Deep and interpretable regression models for ordinal outcomes
Pattern Recognition(2022)
摘要
Outcomes with a natural order commonly occur in prediction problems and often the available input data are a mixture of complex data like images and tabular predictors. Deep Learning (DL) models are state-of-the-art for image classification tasks but frequently treat ordinal outcomes as unordered and lack interpretability. In contrast, classical ordinal regression models consider the outcome's order and yield interpretable predictor effects but are limited to tabular data. We present ordinal neural network transformation models (omkams), which unite DL with classical ordinal regression approaches. ONTRAM5 are a special case of transformation models and trade off flexibility and interpretability by additively decomposing the transformation function into terms for image and tabular data using jointly trained neural networks. The performance of the most flexible ONTRAM is by definition equivalent to a standard multiclass DL model trained with cross-entropy while being faster in training when facing ordinal outcomes. Lastly, we discuss how to interpret model components for both tabular and image data on two publicly available datasets. (C) 2021 Published by Elsevier Ltd.
更多查看译文
关键词
Deep learning,Interpretability,Distributional regression,Ordinal regression,Transformation models
AI 理解论文
溯源树
样例
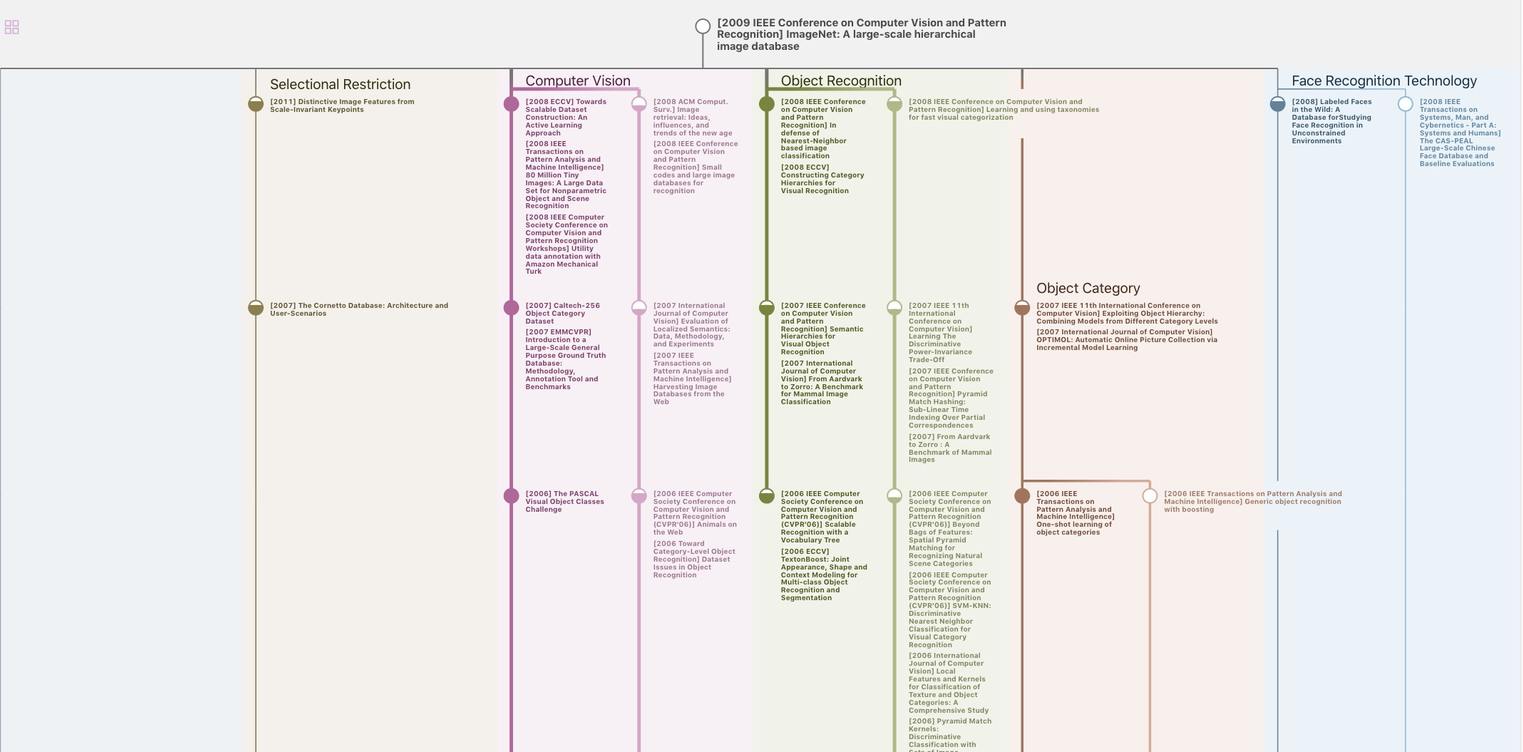
生成溯源树,研究论文发展脉络
Chat Paper
正在生成论文摘要