Assessing autonomous ship navigation using bridge simulators enhanced by cycle-consistent adversarial networks
PROCEEDINGS OF THE INSTITUTION OF MECHANICAL ENGINEERS PART O-JOURNAL OF RISK AND RELIABILITY(2023)
摘要
The advent of artificial intelligence and deep learning has provided sophisticated functionality for sensor fusion and object detection and classification which have accelerated the development of highly automated and autonomous ships as well as decision support systems for maritime navigation. It is, however, challenging to assess how the implementation of these systems affects the safety of ship operation. We propose to utilize marine training simulators to conduct controlled, repeated experiments allowing us to compare and assess how functionality for autonomous navigation and decision support affects navigation performance and safety. However, although marine training simulators are realistic to human navigators, it cannot be assumed that the simulators are sufficiently realistic for testing the object detection and classification functionality, and hence this functionality cannot be directly implemented in the simulators. We propose to overcome this challenge by utilizing Cycle-Consistent Adversarial Networks (Cycle-GANs) to transform the simulator data before object detection and classification is performed. Once object detection and classification are completed, the result is transferred back to the simulator environment. Based on this result, decision support functionality with realistic accuracy and robustness can be presented and autonomous ships can make decisions and navigate in the simulator environment.
更多查看译文
关键词
Simulator-based assessment,autonomous ships,MASS,cycle-GAN,validation and verification,safety assessment,marine bridge training simulators
AI 理解论文
溯源树
样例
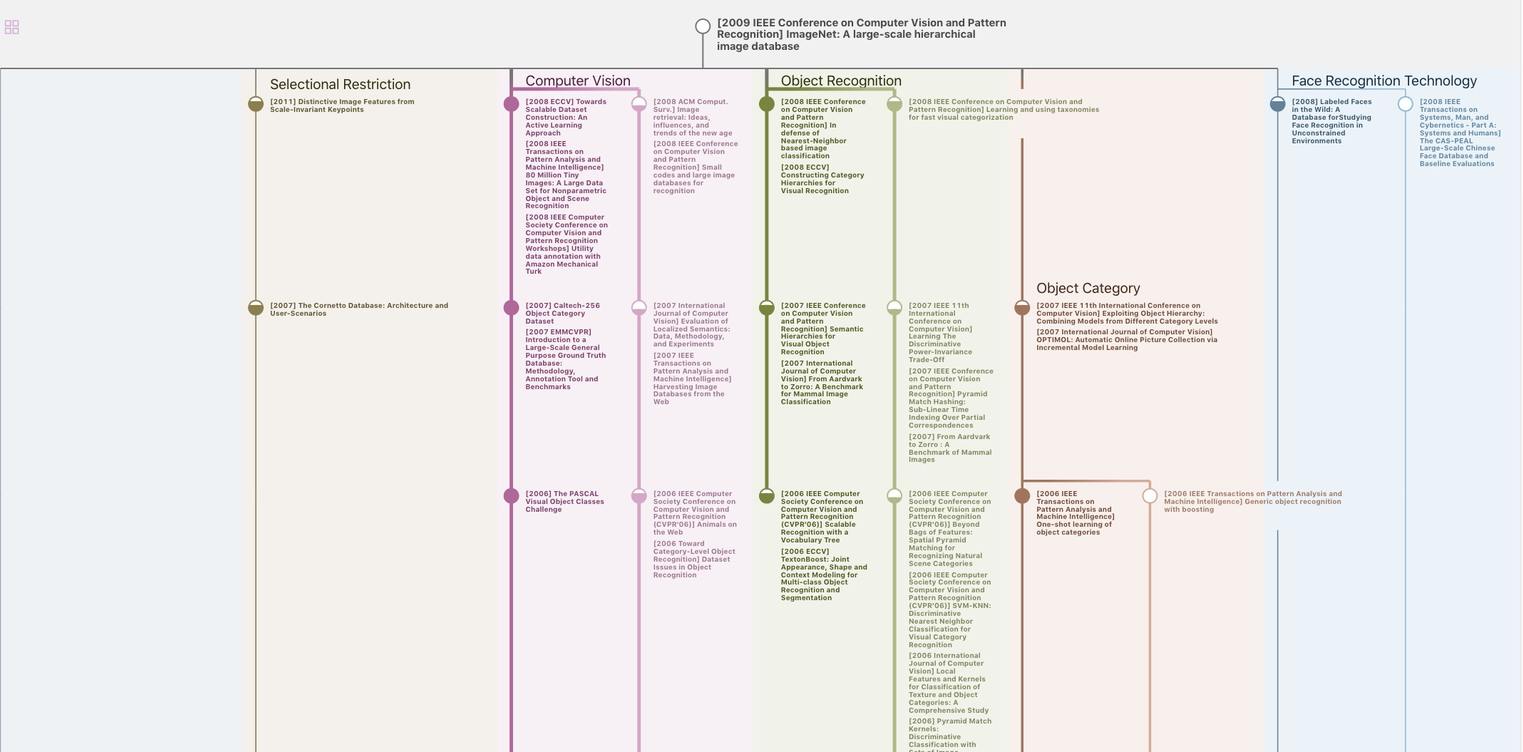
生成溯源树,研究论文发展脉络
Chat Paper
正在生成论文摘要