Satellite Remote Sensing Of Pelagic Sargassum Macroalgae: The Power Of High Resolution And Deep Learning
REMOTE SENSING OF ENVIRONMENT(2021)
摘要
In recent years, massive blooms of pelagic Sargassum have occurred in the Atlantic Ocean, Caribbean Sea, and Gulf of Mexico, and satellite imagery have been used operationally to monitor and track the blooms. However, limited by the coarse resolution and other confounding factors, there is often a data gap in nearshore waters, and the uncertainties in the estimated Sargassum abundance in offshore waters are also unclear. Higher-resolution satellite data may overcome these limitations, yet such a potential is hindered by the lack of reliable methods to accurately detect and quantify Sargassum in an automatic fashion. Here, we address this challenge by combining large quantities of high-resolution satellite data with deep learning. Specifically, data from the Multispectral Instrument (MSI, 10-20 m), Operational Land Imager (OLI, 30 m), WorldView-II (WV-2, 2 m), and PlanetScope/Dove (3 m) are used with a deep convolution neural network (DCNN) to extract Sargassum features and quantify Sargassum biomass density or areal coverage. By utilizing the U-net architecture and the pre-trained weights from the VGG16 model, the DCNN (i.e., the VGGUnet model) can extract Sargassum features while discarding other confusing features (waves, currents, phytoplankton blooms, clouds, cloud shadows, or striping noise). For Sargassum biomass estimated from OLI and MSI images, results indicate an accuracy of -92% and 90%, respectively, when evaluated using images from the same sensor. When Sargassum areal coverage is estimated from WV-2 and Dove images, there is an accuracy of -98% and 82%, respectively. When different sensors are cross-compared, OLI reveals -30% more Sargassum biomass than MODIS from 14 OLI images collected in the Caribbean Sea (path/row: 001/050) for their commonly viewed observable areas, and - 180% more Sargassum biomass than MSI (N = 15, path/row: 001/050); such differences appear systematic (R2 = 0.98 and 0.73, respectively). Compared to the quasi-simultaneous MSI, OLI, and MODIS images, Dove shows higher Sargassum coverage. Higher-resolution sensors tend to observe more Sargassum because they can detect smaller-scale features that are missed by the coarser-resolution sensors, although the difference varies with time and location. The morphological characteristics of Sargassum features from these high-resolution data are also reported to facilitate management actions. The findings here not only fill the knowledge gaps and coverage gaps from previous studies, but more importantly pave the road toward operational monitoring and tracking Sargassum features in nearshore waters.
更多查看译文
关键词
Landsat-8, Sentinel-2, MSI, OLI, Worldview-2, Dove, MODIS, FAI, Sargassum, Clouds, Feature extraction, Deep convolution neural network, Deep learning, U-net, VGGUnet
AI 理解论文
溯源树
样例
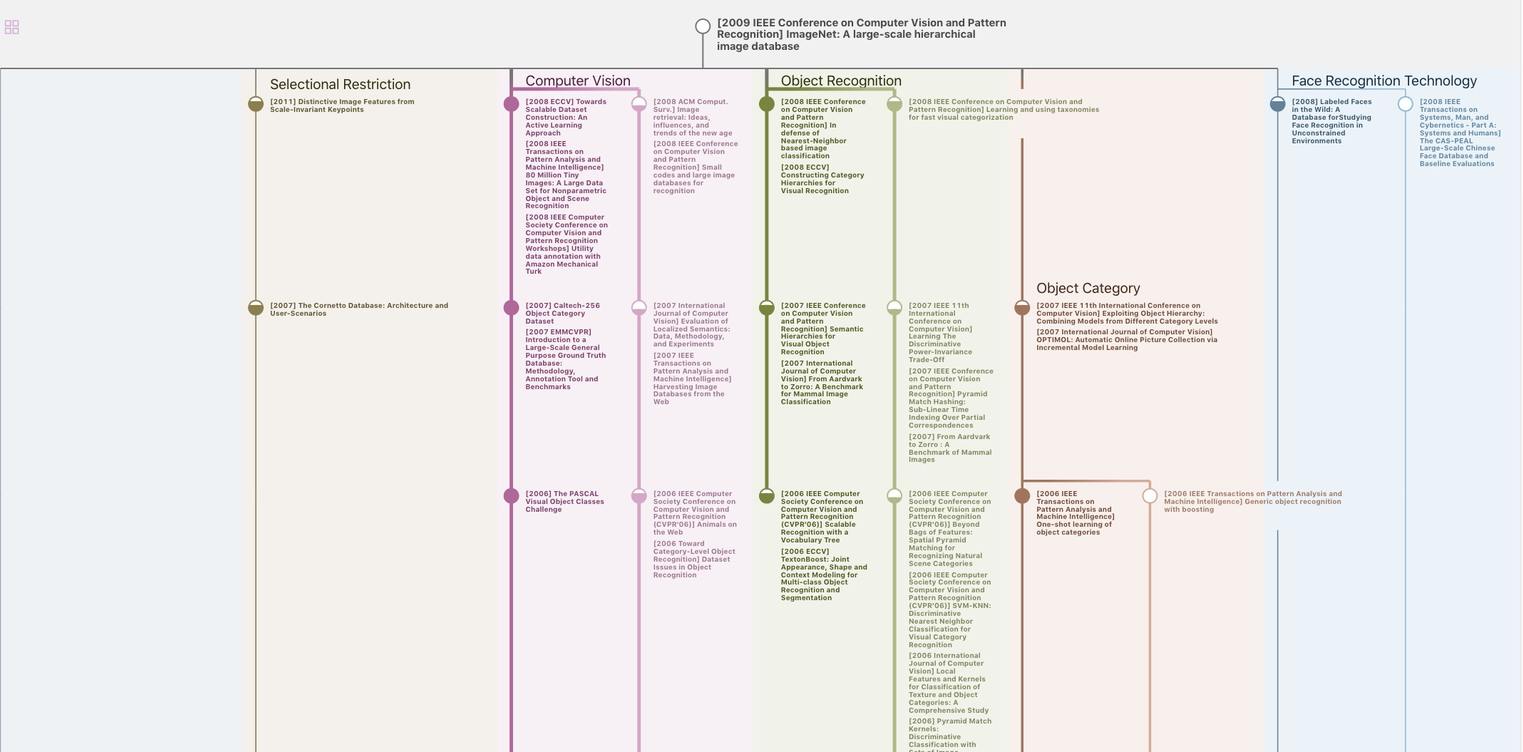
生成溯源树,研究论文发展脉络
Chat Paper
正在生成论文摘要