Data‐Driven Forecasting of Low‐Latitude Ionospheric Total Electron Content Using the Random Forest and LSTM Machine Learning Methods
Space weather(2021)
摘要
In this research, we present data-driven forecasting of ionospheric total electron content (TEC) using the Long-Short Term Memory (LSTM) deep recurrent neural network method. The random forest machine learning method was used to perform a regression analysis and estimate the variable importance of the input parameters. The input data are obtained from satellite and ground based measurements characterizing the solar-terrestrial environment. We estimate the relative importance of 34 different parameters, including the solar flux, solar wind density, and speed the three components of interplanetary magnetic field, Lyman-alpha, the Kp, Dst, and Polar Cap (PC) indices. The TEC measurements are taken with 15-s cadence from an equatorial GPS station located at Bogota, Columbia (4.7110 degrees N, 74.0721 degrees W). The 2008-2017 data set, including the top five parameters estimated using the random forest, is used for training the machine learning models, and the 2018 data set is used for independent testing of the LSTM forecasting. The LSTM method as applied to forecast the TEC up to 5 h ahead, with 30-min cadence. The results indicate that very good forecasts with low root mean square (RMS) error (high correlation) can be made in the near future and the RMS errors increase as we forecast further into the future. The data sources are satellite and ground based measurements characterizing the solar-terrestrial environment.
更多查看译文
关键词
LSTM,TEC forecasting,random forest,space weather,ionosphere
AI 理解论文
溯源树
样例
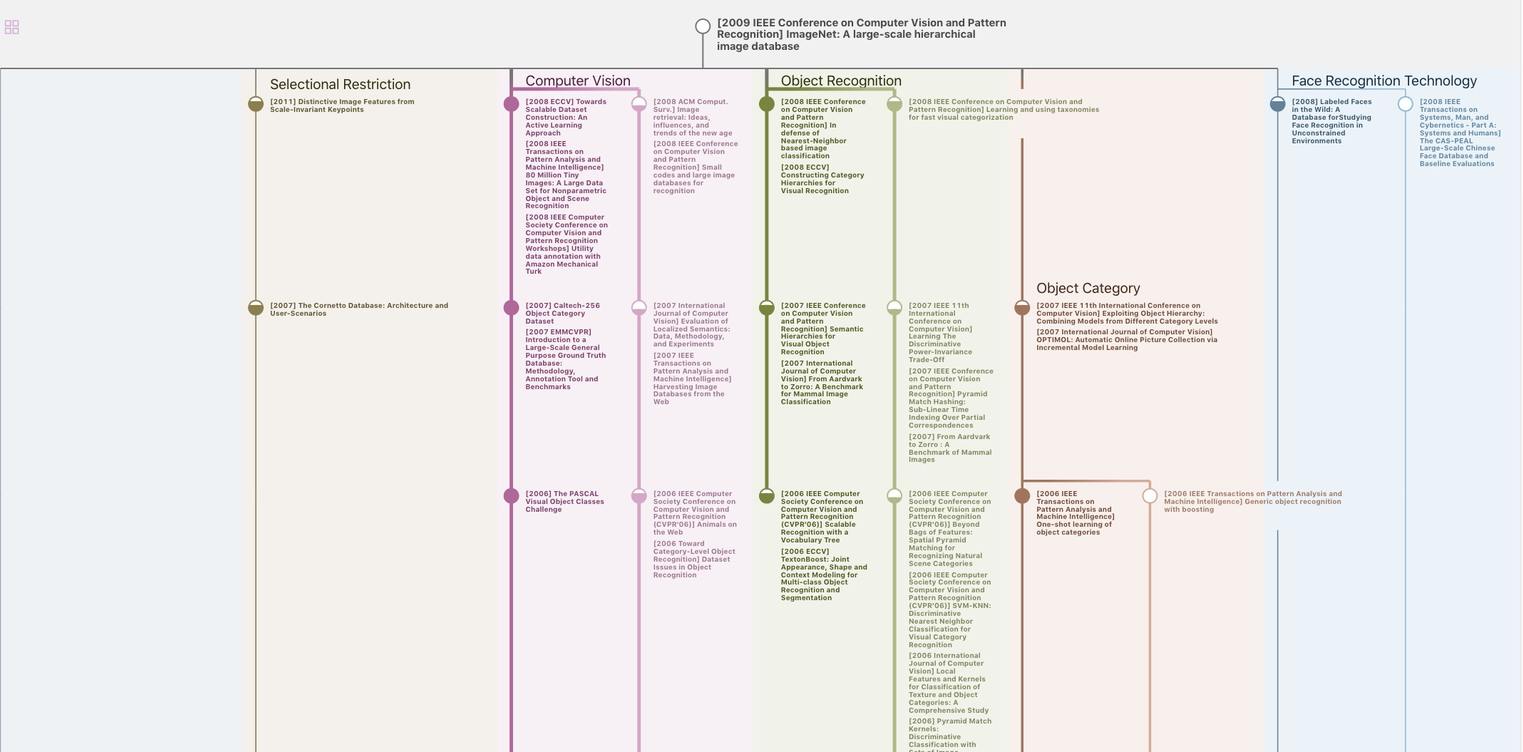
生成溯源树,研究论文发展脉络
Chat Paper
正在生成论文摘要