Credit scoring by one-class classification driven dynamical ensemble learning
JOURNAL OF THE OPERATIONAL RESEARCH SOCIETY(2022)
摘要
It is very useful to endow machines with the ability to measure credit scores of loan applicants. Conventional methodologies always train Credit Scoring (CS) models by using data from clients who passed previous credit examination (i.e. who were considered adequately creditworthy and took out a loan). However, the CS models trained on data from the applicants who with good credit background may not work well for new applicants with plain or ambiguous credit backgrounds. Previous work always alleviates this by techniques of rejected inference and semisupervised learning. In this article, we propose a novel approach called as "One-class Classification Driven Dynamical Ensemble Learning" (abbreviated as OCDDEL). Different from rejected inference or semisupervised learning, OCDDEL does not use inferred labels of past rejected applications. Instead, OCDDEL only relies on past accepted applications and their true labels. It builds a dynamical ensemble model which deal with different test applications in different ways. To determine the ensemble weights for a specific test case, OCDDEL will learn a one-class classifier to separate test applications into groups, according to their similarities with training applicants. An experimental evaluation with 2 real-world datasets demonstrates the effectiveness of our approach.
更多查看译文
关键词
Credit scoring, machine learning, ensemble learning
AI 理解论文
溯源树
样例
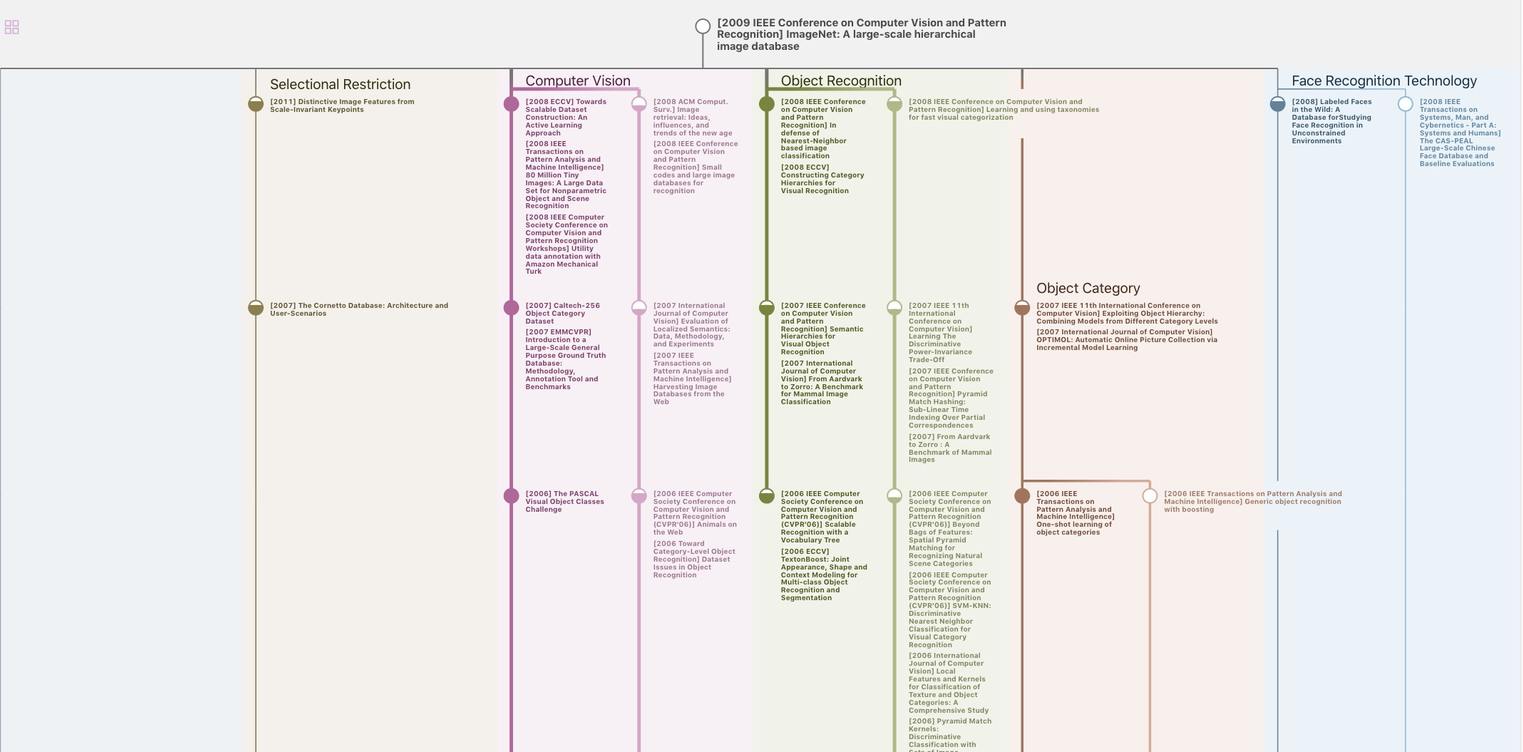
生成溯源树,研究论文发展脉络
Chat Paper
正在生成论文摘要