Particle Filter Algorithm Based on Geometric Center and Likelihood Estimation
AIP advances(2021)
摘要
The improved particle filter (PF) based on the geometric center and likelihood estimation is proposed in order to solve the problem of particle dilution and degradation. In the resampling stage, the geometric center is used to resample the particles. The particles are filtered according to the distance between the particles and the geometric center, and then the particles are resampled. The resampled particles are composed of newborn particles and non-resampled particles. The former can help alleviate the degradation problem, while the latter can keep the diversity of the particle set. In order to ensure effectiveness of the PF, the positioning error threshold of the particle filter is introduced. In the phase of particle weighting calculation, in view of the problem of low accuracy and divergence of PF state estimation caused by non-stationary and non-Gaussian noise, it adopts non-Gaussian noise parameter estimation based on likelihood to approximately estimate the measurement noise instead of the Gaussian density function. The proposed model is applied to particle weight calculation to avoid particle degradation caused by Gaussian density function approximation. The simulation results show that, after the improved algorithm, the root-mean-square error is reduced to 0.085, the variance is reduced to 0.014, and the running time is shortened by 14.8% compared with the polynomial resampling algorithm, which can effectively alleviate particle degradation and dilution in the traditional PF algorithm, and the positioning accuracy is also improved.
更多查看译文
AI 理解论文
溯源树
样例
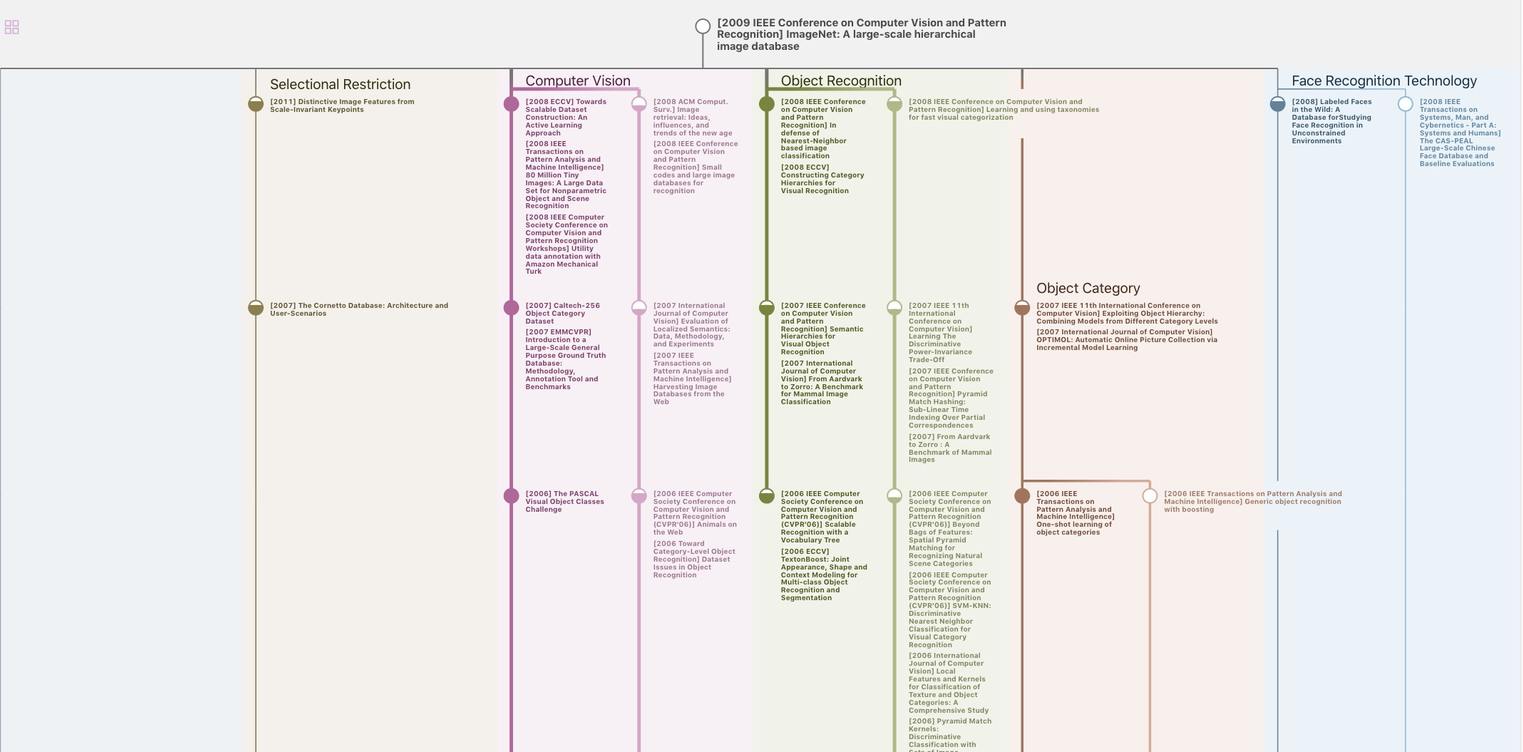
生成溯源树,研究论文发展脉络
Chat Paper
正在生成论文摘要