Sparsifying Regularizations For Stochastic Sample Average Minimization In Ultrasound Computed Tomography
MEDICAL IMAGING 2021: ULTRASONIC IMAGING AND TOMOGRAPHY(2021)
摘要
Ultrasound computed tomography (USCT) is a promising imaging modality for breast cancer screening. Three challenges commonly arising in time-of-flight USCT are (1) to choose a physical forward model that describes acoustic wave propagation in an inhomogeneous medium appropriately (2) to effectively mitigate the ill-posedness for an adequate reconstruction of the model and (3) to efficiently deal with large data sets. In this contribution, we investigate methods that address these three challenges by developing an optimization strategy based on a stochastic descent method that adaptively subsamples the data and analyze its performance in combination with different sparsity-enforcing regularization techniques.
更多查看译文
关键词
Ultrasound computed tomography, sparsity, l(1)-minimization, curvelets, stochastic optimization
AI 理解论文
溯源树
样例
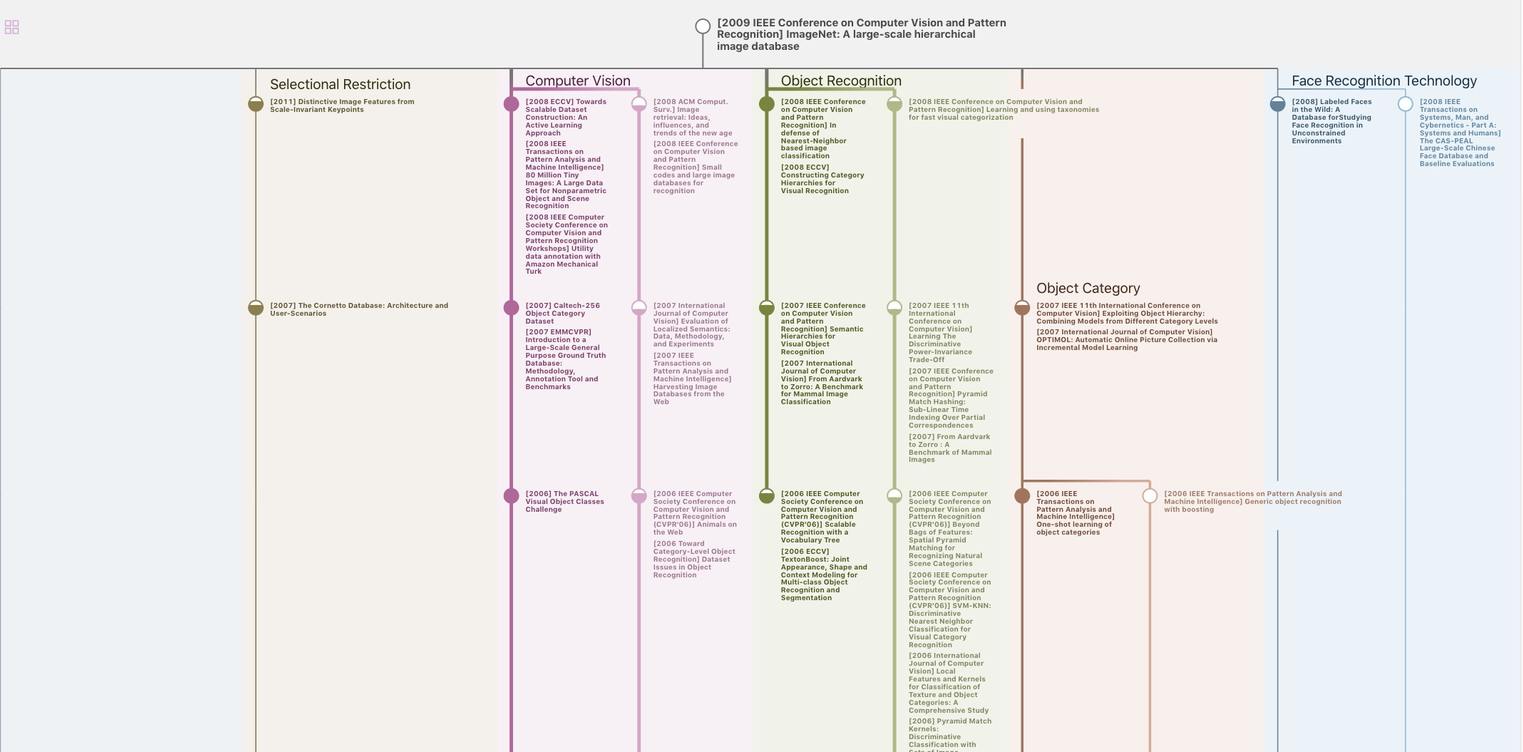
生成溯源树,研究论文发展脉络
Chat Paper
正在生成论文摘要