Military object detection in defense using multi-level capsule networks
SOFT COMPUTING(2021)
摘要
Automatic target detection plays a significant role during war operations. The concept behind automatic target detection in war is military object recognition from the captured images. For object recognition in the given image, convolutional neural network (CNN) architectures are used efficiently. However, CNN suffers from a problem of location invariant and their performance depends mainly on the size of the training set. Generally, the available training data will be in less proportion for military objects due to its operational and security issues. Due to these two issues of CNN, the performance of CNN may degrade abruptly. To address military object recognition, a relatively new neural network architecture based on capsule network (CapsNet) is introduced in this paper. A variant of CapsNet called the multi-level CapsNet framework is projecting in this paper for efficient military object recognition under the case of a small training dataset. The validation of the introduced framework is done on a dataset of military objects collected from the Internet. The dataset contains particularly five different military objects and similar civil ones. Experiments demonstrate that the proposed framework can accomplish a high recognition precision, superior to many other algorithms such as conventional support vector machines and transfer learning-based CNNs.
更多查看译文
关键词
Object recognition,Military objects,Convolution neural network,Capsule networks,Deep learning
AI 理解论文
溯源树
样例
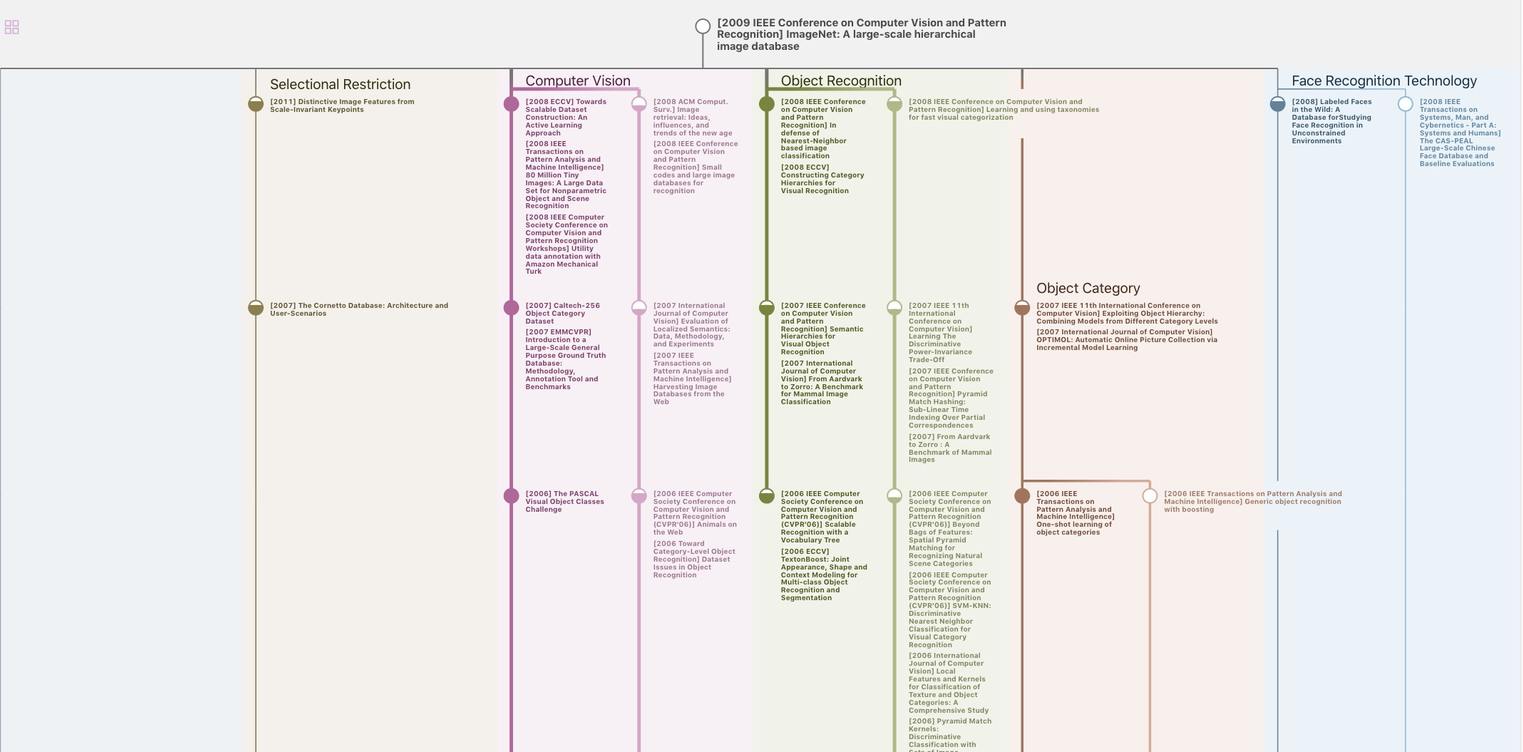
生成溯源树,研究论文发展脉络
Chat Paper
正在生成论文摘要