Optimization Of Cognitive Radio System Using Self-Learning Salp Swarm Algorithm
CMC-COMPUTERS MATERIALS & CONTINUA(2022)
摘要
Cognitive Radio (CR) has been developed as an enabling technology that allows the unused or underused spectrum to be used dynamically to increase spectral efficiency. To improve the overall performance of the CR system it is extremely important to adapt or reconfigure the system parameters. The Decision Engine is a major module in the CR-based system that not only includes radio monitoring and cognition functions but also responsible for parameter adaptation. As meta-heuristic algorithms offer numerous advantages compared to traditional mathematical approaches, the performance of these algorithms is investigated in order to design an efficient CR system that is able to adapt the transmitting parameters to effectively reduce power consumption, bit error rate and adjacent interference of the channel, while maximized secondary user throughput. Self-Learning Salp Swarm Algorithm (SLSSA) is a recent meta-heuristic algorithm that is the enhanced version of SSA inspired by the swarming behavior of salps. In this work, the parametric adaption of CR system is performed by SLSSA and the simulation results show that SLSSA has high accuracy, stability and outperforms other competitive algorithms for maximizing the throughput of secondary users. The results obtained with SLSSA are also shown to be extremely satisfactory and need fewer iterations to converge compared to the competitive methods.
更多查看译文
关键词
Cognitive radio, meta-heuristic algorithm, cognitive decision engine, salp swarm algorithm
AI 理解论文
溯源树
样例
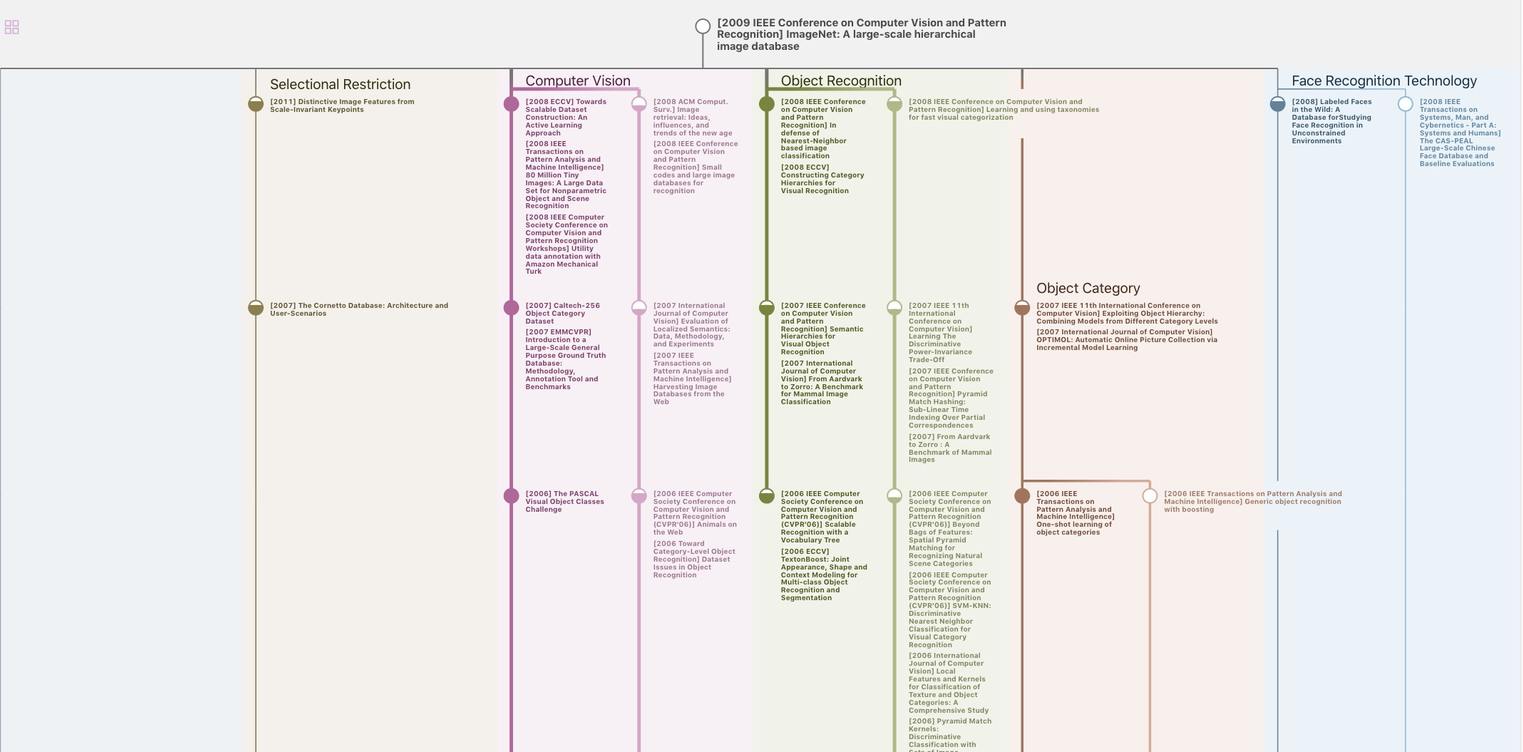
生成溯源树,研究论文发展脉络
Chat Paper
正在生成论文摘要