Comparison of habitat models in quantifying the spatio-temporal distribution of small yellow croaker (Larimichthys polyactis) in Haizhou Bay, China
Estuarine, Coastal and Shelf Science(2021)
摘要
Habitat models can be used to analyze the relationships between species distribution and environmental variables. Different models and response variables are usually selected to evaluate the habitat of species according to their ecological traits. In this study, six habitat models were compared to quantify the spatio-temporal distribution of small yellow croaker (Larimichthys polyactis) in Haizhou Bay, including Generalized Additive Model (GAM), K-Nearest Neighbor (KNN), Multivariate Adaptive Regression Splines (MARS), Generalized Boosted regression Model (GBM), Random forest (RF) and Artificial neural network (NN). We also compared the performance of habitat models with four types of response variables, i.e., (1) presence-absence, (2) habitat ranks, (3) probability and (4) abundance. Model performances were evaluated and assessed through cross validations. Principal component analysis (PCA) and coefficient of variation (CV) were applied to compare the performance of these habitat models. Results showed that machine learning algorithms were better than statistical methods. Random forest (RF) performed the best in identifying the spatio-temporal distribution of small yellow croakers based on PCA and CV analysis. The importance of explanatory variables varied with the type of response variables in habitat modeling. In addition, facing different response variables, species showed different response relationships to explanatory variables. This species was found mainly distributed in the central coastal areas of Haizhou Bay at depths between 10 and 30 m according to predicated distribution maps by optimal models. Bottom temperature, bottom salinity and prey had great impacts on spatial distributions of small yellow croaker. This study proposed a framework to evaluate the performance of different habitat models and response variables, which could be extended to other marine organisms and facilitate the application of habitat models. This study enhances our understanding of the underlying ecological mechanisms that drive spatial distribution of marine species.
更多查看译文
关键词
Habitat models,Machine learning,Classification,Regression,Spatio-temporal distribution
AI 理解论文
溯源树
样例
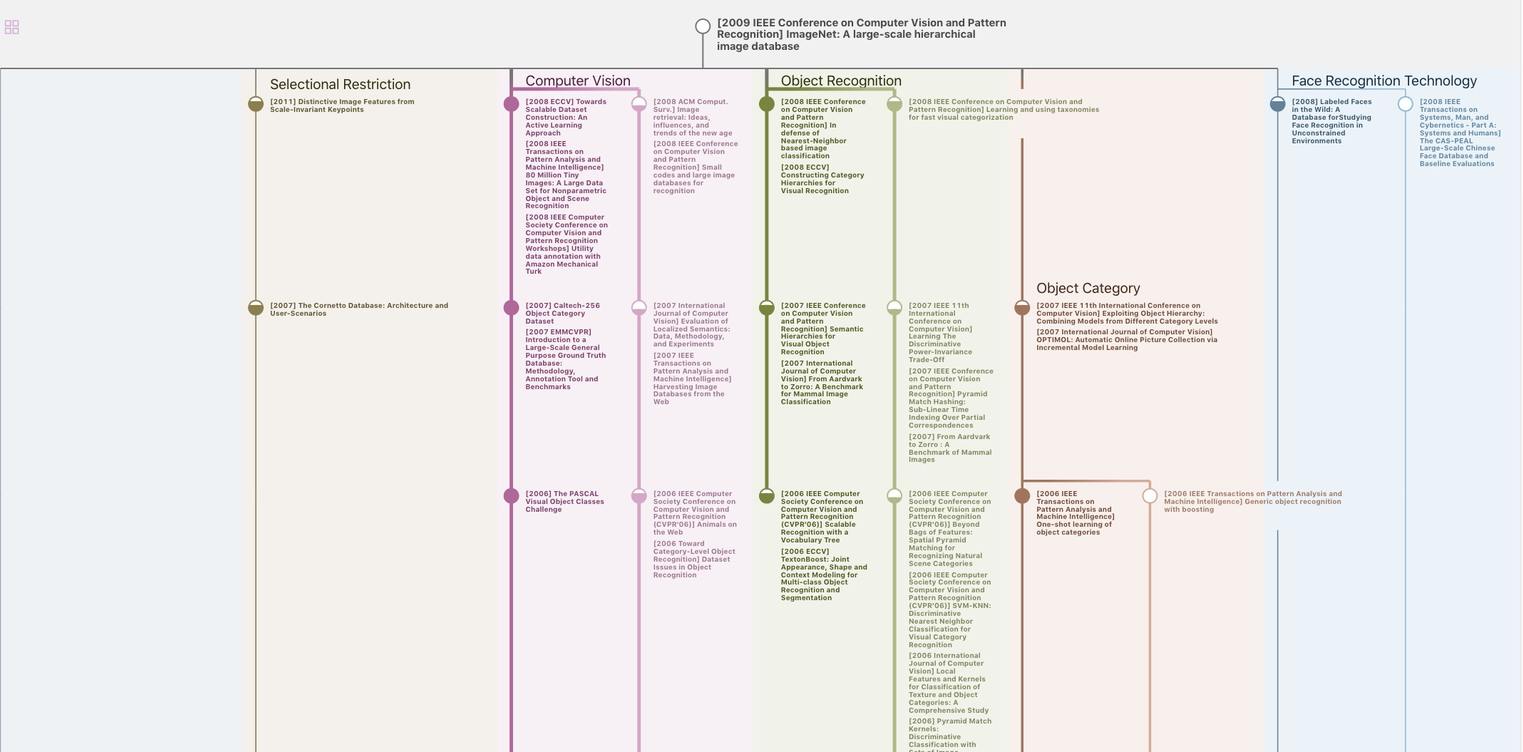
生成溯源树,研究论文发展脉络
Chat Paper
正在生成论文摘要