Cryptographic Based Secure Model on Dataset for Deep Learning Algorithms
Computers, materials & continua/Computers, materials & continua (Print)(2021)
摘要
Deep learning (DL) algorithms have been widely used in various security applications to enhance the performances of decision-based models. Malicious data added by an attacker can cause several security and privacy problems in the operation of DL models. The two most common active attacks are poisoning and evasion attacks, which can cause various problems, includ-ing wrong prediction and misclassification of decision-based models. There-fore, to design an efficient DL model, it is crucial to mitigate these attacks. In this regard, this study proposes a secure neural network (NN) model that provides data security during model training and testing phases. The main idea is to use cryptographic functions, such as hash function (SHA512) and homomorphic encryption (HE) scheme, to provide authenticity, integrity, and confidentiality of data. The performance of the proposed model is evaluated by experiments based on accuracy, precision, attack detection rate (ADR), and computational cost. The results show that the proposed model has achieved an accuracy of 98%, a precision of 0.97, and an ADR of 98%, even for a large number of attacks. Hence, the proposed model can be used to detect attacks and mitigate the attacker motives. The results also show that the computational cost of the proposed model does not increase with model complexity.
更多查看译文
关键词
Deep learning (DL),poisoning attacks,evasion attacks,neural network,hash functions SHA512,homomorphic encryption scheme
AI 理解论文
溯源树
样例
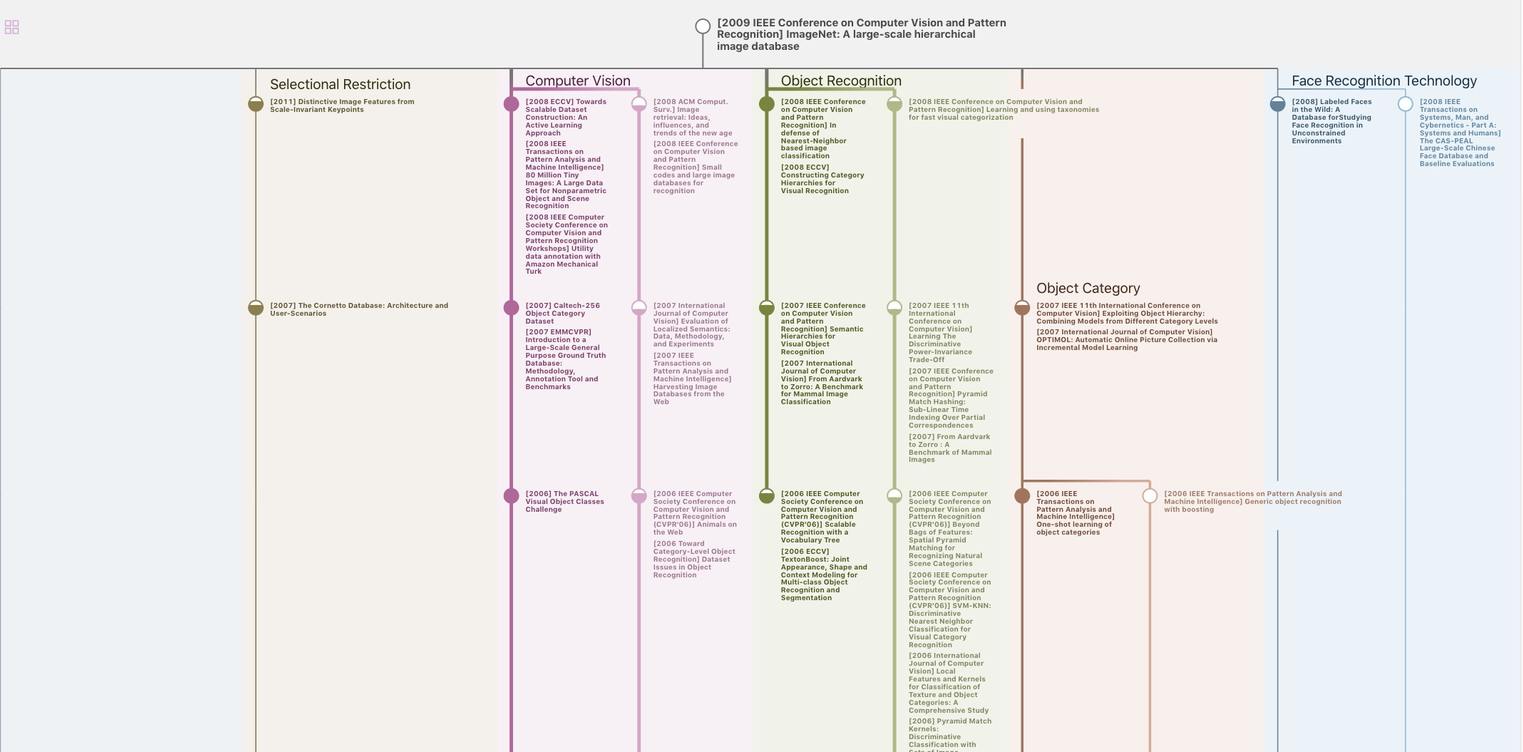
生成溯源树,研究论文发展脉络
Chat Paper
正在生成论文摘要