Real-Time Implementation of the Neutron/Gamma Discrimination in an FPGA-Based DAQ MTCA Platform Using a Convolutional Neural Network
IEEE Transactions on Nuclear Science(2021)
摘要
These days, research on the classification of neutron/gamma waveforms in scintillators using pulse shape discrimination (PSD) techniques is a highly studied topic. Numerous methods have been explored to optimize this classification, with some of the most recent research being focused on machine learning techniques with excellent results. These approaches are mainly based on the use of 1-D convolutional neural networks (CNNs). In this field, field-programmable gate arrays (FPGAs) with high-sampling rate analog to digital converters (ADCs) have been used to perform this classification in real-time. In this work, we select a potential architecture and implement it with the help of the IntelFPGA OpenCL SDK environment. A shorter and C-like development of OpenCL enables a more straightforward modification and optimization of the network architecture. The main goal of this work is the evaluation of the needed resources and the obtained performance to prototype a complete solution in the FPGA. The FPGA design is generated as if it was connected to an ADC module streaming the data samples with the help of a Board Support Package developed for an IntelFPGA ARRIA10 available in an Advanced Mezzanine Card (AMC) module in an Micro Telecommunications Computing Architecture (MTCA.4) platform. The prototyped solution has been integrated into Experimental Physics and Industrial Control System (EPICS) using the nominal device support (NDS) model currently being developed by ITER.
更多查看译文
关键词
Convolutional neural network (CNN),deep learning (DL),field-programmable gate array (FPGA),neutron-gamma (NG) discrimination,OpenCL hardware description
AI 理解论文
溯源树
样例
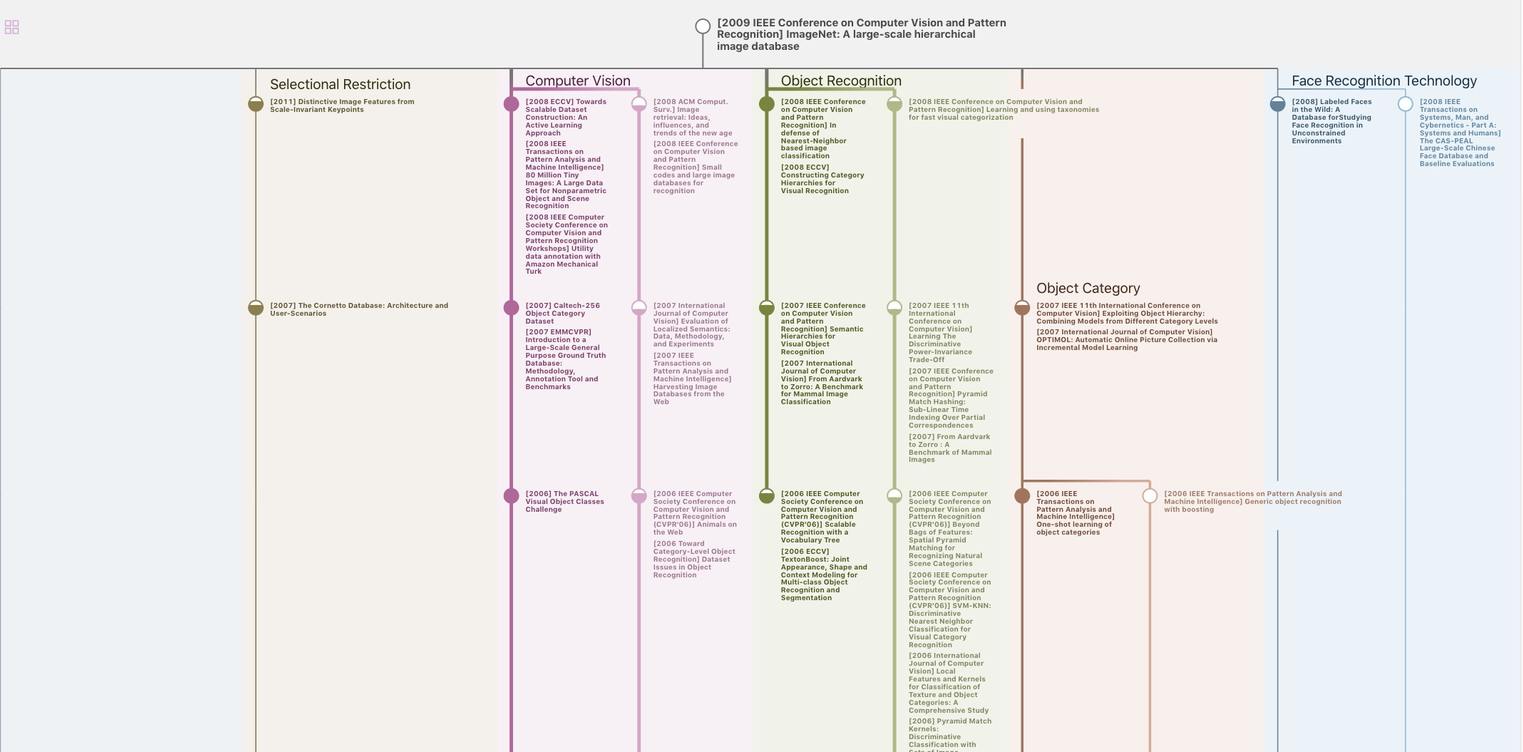
生成溯源树,研究论文发展脉络
Chat Paper
正在生成论文摘要