An Intelligent Forecasting Model for Disease Prediction Using Stack Ensembling Approach
Computers, materials & continua/Computers, materials & continua (Print)(2022)
摘要
This research work proposes a new stack-based generalization ensemble model to forecast the number of incidences of conjunctivitis disease. In addition to forecasting the occurrences of conjunctivitis incidences, the proposed model also improves performance by using the ensemble model. Weekly rate of acute Conjunctivitis per 1000 for Hong Kong is collected for the duration of the first week of January 2010 to the last week of December 2019. Pre-processing techniques such as imputation of missing values and logarithmic transformation are applied to pre-process the data sets. A stacked generalization ensemble model based on Auto-ARIMA (Autoregressive Inte-grated Moving Average), NNAR (Neural Network Autoregression), ETS (Exponential Smoothing), HW (Holt Winter) is proposed and applied on the dataset. Predictive analysis is conducted on the collected dataset of con-junctivitis disease, and further compared for different performance measures. The result shows that the RMSE (Root Mean Square Error), MAE (Mean Absolute Error), MAPE (Mean Absolute Percentage Error), ACF1 (Auto Correlation Function) of the proposed ensemble is decreased significantly. Considering the RMSE, for instance, error values are reduced by 39.23%, 9.13%, 20.42%, and 17.13% in comparison to Auto-ARIMA, NAR, ETS, and HW model respectively. This research concludes that the accuracy of the forecasting of diseases can be significantly increased by applying the proposed stack generalization ensemble model as it minimizes the prediction error and hence provides better prediction trends as compared to Auto-ARIMA, NAR, ETS, and HW model applied discretely.
更多查看译文
关键词
Disease prediction,stack ensemble,neural network autoregression,exponential smoothing,auto-ARIMA,holt winter
AI 理解论文
溯源树
样例
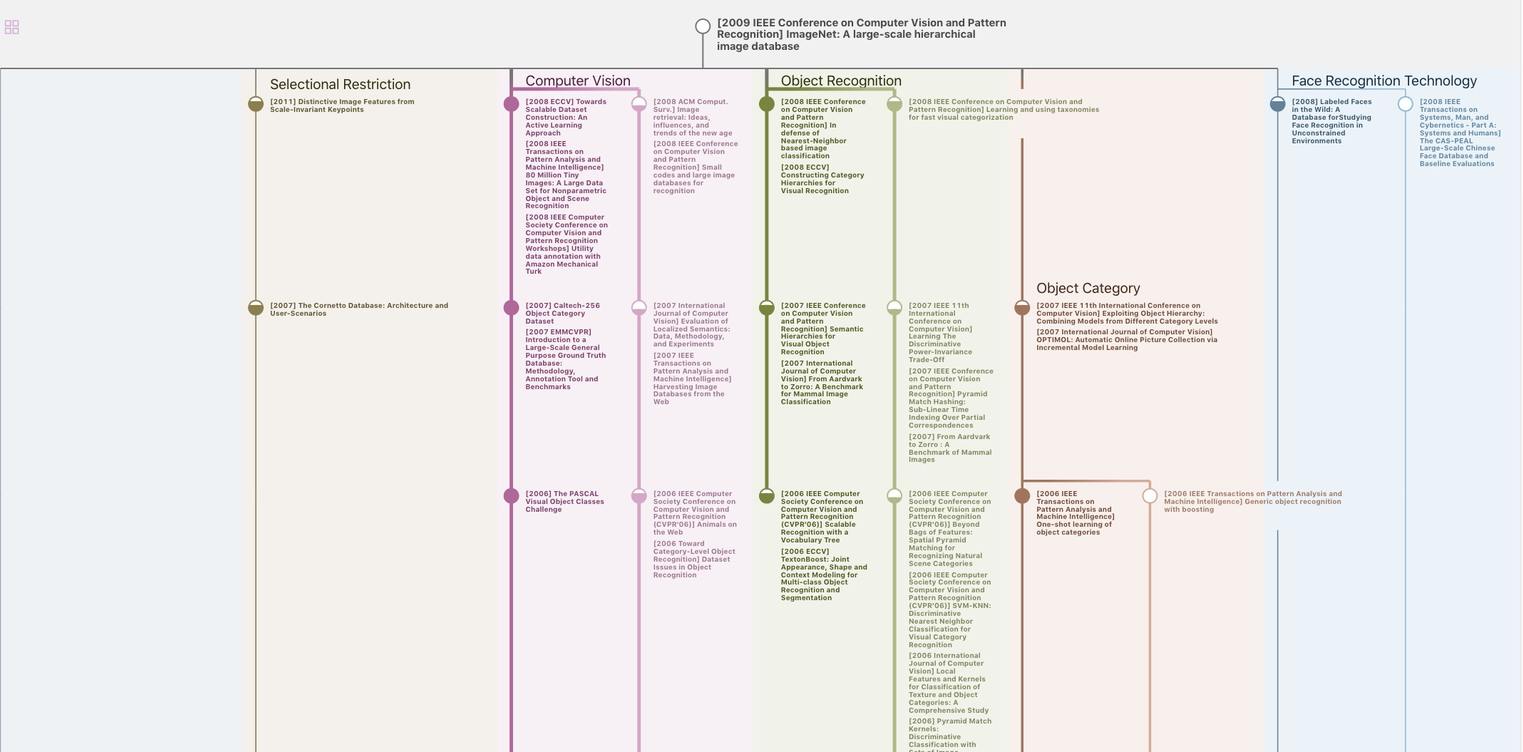
生成溯源树,研究论文发展脉络
Chat Paper
正在生成论文摘要