Code Generation From Natural Language With Less Prior And More Monolingual Data
ACL-IJCNLP 2021: THE 59TH ANNUAL MEETING OF THE ASSOCIATION FOR COMPUTATIONAL LINGUISTICS AND THE 11TH INTERNATIONAL JOINT CONFERENCE ON NATURAL LANGUAGE PROCESSING, VOL 2(2021)
摘要
Training datasets for semantic parsing are typically small due to the higher expertise required for annotation than most other NLP tasks. As a result, models for this application usually need additional prior knowledge to be built into the architecture or algorithm. The increased dependency on human experts hinders automation and raises the development and maintenance costs in practice. This work investigates whether a generic transformer-based seq2seq model can achieve competitive performance with minimal code-generation-specific inductive bias design. By exploiting a relatively sizeable monolingual corpus of the target programming language, which is cheap to mine from the web, we achieved 81.03% exact match accuracy on Django and 32.57 BLEU score on CoNaLa. Both are SOTA to the best of our knowledge. This positive evidence highlights a potentially easier path toward building accurate semantic parsers in practice. (dagger)
更多查看译文
关键词
code generation,monolingual data,natural language
AI 理解论文
溯源树
样例
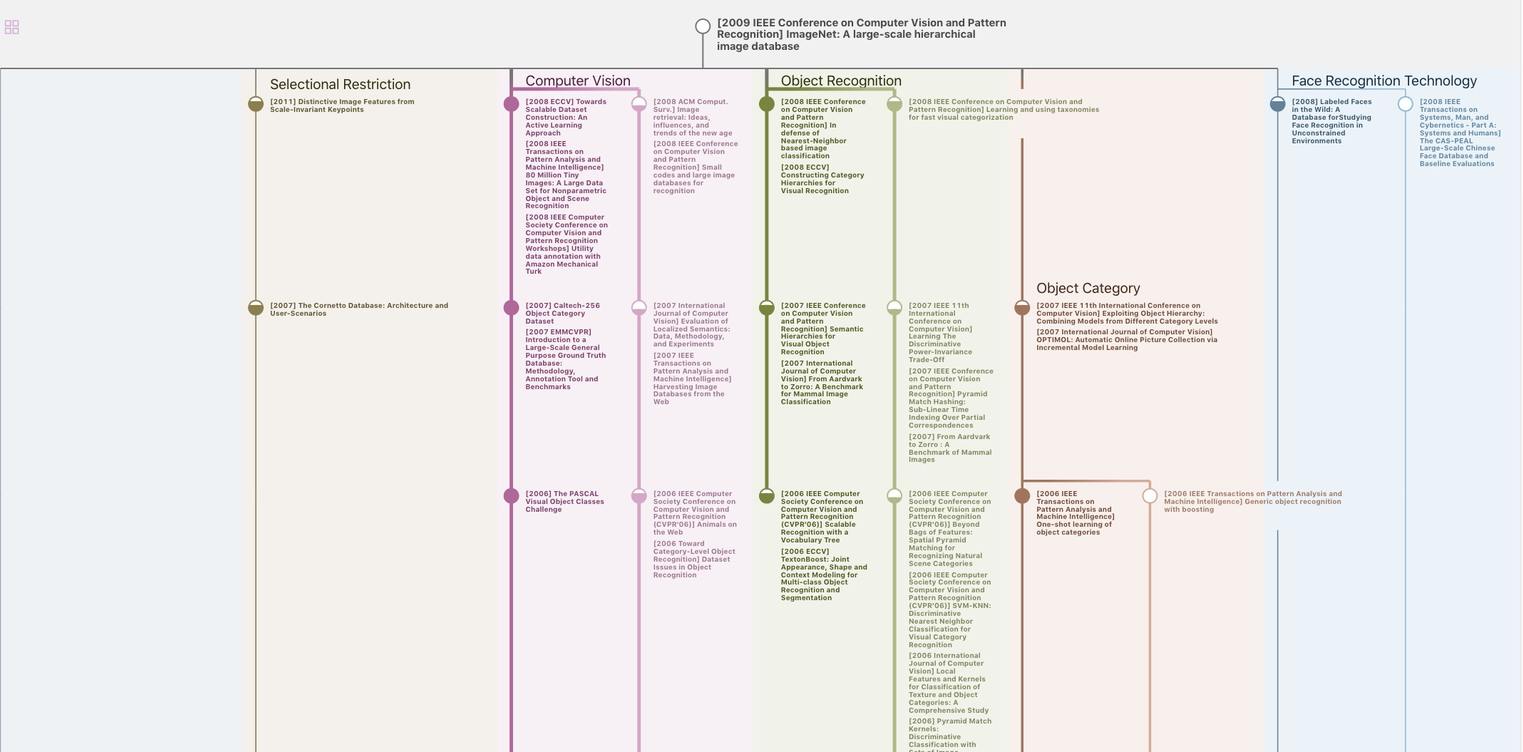
生成溯源树,研究论文发展脉络
Chat Paper
正在生成论文摘要