Robustness enhancement of machine fault diagnostic models for railway applications through data augmentation
Mechanical Systems and Signal Processing(2022)
摘要
The performance of machine learning based machine fault diagnosis (MFD) models could be impaired due to operating condition variations encountered in the real-world industrial environment, such as variations of operating speeds and loads. One major reason for this robustness problem is a lack of adequate training data, especially faulty data, measured in various operating conditions. To cover this gap, we propose a novel data augmentation framework for robustness enhancement in railway MFD applications. First, multibody dynamic simulation (MBS) for physical modeling is applied to simulate arbitrary faulty and operating conditions. Second, fast weighted feature-space averaging (FWFSA) as a new data augmentation technique is developed to augment the simulated faulty data, producing infinite reality-augmented simulation data. The proposed MBS-FWFSA can fit in arbitrary MFD algorithms and transfer-learning settings with minimal effort. Moreover, an in-depth empirical study has been carried out to investigate the causality between condition variations and robustness. A new metric has been defined to evaluate robustness. The experiments also revealed the effect of the proposed MBS-FWFSA and its outperformance against several state-of-the-art augmentation methods. The code and data used in this paper have been shared in our GitHub repository: https://github.com/quickhdsdc/Robustness-Enhancement-of-Machine-Fault-Diagnostic-Models.
更多查看译文
关键词
Data augmentation,Machine fault diagnosis,Machine learning,Robustness,Covariate shift
AI 理解论文
溯源树
样例
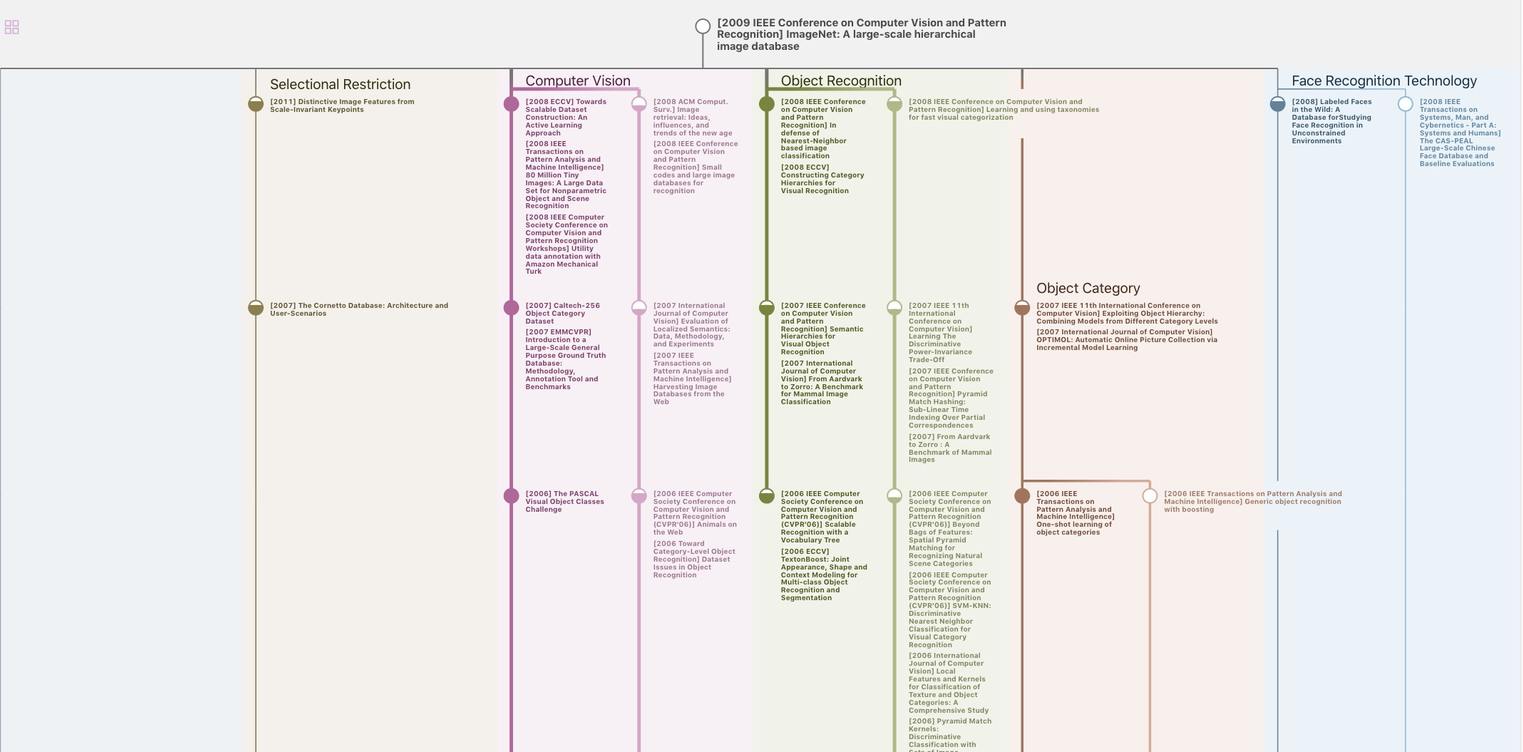
生成溯源树,研究论文发展脉络
Chat Paper
正在生成论文摘要