Hierarchical point process models for recurring safety critical events involving commercial truck drivers: A reliability framework for human performance modeling
JOURNAL OF QUALITY TECHNOLOGY(2022)
摘要
Quality in the trucking industry involves several facets, including on-time performance and safety. In the largest naturalistic driving study to-date, with 496 drivers and 13 M miles driven, we address two safety questions: (a) does the occurrence of safety critical events increase during a driving shift? and (b) what is the effect of rest breaks on the incidence of those events? To address these two questions, we adopt point process models, commonly used to assess the reliability of repairable systems, to model the occurrence/likelihood of safety critical events. To account for driver differences, driver-level random effects were also assumed. Our results show that: (a) the intensity for hard brakes decreases throughout a shift, (b) rest breaks reduce the likelihood of activation of the automated collision mitigation system, and (c) there is a fair amount of variability among drivers. Given that a hard brake (a less severe safety critical event) is more common in the beginning of the shift, it can potentially be explained by an increased likelihood of being in a local/city road and/or increased likelihood of aggressive driving behavior early in a driver's shift. Furthermore, we quantified the impact of rest breaks in reducing engagement of the more severe automated collision mitigation system, providing data-driven evidence on the importance of rest-break scheduling for trucking safety. Properties of the approach were also investigated through a simulation study, where we examined the consequences of an incorrect specification of the Bayesian priors.
更多查看译文
关键词
Bayesian hierarchical models, reliability, safety-critical events, trucking
AI 理解论文
溯源树
样例
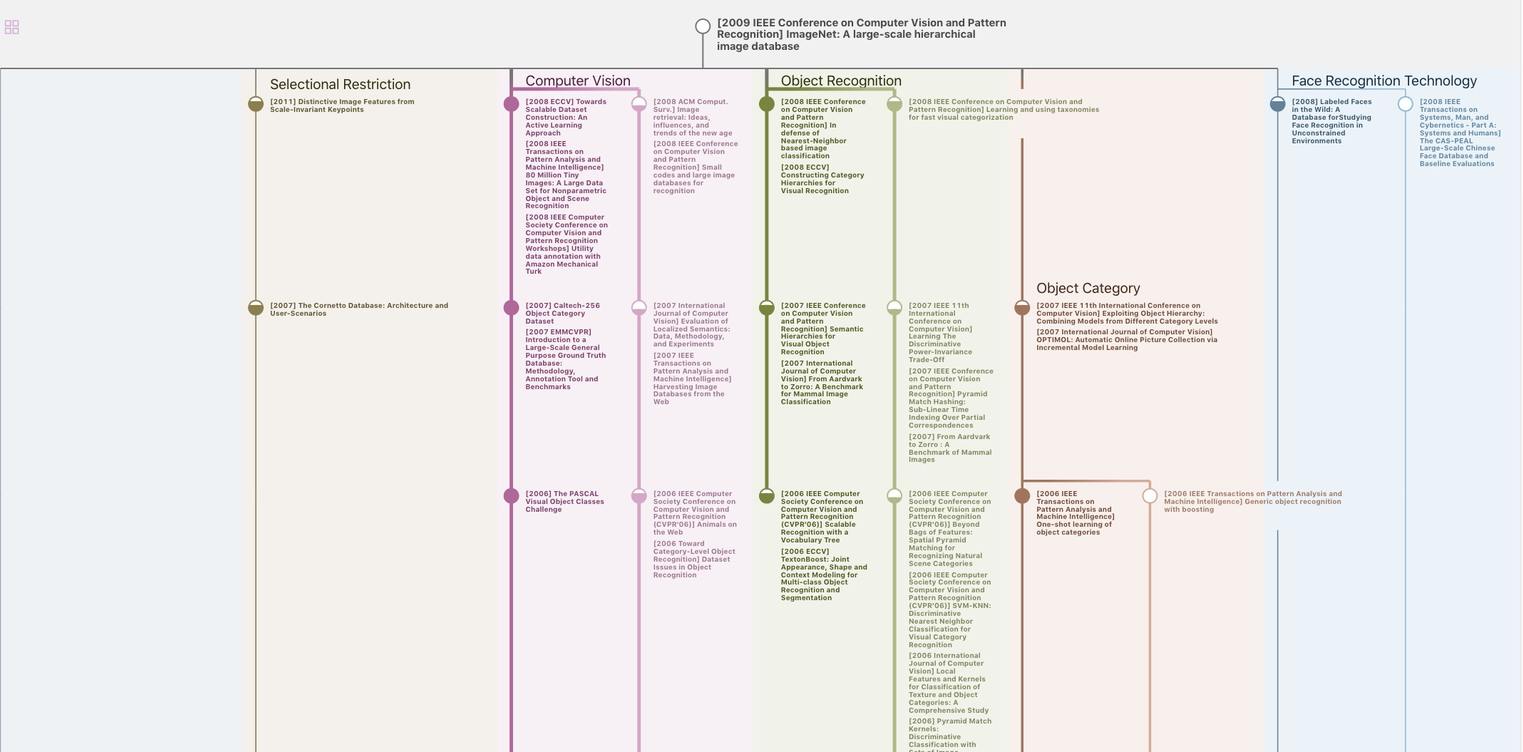
生成溯源树,研究论文发展脉络
Chat Paper
正在生成论文摘要