Accented Speech Recognition Based On End-To-End Domain Adversarial Training Of Neural Networks
APPLIED SCIENCES-BASEL(2021)
摘要
Featured Application This research can be applied for automatic speech recognition systems that handle input speech with two or more accents. The performance of automatic speech recognition (ASR) may be degraded when accented speech is recognized because the speech has some linguistic differences from standard speech. Conventional accented speech recognition studies have utilized the accent embedding method, in which the accent embedding features are directly fed into the ASR network. Although the method improves the performance of accented speech recognition, it has some restrictions, such as increasing the computational costs. This study proposes an efficient method of training the ASR model for accented speech in a domain adversarial way based on the Domain Adversarial Neural Network (DANN). The DANN plays a role as a domain adaptation in which the training data and test data have different distributions. Thus, our approach is expected to construct a reliable ASR model for accented speech by reducing the distribution differences between accented speech and standard speech. DANN has three sub-networks: the feature extractor, the domain classifier, and the label predictor. To adjust the DANN for accented speech recognition, we constructed these three sub-networks independently, considering the characteristics of accented speech. In particular, we used an end-to-end framework based on Connectionist Temporal Classification (CTC) to develop the label predictor, a very important module that directly affects ASR results. To verify the efficiency of the proposed approach, we conducted several experiments of accented speech recognition for four English accents including Australian, Canadian, British (England), and Indian accents. The experimental results showed that the proposed DANN-based model outperformed the baseline model for all accents, indicating that the end-to-end domain adversarial training effectively reduced the distribution differences between accented speech and standard speech.
更多查看译文
关键词
accented speech recognition, speech recognition, end-to-end domain adversarial training, domain adversarial neural network, domain adaptation, connectionist temporal classification
AI 理解论文
溯源树
样例
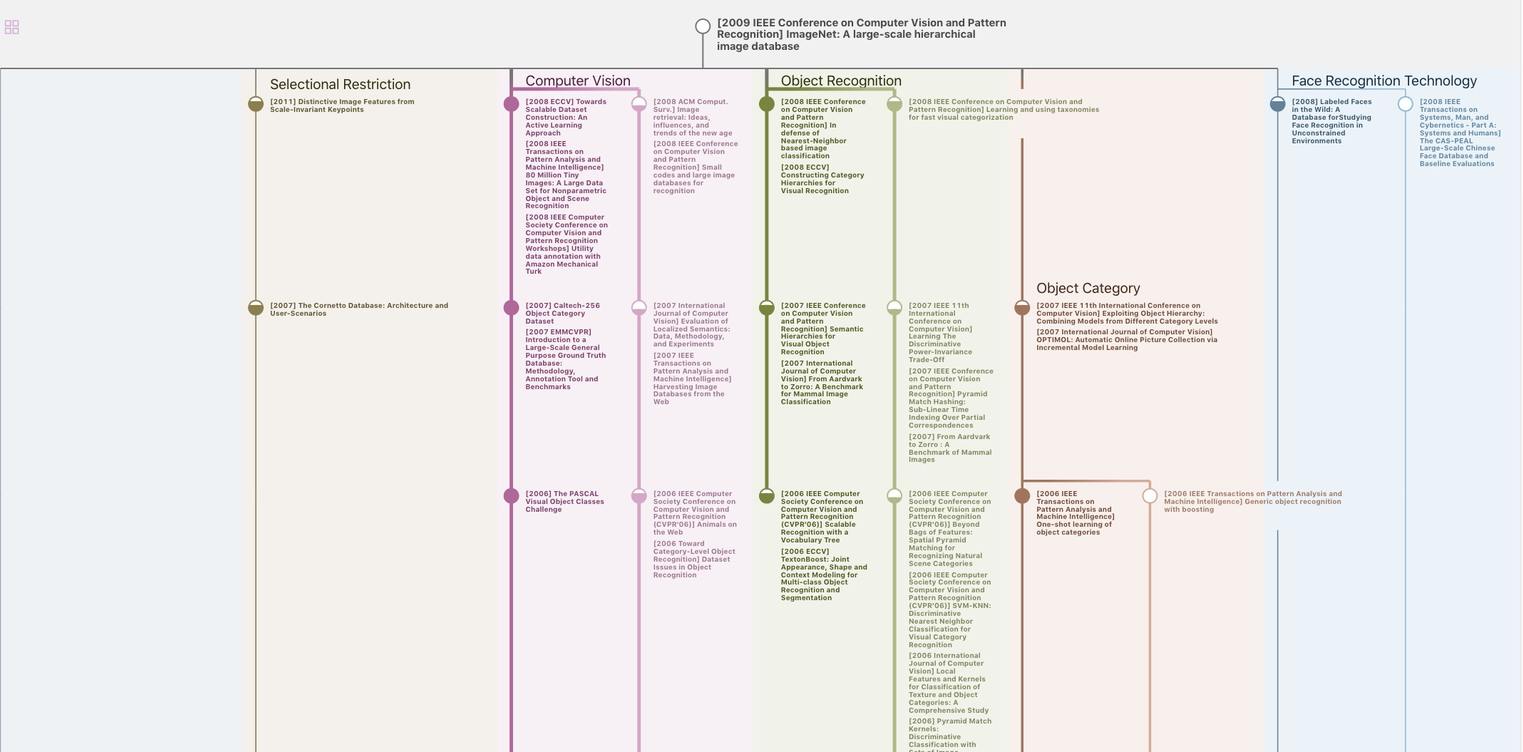
生成溯源树,研究论文发展脉络
Chat Paper
正在生成论文摘要