Dan-Conv: Depth Aware Non-Local Convolution For Lidar Depth Completion
ELECTRONICS LETTERS(2021)
摘要
Sparse LiDAR depth completion is a beneficial task for many robotic applications. It commonly generates a dense depth prediction from a sparse depth map and its corresponding aligned RGB image. This image-guided depth completion task mainly has two challenges: sparse data processing and multi-modality data fusion. In this letter, they are dealt with by two novel solutions: (1) To efficiently process sparse depth input, a Depth Aware Non-local Convolution (DAN-Conv) is proposed. It augments the spatial sampling locations of a convolution operation. Specifically, DAN-Conv constructs a non-local neighbourhood relationship by exploiting the intrinsic correlation among observable depth pixels. In particular, it can readily replace standard convolution without introducing additional network parameters. (2) A Symmetric Co-attention Module (SCM) is proposed to fuse and enhance features from depth and image domain. It estimates the importance of complementary features by the co-attention mechanism. Finally, a neural network built on DAN-Conv and SCM is proposed. It achieves competitive performance on the challenging KITTI depth completion benchmark. Comparing to approaches with approximate accuracy, this lightweight network requires significantly fewer learnable parameters.
更多查看译文
关键词
Optical, image and video signal processing,Optical radar,Computer vision and image processing techniques,Data handling techniques
AI 理解论文
溯源树
样例
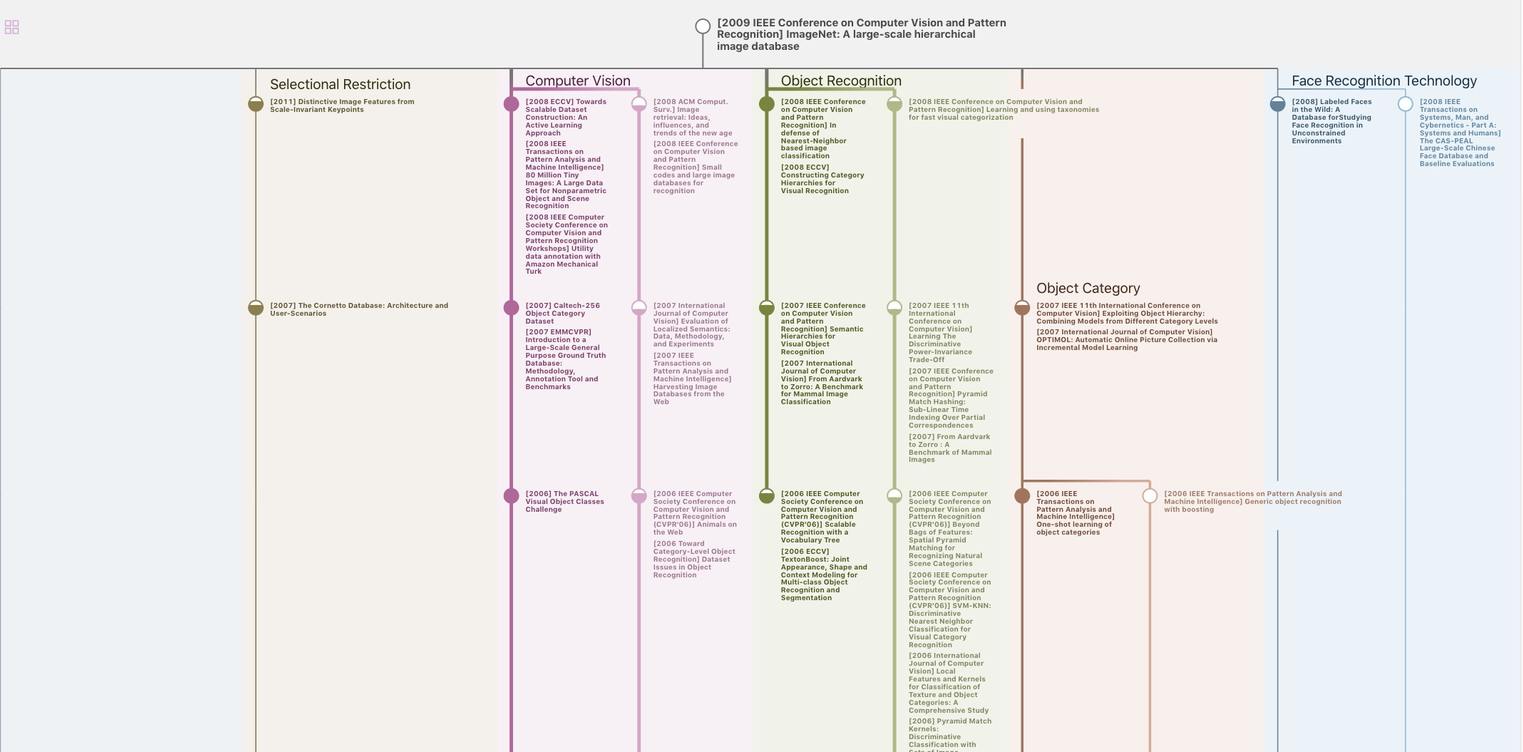
生成溯源树,研究论文发展脉络
Chat Paper
正在生成论文摘要