Development of a machine learning-based predictor for identifying and discovering antioxidant peptides based on a new strategy
Food Control(2022)
摘要
It is necessary to solve the problem of food corruption and oxidation to improve food quality. Peptides are a good candidate to solve the above problems. In this paper, a machine learning method was used to construct an antioxidant peptide classification model based on the pseudo-amino acid composition and motifs of peptides as input features. The AUC of PseAAC-dipeptide-motif hybrid model is 0.939 and the average precision score is 0.947, which is the best among all models in this paper. Besides, the classification threshold has been increased to make the model precision above 0.95. Then, the model was used as predictor to discover potential antioxidant peptides from a random peptide dataset. According to the predicted results, 5 potential antioxidant peptides (PSGK, LKPQ, GRP, QCQ, QGM) were synthesized to determine their DPPH radical-scavenging activity and the total antioxidant capacity (T-AOC). The experimental results show that QCQ has strong antioxidant properties, its T-AOC value is 9.59U/mg prot, and the DPPH scavenging activity is 95.52% at concentration of 125 μg/mL. Meantime, the predictor can be used to mine multifunctional peptides with antioxidant function. In general, the predictor is an effective tool for discovering peptides with antioxidant function.
更多查看译文
关键词
Machine learning,SVM,Hybrid model,Multifunctional peptide,Antioxidant peptide
AI 理解论文
溯源树
样例
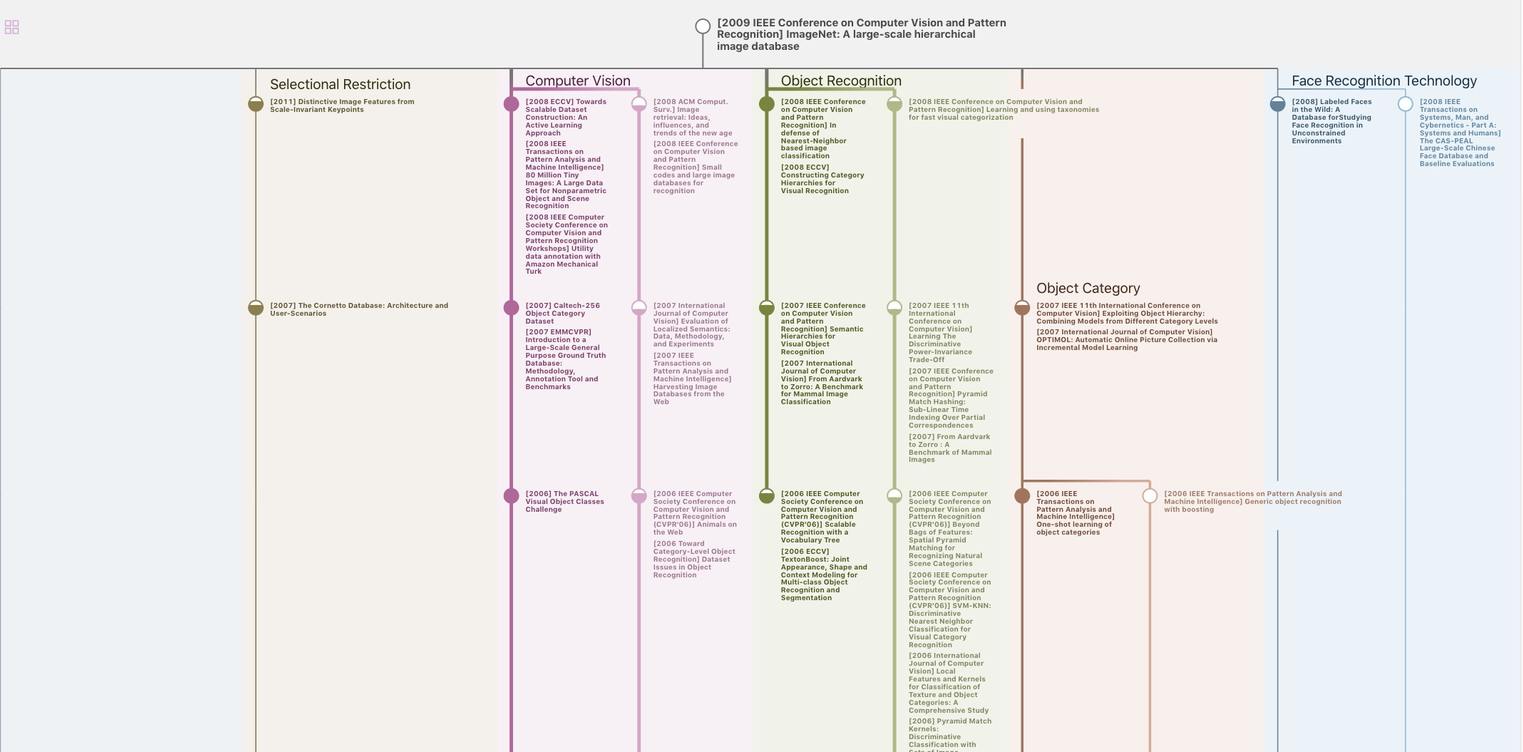
生成溯源树,研究论文发展脉络
Chat Paper
正在生成论文摘要