Locality Preserving And Label-Aware Constraint-Based Hybrid Dictionary Learning For Image Classification
APPLIED SCIENCES-BASEL(2021)
摘要
Dictionary learning has been an important role in the success of data representation. As a complete view of data representation, hybrid dictionary learning (HDL) is still in its infant stage. In previous HDL approaches, the scheme of how to learn an effective hybrid dictionary for image classification has not been well addressed. In this paper, we proposed a locality preserving and label-aware constraint-based hybrid dictionary learning (LPLC-HDL) method, and apply it in image classification effectively. More specifically, the locality information of the data is preserved by using a graph Laplacian matrix based on the shared dictionary for learning the commonality representation, and a label-aware constraint with group regularization is imposed on the coding coefficients corresponding to the class-specific dictionary for learning the particularity representation. Moreover, all the introduced constraints in the proposed LPLC-HDL method are based on the l2-norm regularization, which can be solved efficiently via employing an alternative optimization strategy. The extensive experiments on the benchmark image datasets demonstrate that our method is an improvement over previous competing methods on both the hand-crafted and deep features.
更多查看译文
关键词
locality preserving, label-aware constraint, hybrid dictionary learning, image classification
AI 理解论文
溯源树
样例
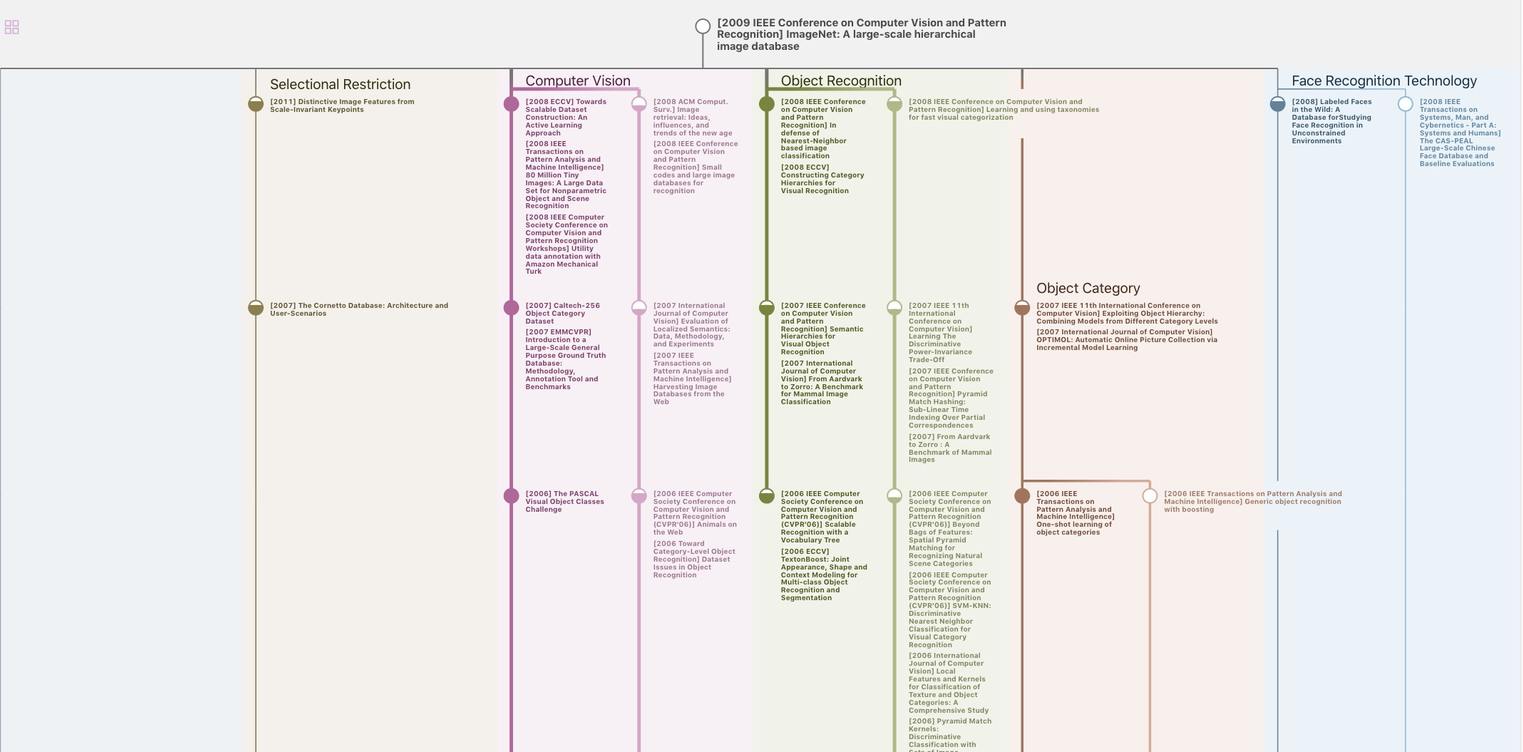
生成溯源树,研究论文发展脉络
Chat Paper
正在生成论文摘要