Arf-Net: An Adaptive Receptive Field Network For Breast Mass Segmentation In Whole Mammograms And Ultrasound Images
BIOMEDICAL SIGNAL PROCESSING AND CONTROL(2022)
摘要
UNet adopting an encoder-decoder structure has been used widely in medical image segmentation tasks for its outstanding performance. However, in our work, we find that UNet has the worse segmentation performance of small masses. The reason behind this is that the sizes of receptive fields are limited. In this work, to address this issue, we develop a novel end-to-end model, Adaptive Receptive Field Network (ARF-Net), for the precise breast mass segmentation in whole mammographic images and ultrasound images. ARF-Net composes of an encoder network and a corresponding decoder network, followed by a pixel-wise classifier. In ARF-Net, a Selective Receptive Filed Module (SRFM) is proposed to allocate the suitable sizes of receptive fields to the breast masses of different sizes. SRFM consists of a Multiple Receptive Field Module (MRFM) for generating multiple receptive fields of different sizes and a Multi-Scale Selection Module (MSSM) for selecting the suitable sizes of receptive fields based on the objects' size. The proposed ARF-Net achieves the dice index of 86.1%, 85.75%, and 88.12% on the two mammographic databases (INbreast and CBIS-DDSM) and one ultrasonic database (UDIAT), respectively. Moreover, extensive ablation experiments show that ARF-Net transcends several state-of-the-art segmentation networks, and the developed MSSM exceeds several counterparts.
更多查看译文
关键词
Segmentation, Mammogram, Ultrasound image, Deep learning, Encoder-decoder
AI 理解论文
溯源树
样例
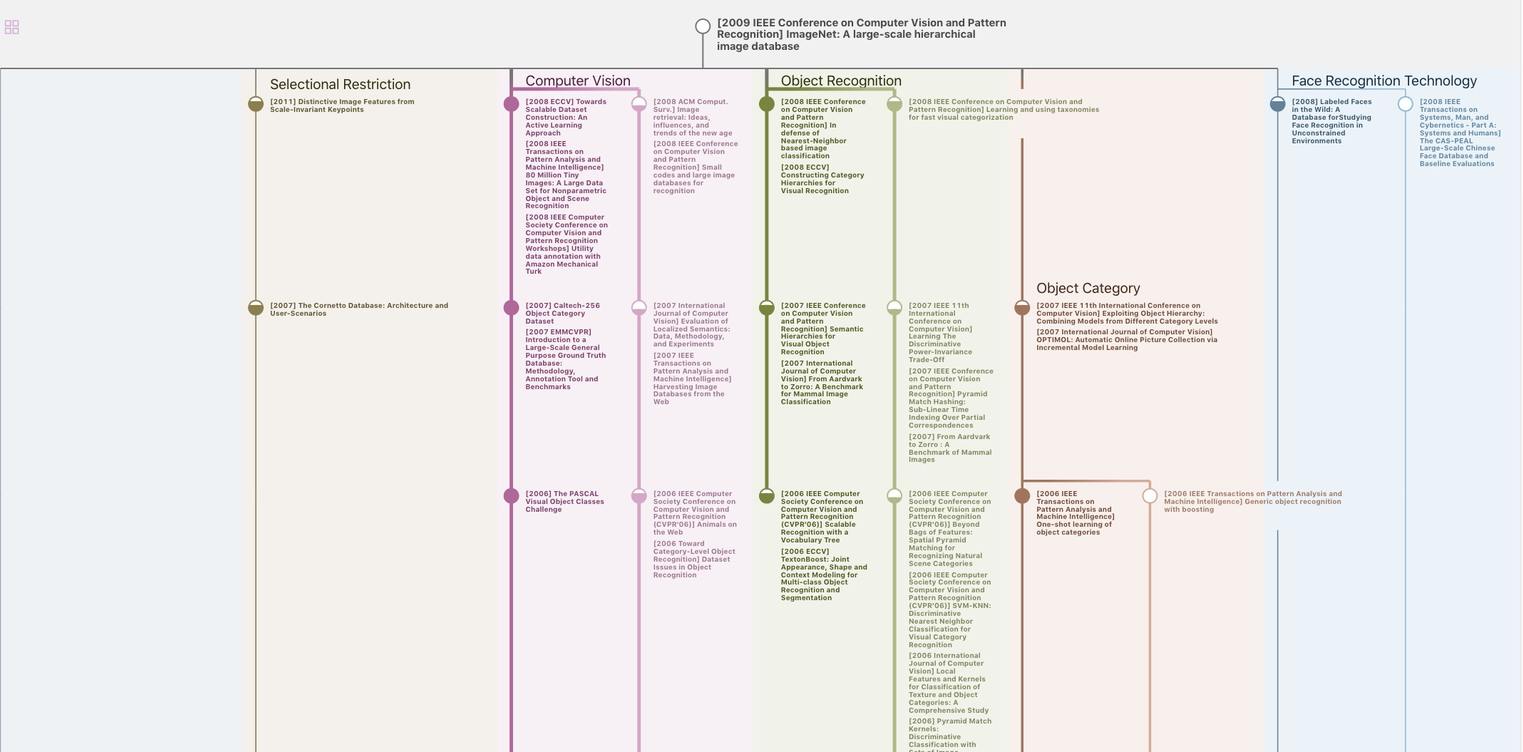
生成溯源树,研究论文发展脉络
Chat Paper
正在生成论文摘要