Rapid Data Annotation For Sand-Like Granular Instance Segmentation Using Mask-Rcnn
AUTOMATION IN CONSTRUCTION(2022)
摘要
Image processing, as an efficient and accurate technology, has been widely applied to characterize granular object morphology in many fields, such as construction engineering, material science, agriculture, etc. Tradi-tional static image processing is not autonomous because it cannot automatically segment contacting particles. In contrast, the current deep-learning-based algorithms can achieve high degree of autonomy in instance seg-mentation given it is well trained. However, lack of training data is a common pain point as it requires extensive manual labour using the conventional labelling tools. In this study, using sand as an example, we proposed a mask labelling methodology that can establish a large and diverse training set without manual labelling. The trained Mask-RCNN demonstrates excellent performance on a densely packed particle image. Using the data labelling method proposed in this study and the deep-learning algorithms, fully automated image processing can be realized for granular materials without massive manual labelling workload.
更多查看译文
关键词
Granular matter, Morphology characterization, Overlapping particle segmentation, Zero manual labelling, Mask-RCNN
AI 理解论文
溯源树
样例
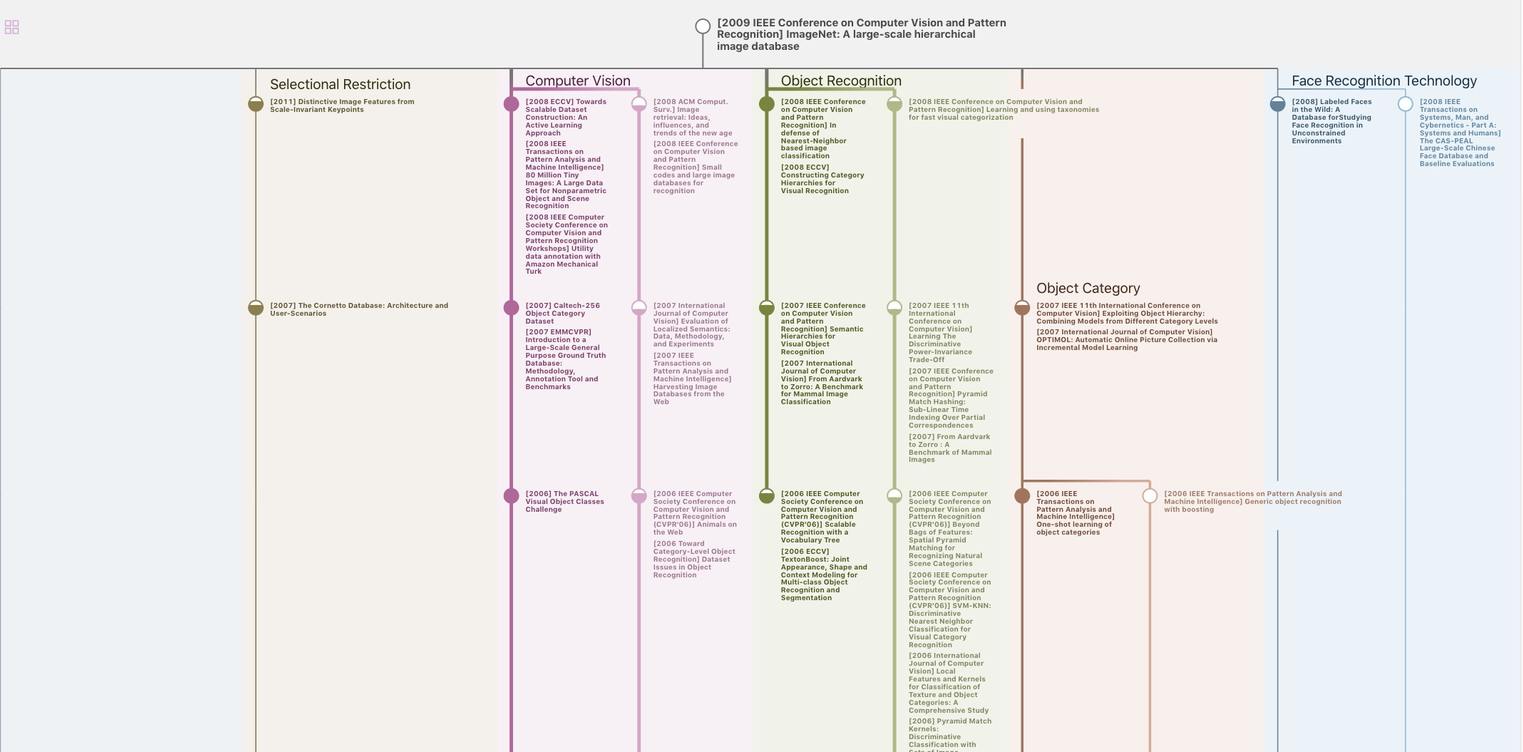
生成溯源树,研究论文发展脉络
Chat Paper
正在生成论文摘要