Machine learning assisted history matching for a deepwater lobe system
Journal of Petroleum Science and Engineering(2021)
摘要
High exploration costs resulting in sparse datasets and complicated geological structures in deepwater depositional systems make the reservoir characterization extremely difficult. To meet this challenge, rule-based geostatistical subsurface modeling has been developed to fill this data gap with enhanced integration of geological concepts for deepwater reservoirs based on geological rules constrained by this sparse well data. The rule-based model simulates sediment dynamics through depositional rules to calculate reservoir architecture and the associated rock properties distributions. As a result, rule-based models incorporate conceptual and qualitative information, such as temporal deposition sequence and consequent compensational stacking patterns. Integrating quantitative data, such as fluid production history, is a remaining obstacle to the broad application of rule-based subsurface models.
更多查看译文
关键词
Rule-based model,Generative adversarial network,Machine learning,Ensemble-based modeling,History matching,Ensemble kalman filter
AI 理解论文
溯源树
样例
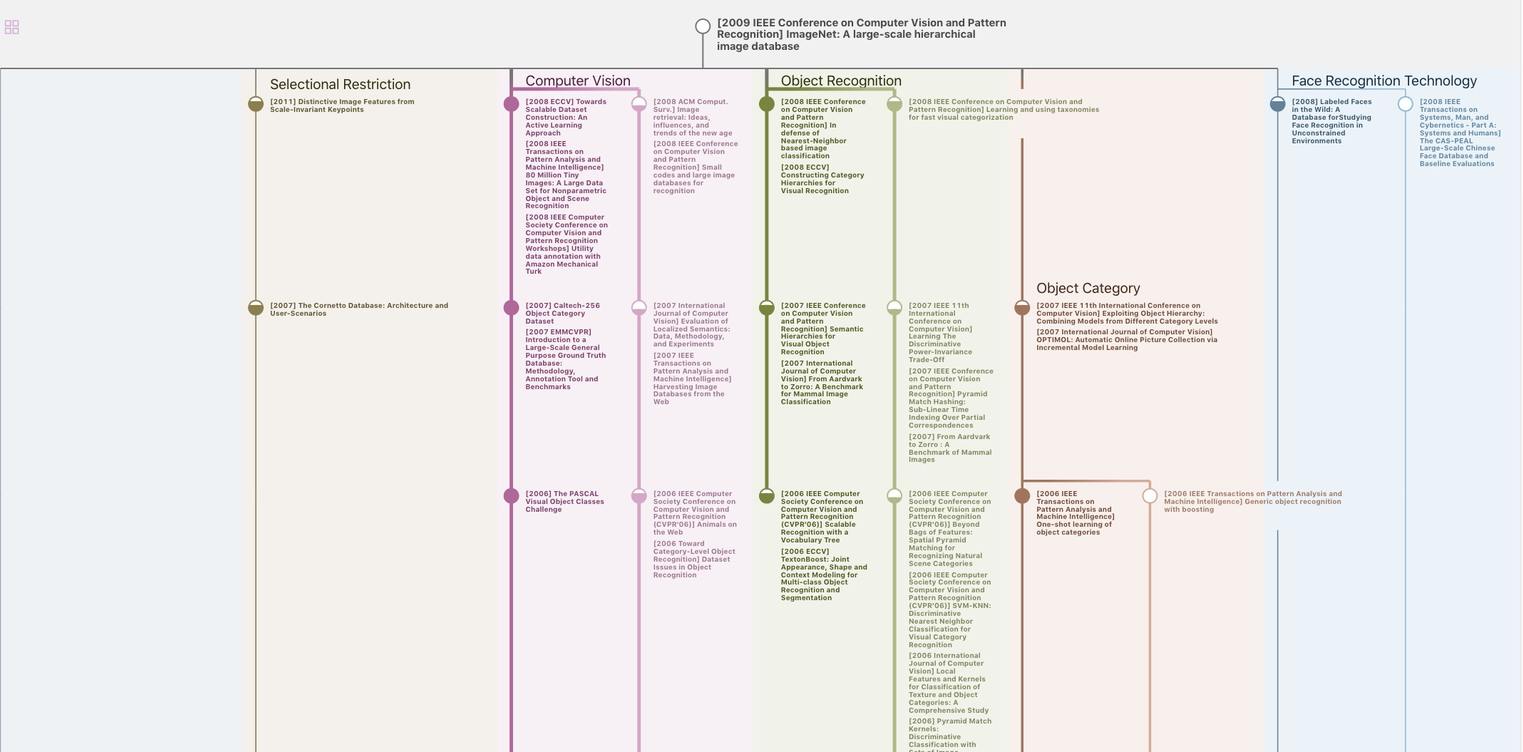
生成溯源树,研究论文发展脉络
Chat Paper
正在生成论文摘要