Content Matters: Clustering Web Pages for QoE Analysis With WebCLUST
IEEE ACCESS(2021)
摘要
The properties of a web page have a strong impact on its overall loading process, including the download of its contents and their progressive rendering at the browser. As a consequence, web page content has a strong impact on the experience of web users. In this paper, we present WebCLUST, a clustering-based classification approach for web pages, which groups pages into quality-meaningful content classes impacting the Quality of Experience (QoE) of the users. Groups are defined based on standard Multipurpose Internet Mail Extensions (MIME) content breakdown and external subdomain connections, obtained through in-browser, application level measurements. Using a large corpus of multi-device, heterogeneous web content and QoE-relevant measurements for the top-500 most popular websites in the Internet, we show how WebCLUST can automatically identify relevant web content classes showing significantly different performance in terms of Web QoE relevant metrics, such as Speed Index. We additionally evaluate the impact of content caching and device type on the identification performance of WebCLUST, showing how content classes might look significantly different, depending on the access device type (desktop vs mobile), as well as when considering browser caching. Our findings suggest that Web QoE assessment should explicitly consider page content and subdomain embedding within the analysis, especially when it comes to recent work on Web QoE inference through machine learning models. To the best of our knowledge, this is the first study showing the impact of web content on Web QoE metrics, opening the door to new Web QoE assessment strategies.
更多查看译文
关键词
Web pages, Quality of experience, Browsers, Measurement, Tools, Rendering (computer graphics), Performance evaluation, Web, Quality of Experience, clustering, WebPageTest, service performance
AI 理解论文
溯源树
样例
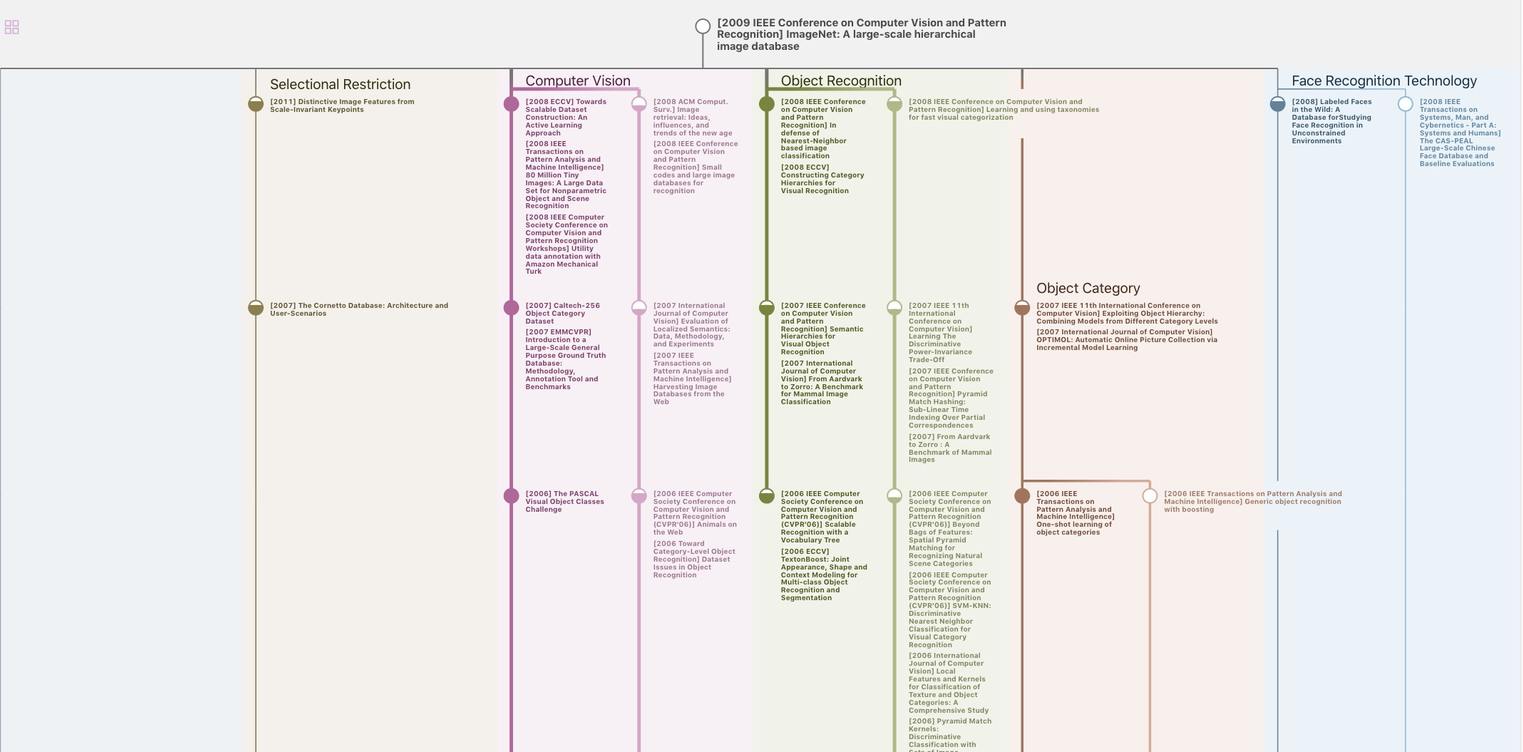
生成溯源树,研究论文发展脉络
Chat Paper
正在生成论文摘要