Recognition of Early Blight and Late Blight Diseases on Potato Leaves Based on Graph Cut Segmentation
Journal of agriculture and food research(2021)
摘要
Late blight and early blight are the most destructive diseases for potatoes. It is valuable to distinguish diseases and their degrees of infection on potato leaves for timely prevention. This study investigated an accurate recognition method for detecting the disease type and degree of infection from potato leaf images. To segment the leaf from the images efficiently and accurately, an automatic scheme for the graph-cut algorithm is developed. The seeds of the foreground were extracted by Otsu thresholding, and the seeds of the background were extracted by color statistical thresholding on a* and b* components. To remove the backgrounds that have similar color as the infected patch, the superpixels that neighbor the outline of the leaf will be iteratively eliminated when their entropies are far from those of the major part of the leaf. Then, the color features were extracted from the individual channels of the L*a*b* on the refined region of interest (ROI), and the texture features were extracted using a local binary pattern (LBP). Finally, four classifiers based on the k-nearest neighbor (k-NN), support vector machine (SVM), artificial neural network (ANN) and random forest (RF) methods were adopted to evaluate the performance for recognition of potato disease. The performance of the proposed method was evaluated on 2840 images of healthy and diseased potato leaves. The segmentation results showed that the average intersection over union (IoU) was 93.70% for the five classes. For disease classification, the SVM classifier achieved the highest overall accuracy of 97.4% compared with k-NN, ANN and RF. For the degree of infection classification, and the SVM classifier achieved the highest overall accuracy of 91.0%. To enhance the classification performance, a combination of six types of features was evaluated. The results showed that SVM achieved the highest overall accuracy of 92.1% with the combinations of a local binary pattern (LBP) on the a* component, LBP on the b* component, the color histogram on the L* component, and the color histogram on the a* component.
更多查看译文
关键词
Potato disease,Disease recognition,Early blight,Late blight,Image segmentation,Graph-cut algorithm
AI 理解论文
溯源树
样例
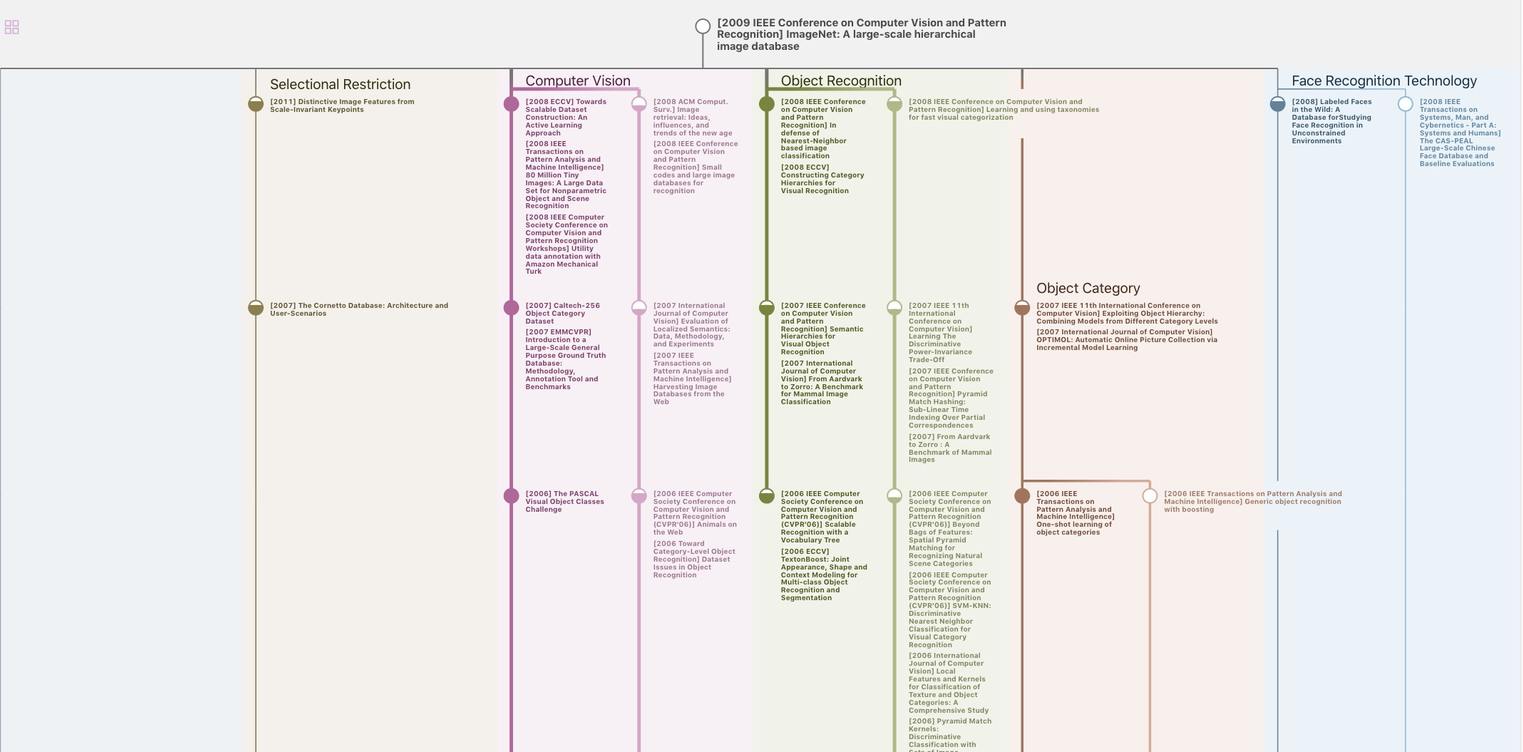
生成溯源树,研究论文发展脉络
Chat Paper
正在生成论文摘要