Hlstm: Heterogeneous Long Short-Term Memory Network For Large-Scale Insar Ground Subsidence Prediction
IEEE JOURNAL OF SELECTED TOPICS IN APPLIED EARTH OBSERVATIONS AND REMOTE SENSING(2021)
摘要
Accurate prediction of ground subsidence is of great significance for the prevention and mitigation of this type of geological disaster. It is still a challenge when wide area is concerned. In this article, a heterogeneous long short-term memory (HLSTM) network is proposed for large-scale ground subsidence prediction based on interferometric synthetic aperture radar (InSAR) data. First, the study area is divided into homogeneous subregions through spatial clustering of InSAR-derived subsidence velocity. Second, a specific LSTM model is constructed to capture complex nonlinear temporal correlations embedded in InSAR-derived subsidence time series for each subregion. Essentially both spatial heterogeneity and temporal correlation are incorporated into the HLSTM prediction. In the experiment part, the HLSTM predictor is validated using a subsidence monitoring result from 80 Sentinel-1 images acquired over Cangzhou, China, from 2017 to 2019. The HLSTM result shows the highest prediction accuracy through comparisons with the results from other seven methods.
更多查看译文
关键词
Predictive models, Training, Strain, Data models, Time series analysis, Indexes, Geology, Deep learning, heterogeneity, interferometric synthetic aperture radar (InSAR), long short-term memory (LSTM), subsidence prediction
AI 理解论文
溯源树
样例
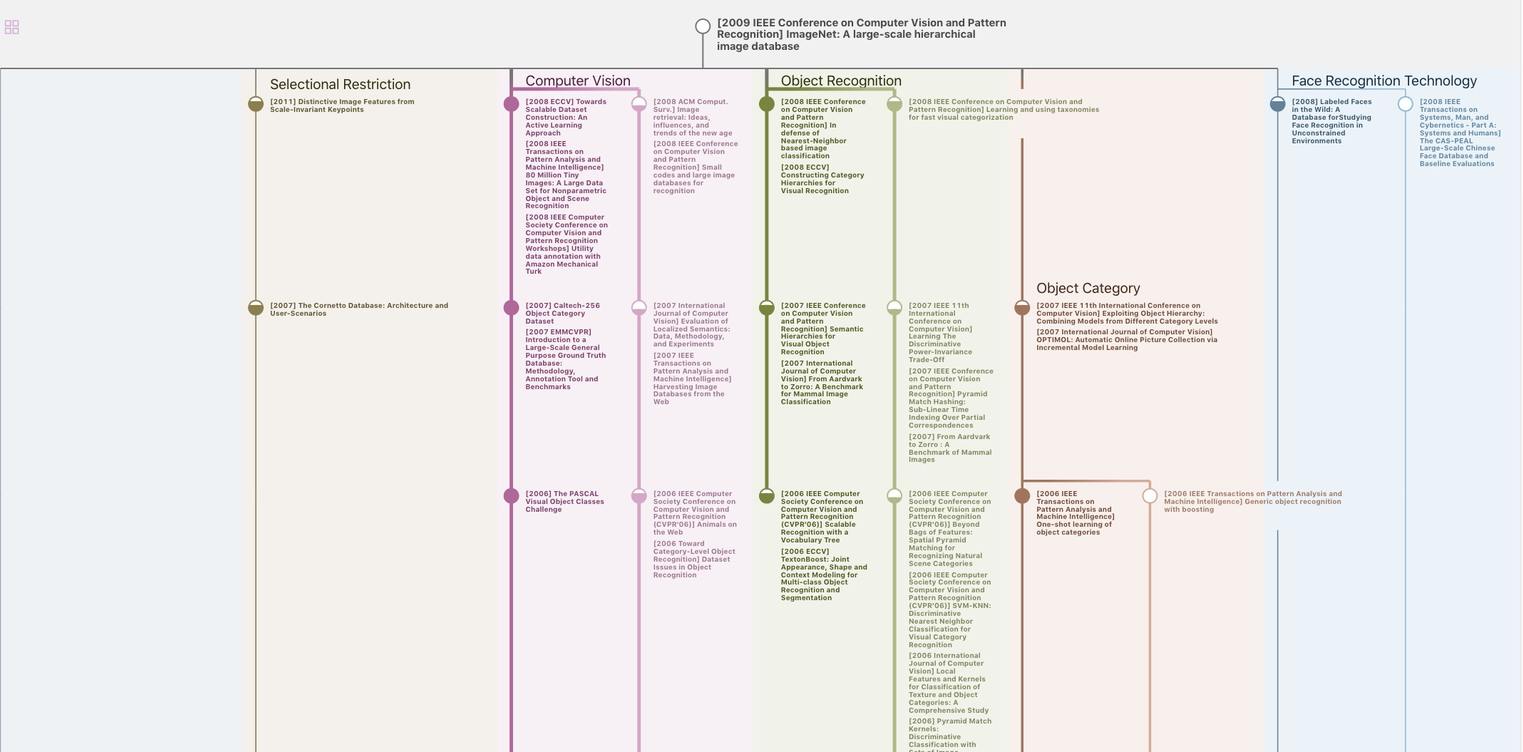
生成溯源树,研究论文发展脉络
Chat Paper
正在生成论文摘要