Deep Convolutional Neural Network Probability Imaging For Plate Structural Health Monitoring Using Guided Waves
IEEE TRANSACTIONS ON INSTRUMENTATION AND MEASUREMENT(2021)
摘要
Guided wave imaging can obtain the damage characterization of imaging features and time difference, and has become a promising tool for structural health monitoring. But most current imaging algorithms still manually select imaging features from first wave or scatter signal. Such manual feature extraction method highly depends on the selection criterion and would significantly reduce the generalization of the monitoring model. In this article, a deep convolutional neural network probability imaging algorithm (DCNN-PIA) is proposed to provide an automatic high-level damage index extraction method for guided wave imaging. Feature selection and multisensor imbalance can be turned away in this semisupervised deep learning method, and the damage will be presented visually. The experiment results illustrate that the proposed method can detect the damage only by using normal state signals, presents a good materials generalization in both aluminum plate and composite plate, and has better performance than other state-of-the-art methods.
更多查看译文
关键词
Convolutional neural network, deep learning, guided wave, probability imaging, structural health monitoring
AI 理解论文
溯源树
样例
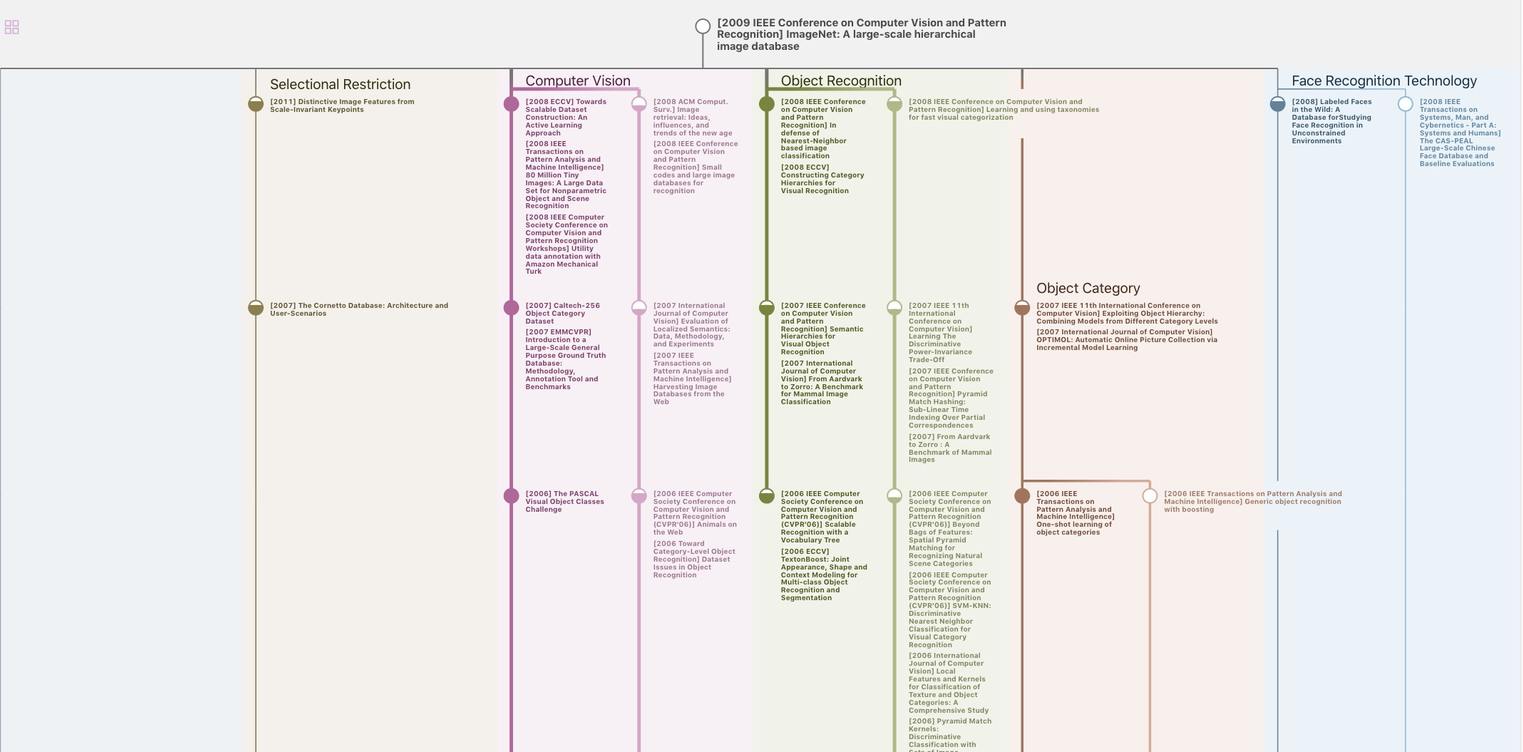
生成溯源树,研究论文发展脉络
Chat Paper
正在生成论文摘要