Mining Process Data To Detect Aberrant Test Takers
MEASUREMENT-INTERDISCIPLINARY RESEARCH AND PERSPECTIVES(2021)
摘要
Item harvesters who memorize, record and share test items can jeopardize the validity and fairness of credentialing tests. Item harvesting behaviors are difficult to detect by the existing statistical modeling approaches due to the absence of operational definitions and the idiosyncratic nature of human behaviors. Motivated to detect the hard-to-define aberrant test-taking behaviors like item harvesting, we proposed a data-mining approach that utilized the process data and identified the examinees whose test-taking processes deviate from the majority of examinees. Specifically, two steps were implemented in the proposed approach: First, archetypes of test-taking processes are learned with the k-means clustering algorithm; second, examinees whose behavioral patterns deviate from the archetypes are flagged for further investigation. Given that the process data makes it possible to capture more subtle differences between the aberrant test-takers and normal examinees, the proposed approach is expected to be complementary of the statistical modeling methods, capture additional types of aberrant test-takers and increase the probability of discovering the elusive item harvesters.
更多查看译文
关键词
Process data, cheating detection, abnormal test-taking behavior, clustering, data mining
AI 理解论文
溯源树
样例
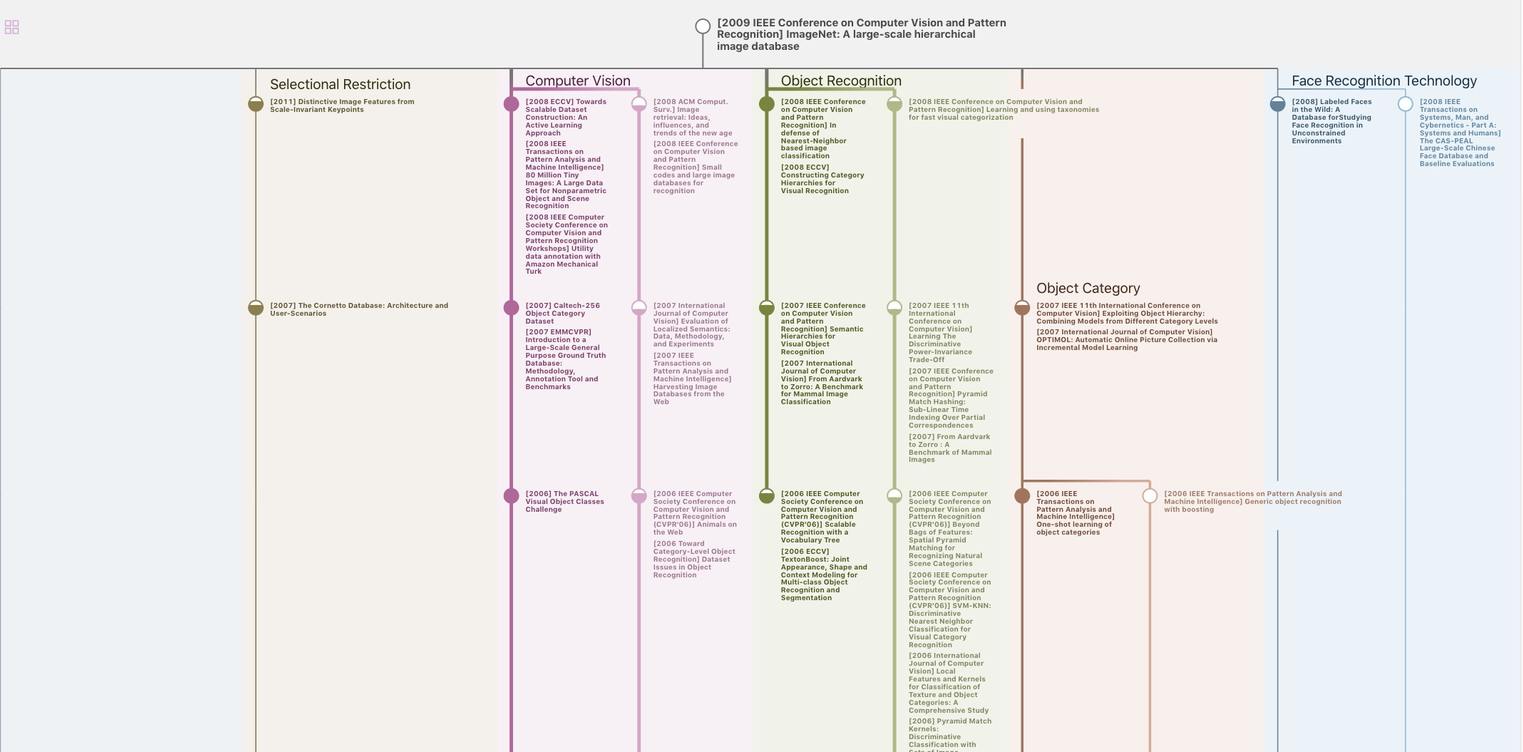
生成溯源树,研究论文发展脉络
Chat Paper
正在生成论文摘要