Scrapnet: An Efficient Approach To Trash Classification
IEEE ACCESS(2021)
摘要
As people have become more aware of their actions and how they affect their surroundings, they have realized the dire state of the environment. As a result, the recycling movement has gained momentum as a measure to save it. Contemporarily, the recycling industry has not seen a major shift and the problems that existed decades earlier persist. Trash classification is at the core of these problems, because if you can't classify it, obviously you can't recycle it. Manual classification often leads to misclassification as humans classify or judge things based on their experiences, knowledge, and not just absolute facts. Additionally, if the waste to be sorted is toxic, being in direct contact may be physically harmful to the people involved. Until a solution is found for this problem, the recycling industry won't be on par with the rise in recycling culture. Thus, this is the problem we have set out to solve; this paper proposes a Deep Learning model based on EfficientNet Architecture that can classify different kinds of trash with an accuracy high enough to make it a viable solution for the industry while using a comparatively lower number of parameters than existing methods. We achieved an accuracy of 98% on the TrashNet dataset, the standard data for Trash classification, and outperformed all existing models. Additionally, as no large dataset with a varied set of trash images was present, we created a new dataset of 8135 images by combining various datasets and standardized them, achieving a classification accuracy of 92.87% with EfficientNet B3.
更多查看译文
关键词
Recycling, Deep learning, Plastics, Industries, Waste management, Glass, Feature extraction, EfficientNet, recycle, ScrapNet, trash, trash classification
AI 理解论文
溯源树
样例
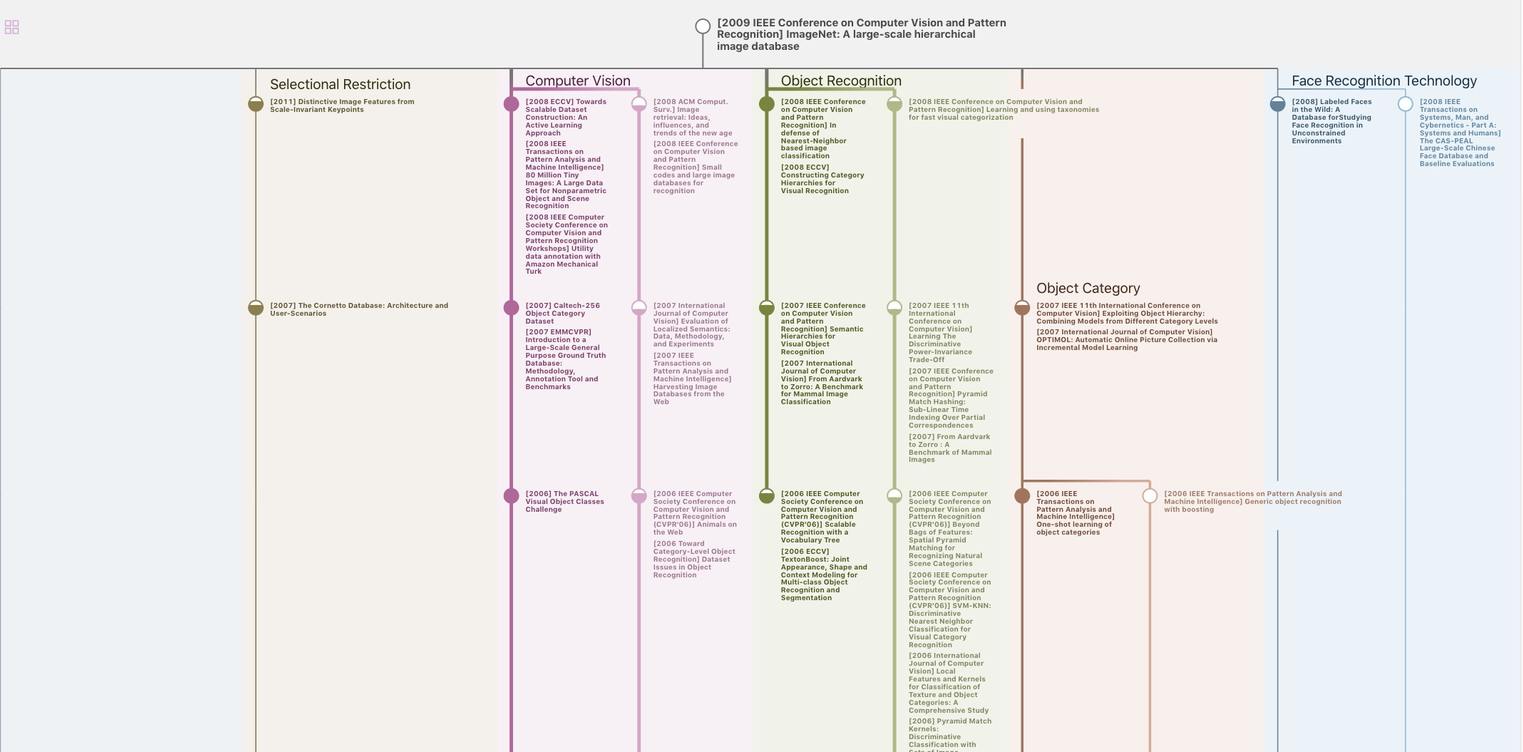
生成溯源树,研究论文发展脉络
Chat Paper
正在生成论文摘要