RF Canceller Tuning Acceleration Using Neural Network Machine Learning for In-Band Full-Duplex Systems
IEEE Open Journal of the Communications Society(2021)
摘要
The fifth-generation wireless system framework provides the option to evaluate the performance of in-band full-duplex (IBFD) operation through flexible duplexing. The resulting self-interference, however, must be mitigated within a fraction of a symbol duration for successful communication. This paper introduces the use of neural network machine learning to accelerate the tuning of multi-tap adaptive RF cancellers. Additionally, the optimal network configurations, input data structures and training dataset densities that optimize the performance of this technique are presented. The tuning results of a prototype system using a two-tap canceller were measured over 20 and 100 MHz bandwidths centered at 2.5 GHz, and demonstrated averages of 40 dB cancellation and 6 tuning iterations. These results are compared to a survey of previously-reported adaptive cancellers, and illustrate that this novel application of machine learning to RF canceller tuning provides the fastest convergence speed to date, which can enable IBFD operation in dynamic interference environments.
更多查看译文
关键词
5G mobile communication,in-band full-duplex,machine learning,RF cancellation,self-interference cancellation
AI 理解论文
溯源树
样例
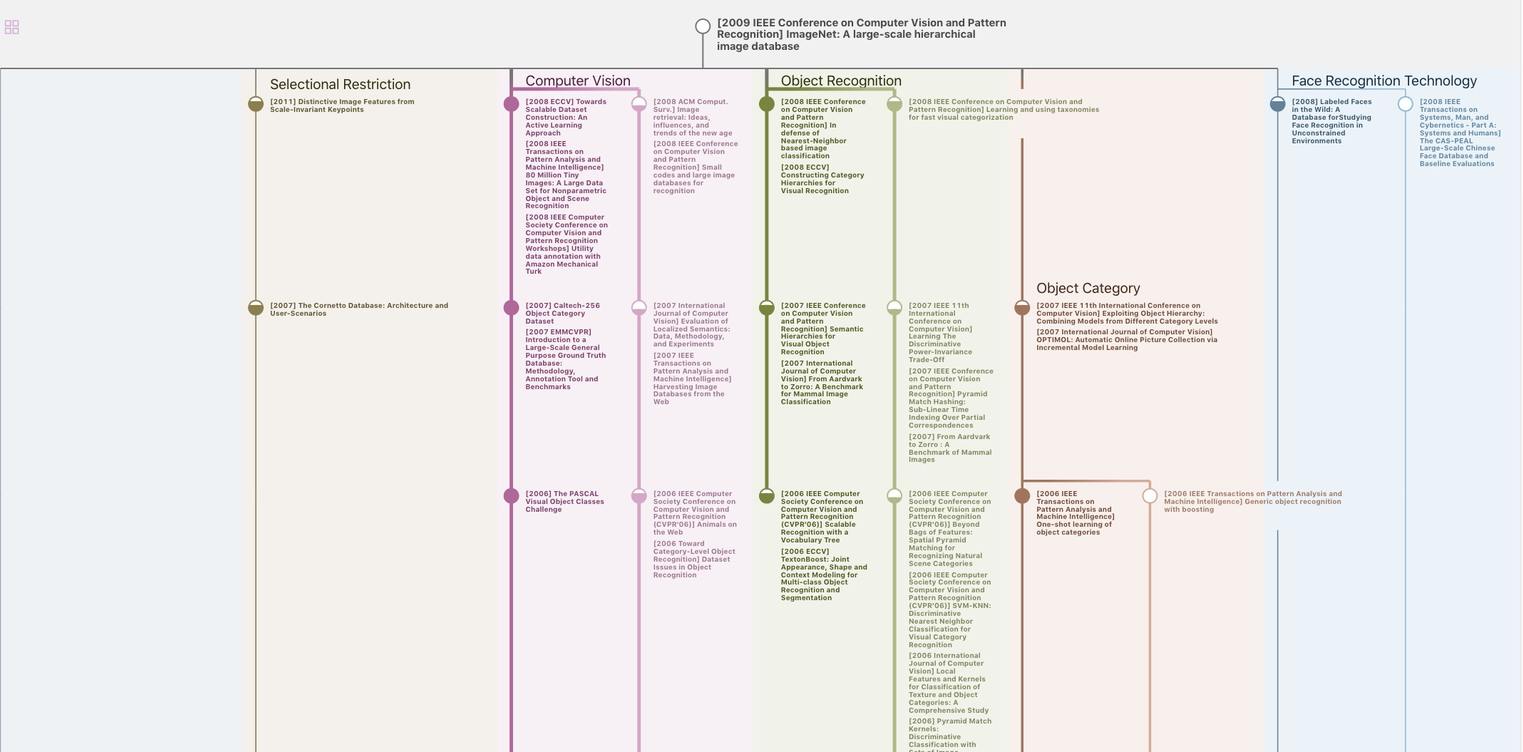
生成溯源树,研究论文发展脉络
Chat Paper
正在生成论文摘要