Research on Action Strategies and Simulations of DRL and MCTS-based Intelligent Round Game
INTERNATIONAL JOURNAL OF CONTROL AUTOMATION AND SYSTEMS(2021)
摘要
The reinforcement learning problem of complex action control in multiplayer online battlefield games has brought considerable interest in the deep learning field. This problem involves more complex states and action spaces than traditional confrontation games, making it difficult to search for any strategy with human-level performance. This paper presents a deep reinforcement learning model to solve this problem from the perspective of game simulations and algorithm implementation. A reverse reinforcement-learning model based on high-level player training data is established to support downstream algorithms. With less training data, the proposed model is converged quicker, and more consistent with the action strategies of high-level players’ decision-making. Then an intelligent deduction algorithm based on DDQN is developed to achieve a better generalization ability under the guidance of a given reward function. At the game simulation level, this paper constructs Monte Carlo Tree Search Intelligent Decision Model for turn-based antagonistic deduction games to generate next-step actions. Furthermore, a prototype game simulator that combines offline with online functions is implemented to verify the performance of proposed model and algorithm. The experiments show that our proposed approach not only has a better reference value to the antagonistic environment using incomplete information, but also accurate and effective in predicting the return value. Moreover, our work provides a theoretical validation platform and testbed for related research on game AI for deductive games.
更多查看译文
关键词
DDQN,deep reinforcement learning,MCTS,round game
AI 理解论文
溯源树
样例
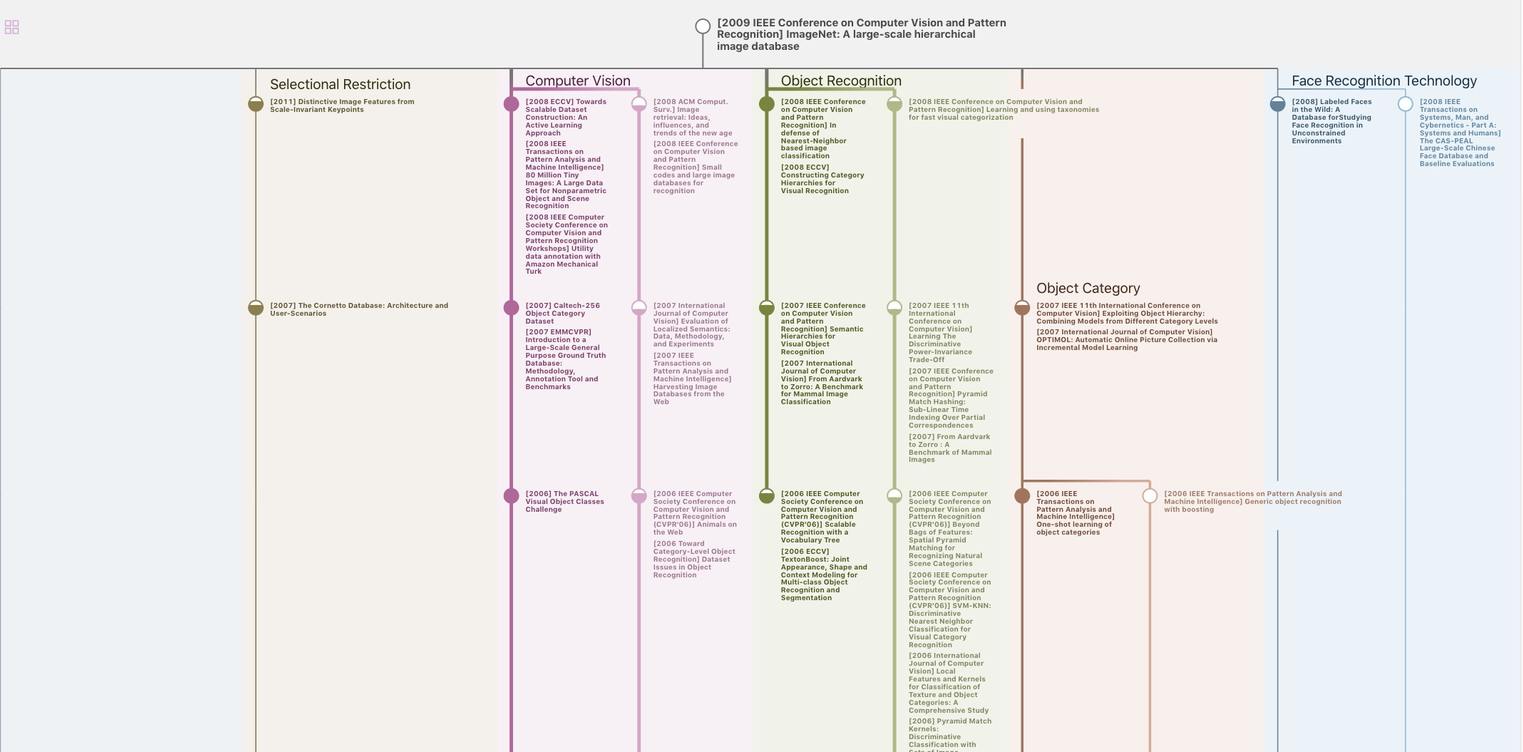
生成溯源树,研究论文发展脉络
Chat Paper
正在生成论文摘要