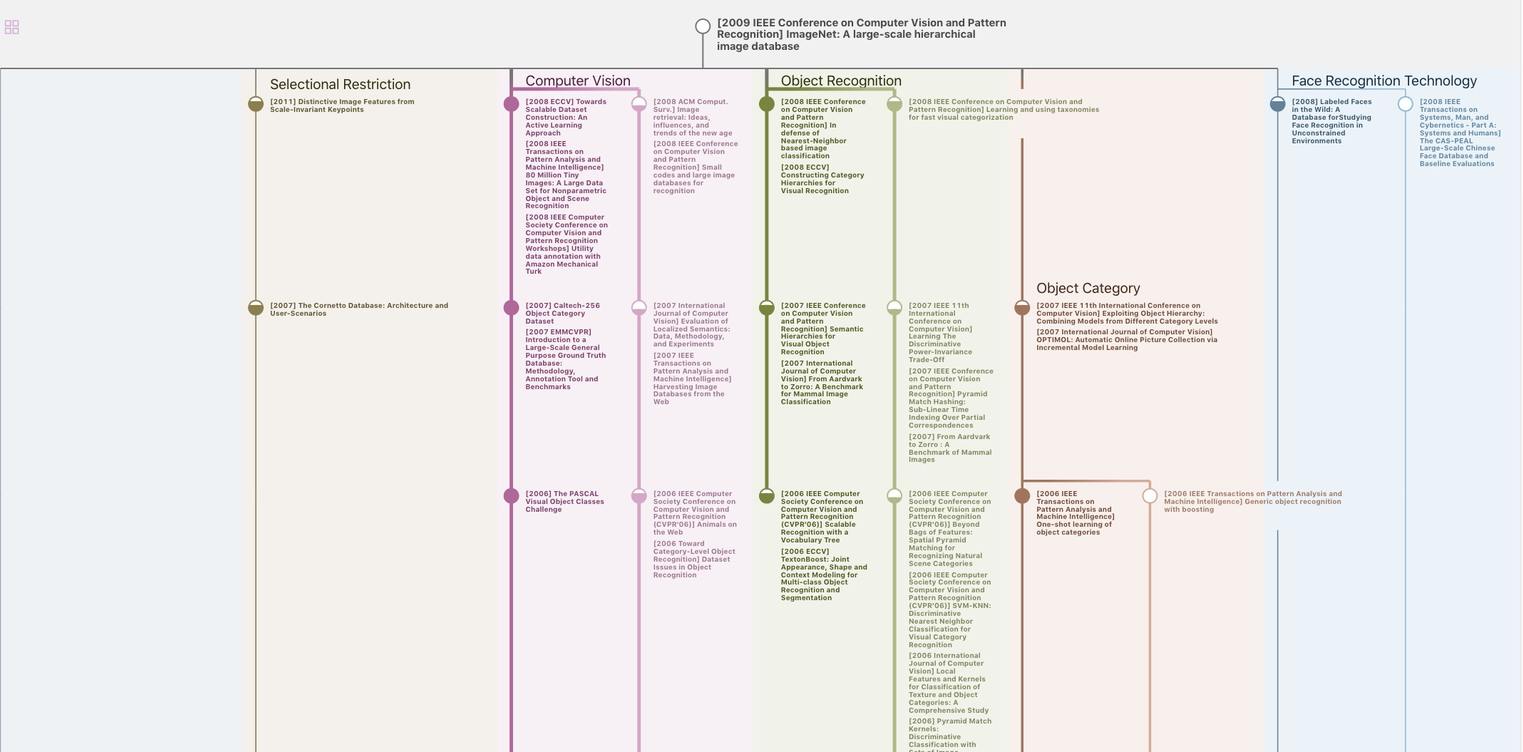
生成溯源树,研究论文发展脉络
Chat Paper
正在生成论文摘要