Prediction of marine heat flow based on the random forest method and geological and geophysical features
MARINE GEOPHYSICAL RESEARCH(2021)
摘要
Geothermal heat flow, as a parameter characterizing the Earth's thermal state, records deep thermodynamic processes. However, measurements of heat flow (HF) in the oceanic crust are relatively sparse and susceptible to surface activity such as hydrothermal circulation. We propose a machine learning approach to predict marine HF (MHF). We apply the random forest (RF) regression algorithm to train predictors capable of mapping multiple geological and geophysical features to MHF, thus enabling HF prediction in the global oceanic crust. We generate three data sets with different qualities of HF measurements for training. The best predictor has an accuracy of ~ 0.13 (normalized root mean squared error) or ~ 0.07 (normalized mean absolute error), and the predicted global oceanic crust HF map reflects the basic pattern of the MHF distribution. We find by comparison that the quality of HF measurement affects the prediction results. Then, we use a cross-prediction scheme to screen out the "underestimated" measured HF cases, which are mostly located in tectonic environments such as mid-ocean ridges and back-arc basins and show high spatial correlation with hydrothermal circulation. Furthermore, we conduct experimental calculations for the extent and proportion of underestimated cases in the oceanic part of a new global heat flow (NGHF) database; for example, the proportion of records with the degree of underestimation greater than 50% is approximately 30.8%. These calculations can provide reference information for the selection and application of MHF records in the NGHF database.
更多查看译文
关键词
Marine heat flow, Machine learning, Hydrothermal circulation, Random forest method
AI 理解论文
溯源树
样例
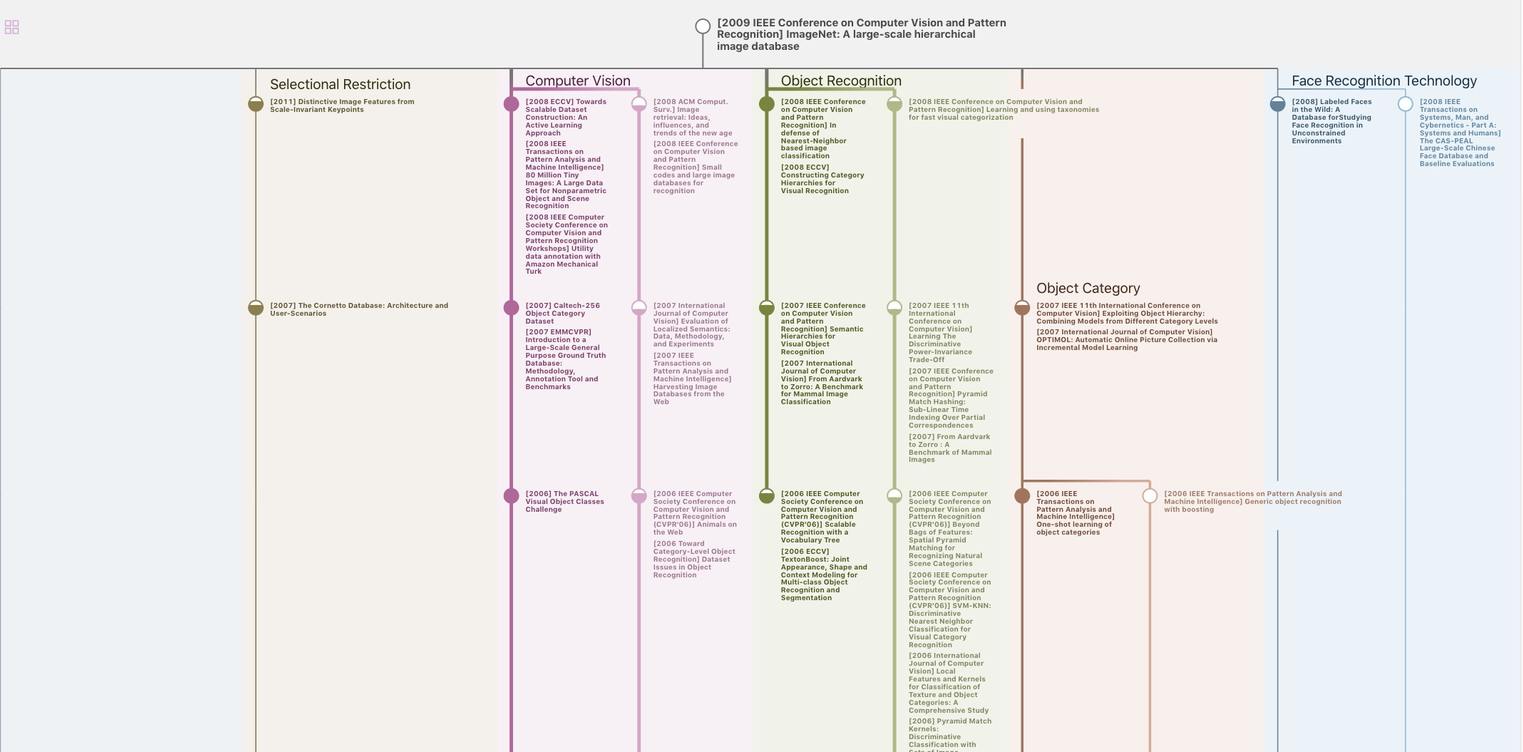
生成溯源树,研究论文发展脉络
Chat Paper
正在生成论文摘要